Set Distribution Networks: a Generative Model for Sets of Images
arxiv(2020)
摘要
Images with shared characteristics naturally form sets. For example, in a face verification benchmark, images of the same identity form sets. For generative models, the standard way of dealing with sets is to represent each as a one hot vector, and learn a conditional generative model $p(\mathbf{x}|\mathbf{y})$. This representation assumes that the number of sets is limited and known, such that the distribution over sets reduces to a simple multinomial distribution. In contrast, we study a more generic problem where the number of sets is large and unknown. We introduce Set Distribution Networks (SDNs), a novel framework that learns to autoencode and freely generate sets. We achieve this by jointly learning a set encoder, set discriminator, set generator, and set prior. We show that SDNs are able to reconstruct image sets that preserve salient attributes of the inputs in our benchmark datasets, and are also able to generate novel objects/identities. We examine the sets generated by SDN with a pre-trained 3D reconstruction network and a face verification network, respectively, as a novel way to evaluate the quality of generated sets of images.
更多查看译文
关键词
distribution networks,generative model,images,sets
AI 理解论文
溯源树
样例
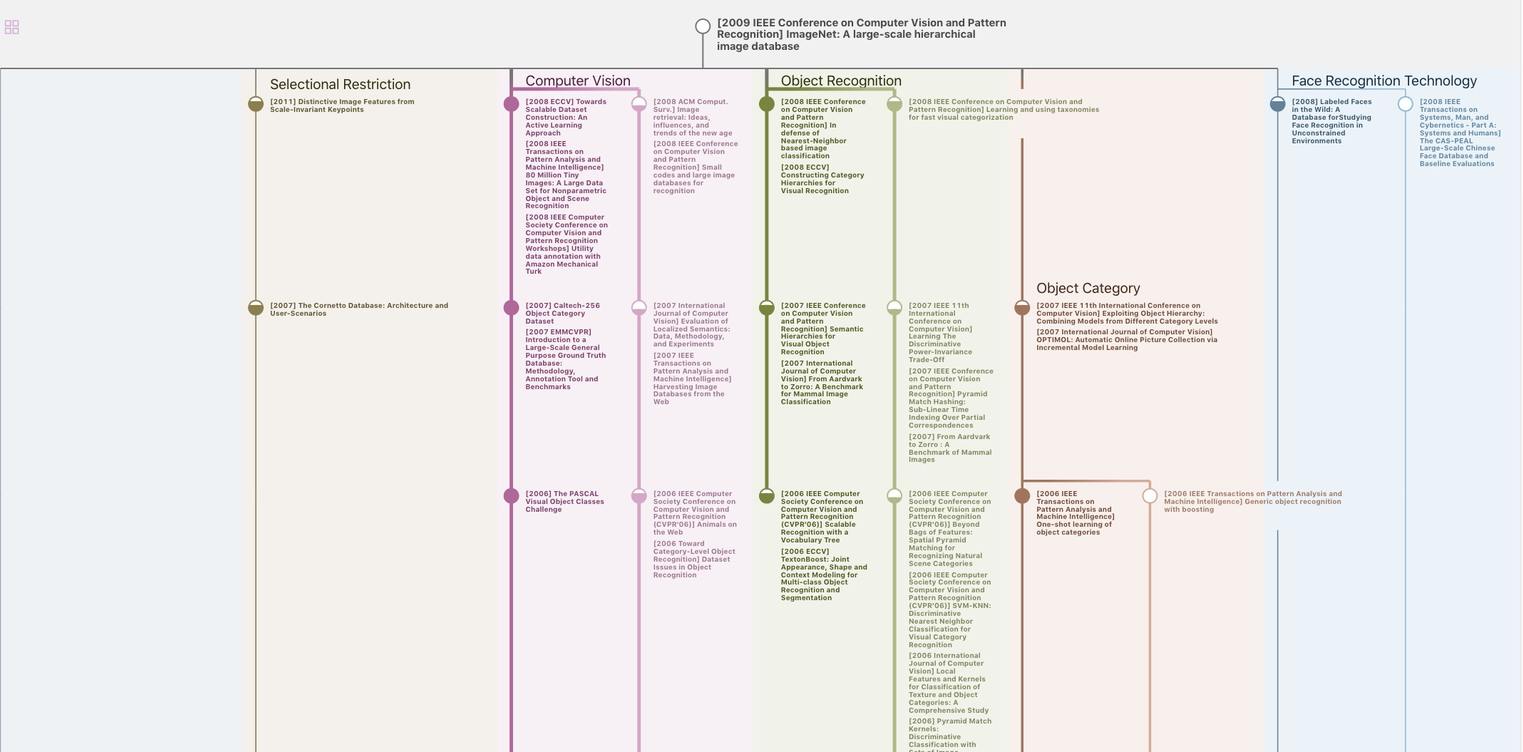
生成溯源树,研究论文发展脉络
Chat Paper
正在生成论文摘要