Evaluating Robustness of SHARP–An Adaptive Human Behavior Model for Repeated SSGs
user-5ebe28d54c775eda72abcdf7(2016)
摘要
Several competing human behavior models have been proposed to model and protect against boundedly rational adversaries in repeated Stackelberg security games (RSSGs). One such recent model, SHARP, addressed the limitations of earlier models and demonstrated its superiority in RSSGs against human subjects recruited from the Amazon Mechanical Turk platform in the first “longitudinal” study–at least in the context of SSGs. SHARP has three key novelties:(i) SHARP reasons based on success or failure of the adversary’s past actions on exposed portions of the attack surface to model adversary adaptiveness;(ii) SHARP reasons about similarity between exposed and unexposed areas of the attack surface, and also incorporates a discounting parameter to mitigate adversary’s lack of exposure to enough of the attack surface; and (iii) SHARP integrates a non-linear probability weighting function to capture the adversary’s true weighting of probability. However, despite its success, the effectiveness of SHARP’s modeling considerations and also the robustness of the experimental results has never been tested. Therefore, in this paper, we provide the following new contributions. First, we test our model SHARP in human subjects experiments at the Bukit Barisan Seletan National Park in Indonesia against wildlife security experts and provide results and analysis of the data. Second, we conduct new human subjects experiments on Amazon Mechanical Turk (AMT) to show the extent to which past successes and failures affect the adversary’s future decisions in RSSGS. Third, we conduct new analysis on our human subjects data and illustrate the …
更多查看译文
AI 理解论文
溯源树
样例
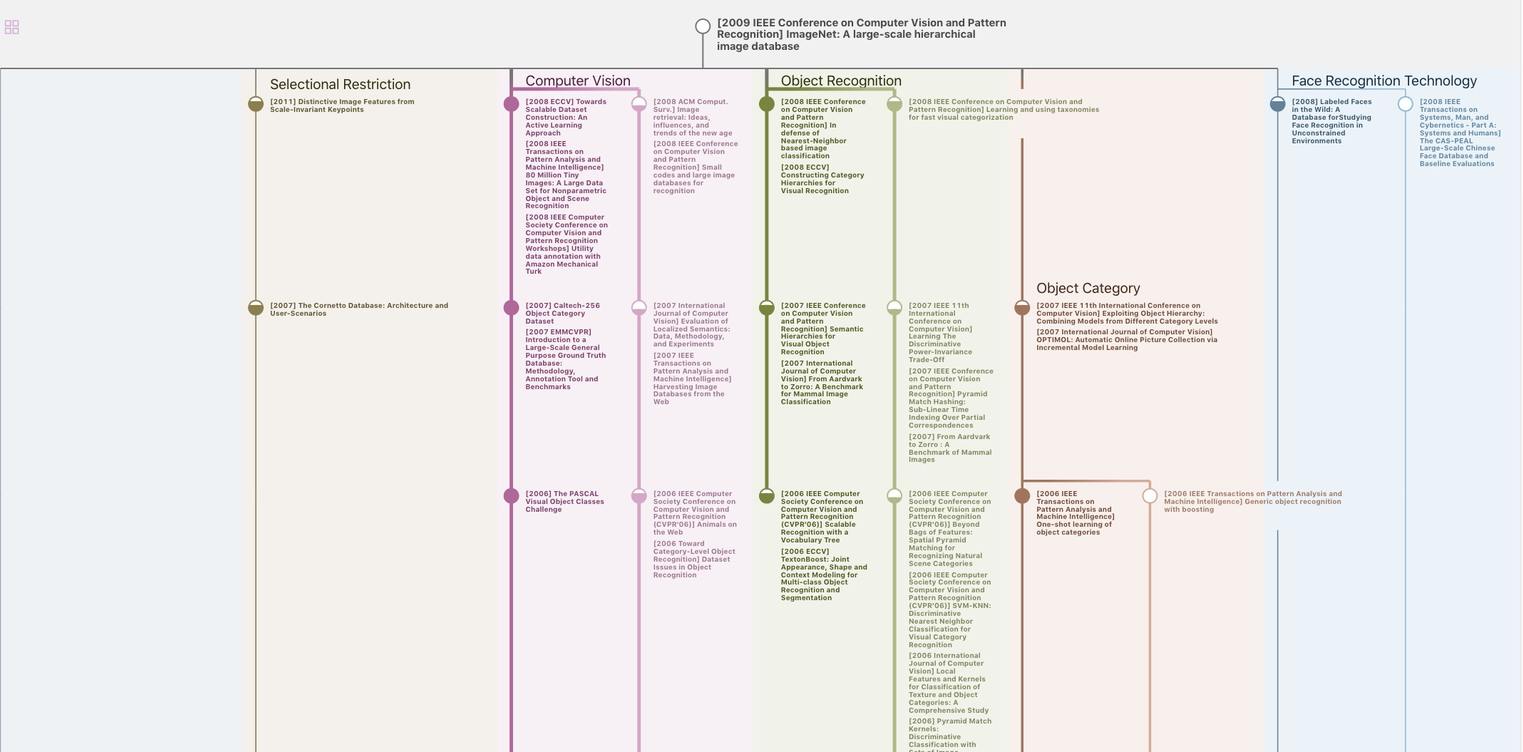
生成溯源树,研究论文发展脉络
Chat Paper
正在生成论文摘要