Identification of Neuronal Polarity by Node-Based Machine Learning
NEUROINFORMATICS(2021)
摘要
Identifying the direction of signal flows in neural networks is important for understanding the intricate information dynamics of a living brain. Using a dataset of 213 projection neurons distributed in more than 15 neuropils of a Drosophila brain, we develop a powerful machine learning algorithm: node-based polarity identifier of neurons (NPIN). The proposed model is trained only by information specific to nodes, the branch points on the skeleton, and includes both Soma Features (which contain spatial information from a given node to a soma) and Local Features (which contain morphological information of a given node). After including the spatial correlations between nodal polarities, our NPIN provided extremely high accuracy (>96.0%) for the classification of neuronal polarity, even for complex neurons with more than two dendrite/axon clusters. Finally, we further apply NPIN to classify the neuronal polarity of neurons in other species (Blowfly and Moth), which have much less neuronal data available. Our results demonstrate the potential of NPIN as a powerful tool to identify the neuronal polarity of insects and to map out the signal flows in the brain’s neural networks if more training data become available in the future.
更多查看译文
关键词
Neuronal polarity, Machine learning, Drosophila, Connectome, Axon, Dendrite
AI 理解论文
溯源树
样例
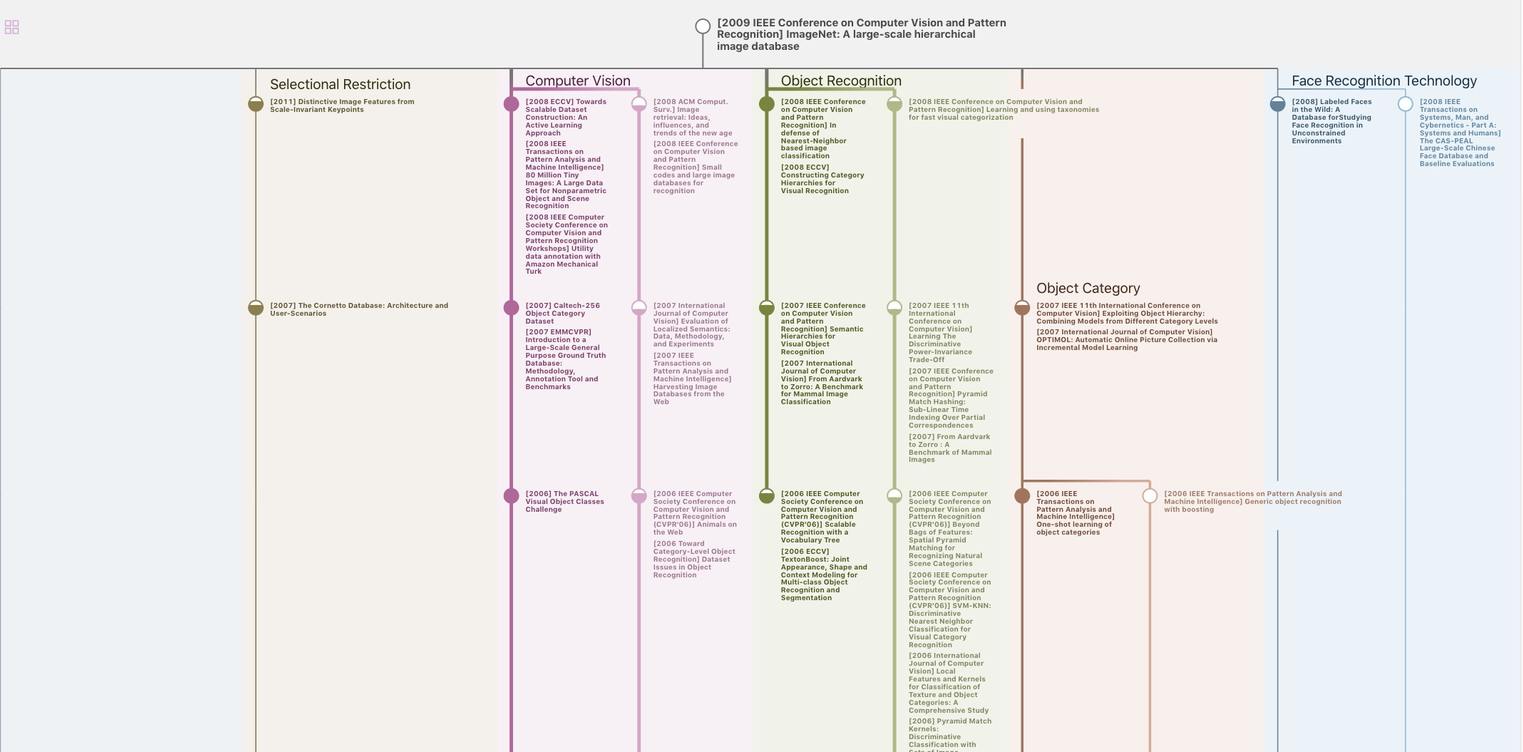
生成溯源树,研究论文发展脉络
Chat Paper
正在生成论文摘要