MSMD-Net: Deep Stereo Matching with Multi-scale and Multi-dimension Cost Volume.
CoRR(2020)
摘要
Deep end-to-end learning based stereo matching methods have achieved great success as witnessed by the leaderboards across different benchmarking datasets (KITTI, Middlebury, ETH3D, etc), where the cost volume representation is an indispensable step to the success. However, most existing work only employs a single cost volume, which cannot fully exploit the multi-scale cues in stereo matching and provide guidance for disparity refinement. What's more, the single cost volume representation also limits the disparity range and the resolution of the disparity estimation. In this paper, we propose MSMD-Net (Multi-Scale and Multi-Dimension) to construct multi-scale and multi-dimension cost volume. At the multi-scale level, we generate four 4D combination volumes at different scales and integrate them in 3D cost aggregation to predict an initial disparity estimation. At the multi-dimension level, we construct a 3D warped correlation volume and use it to refine the initial disparity map with residual learning. These two dimensional cost volumes are complementary to each other and can boost the performance of disparity estimation. Additionally, we propose a switch training strategy to further improve the accuracy of disparity estimation, where we switch two kinds of different activation functions to alleviate the overfitting issue in the pre-training process. Our proposed method was evaluated on several benchmark datasets and ranked first on KITTI 2012 leaderboard and second on KITTI 2015 leaderboard as of June 23.The code of MSMD-Net is available at https://github.com/gallenszl/MSMD-Net.
更多查看译文
关键词
Stereo matching, Pyramid cost volume, Cross-domain generalization
AI 理解论文
溯源树
样例
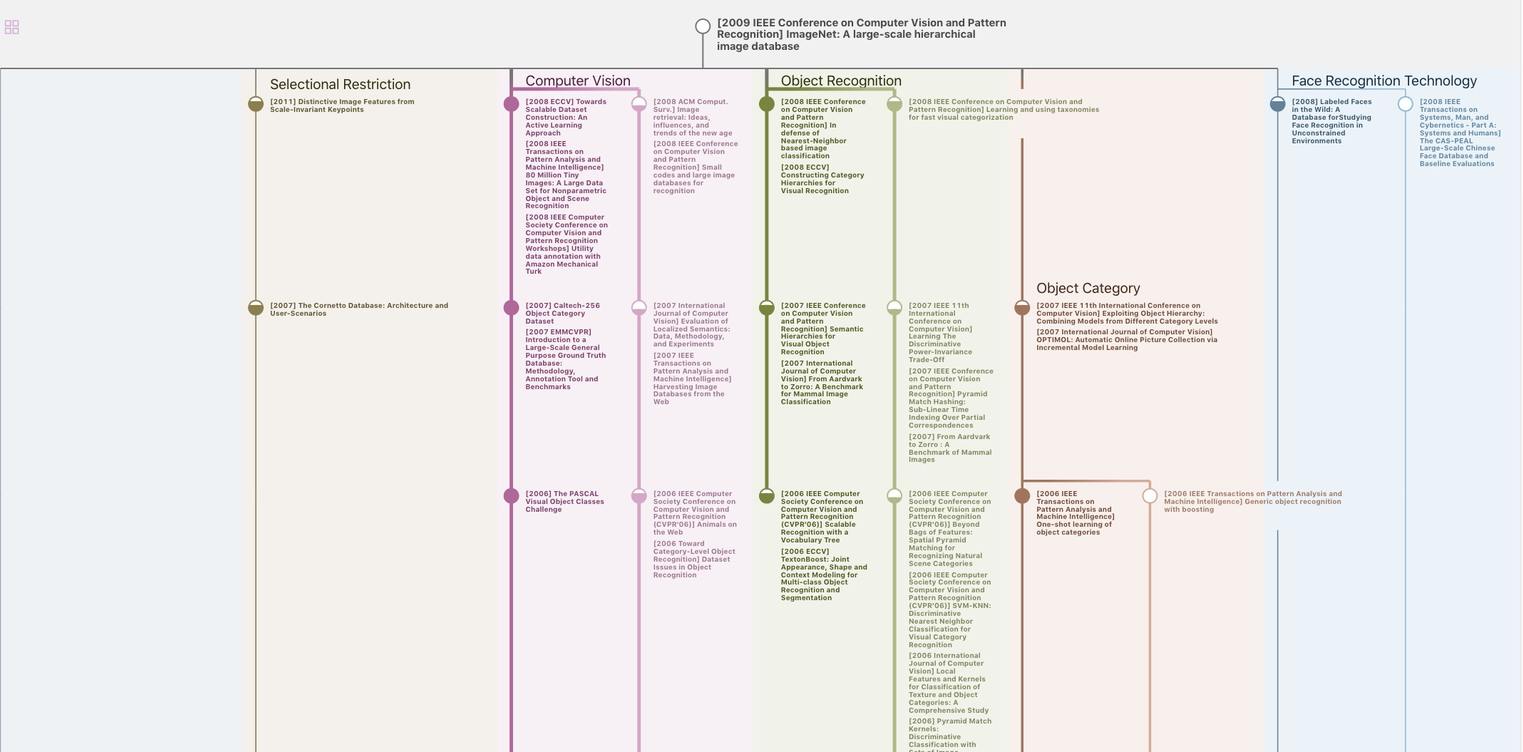
生成溯源树,研究论文发展脉络
Chat Paper
正在生成论文摘要