Self-Supervised Learning: Generative or Contrastive
IEEE TRANSACTIONS ON KNOWLEDGE AND DATA ENGINEERING(2023)
摘要
Deep supervised learning has achieved great success in the last decade. However, its defects of heavy dependence on manual labels and vulnerability to attacks have driven people to find other paradigms. As an alternative, self-supervised learning (SSL) attracts many researchers for its soaring performance on representation learning in the last several years. Self-supervised representation learning leverages input data itself as supervision and benefits almost all types of downstream tasks. In this survey, we take a look into new self-supervised learning methods for representation in computer vision, natural language processing, and graph learning. We comprehensively review the existing empirical methods and summarize them into three main categories according to their objectives: generative, contrastive, and generative-contrastive (adversarial). We further collect related theoretical analysis on self-supervised learning to provide deeper thoughts on why self-supervised learning works. Finally, we briefly discuss open problems and future directions for self-supervised learning. An outline slide for the survey is provided(1).
更多查看译文
关键词
Self-supervised learning,generative model,contrastive learning,deep learning
AI 理解论文
溯源树
样例
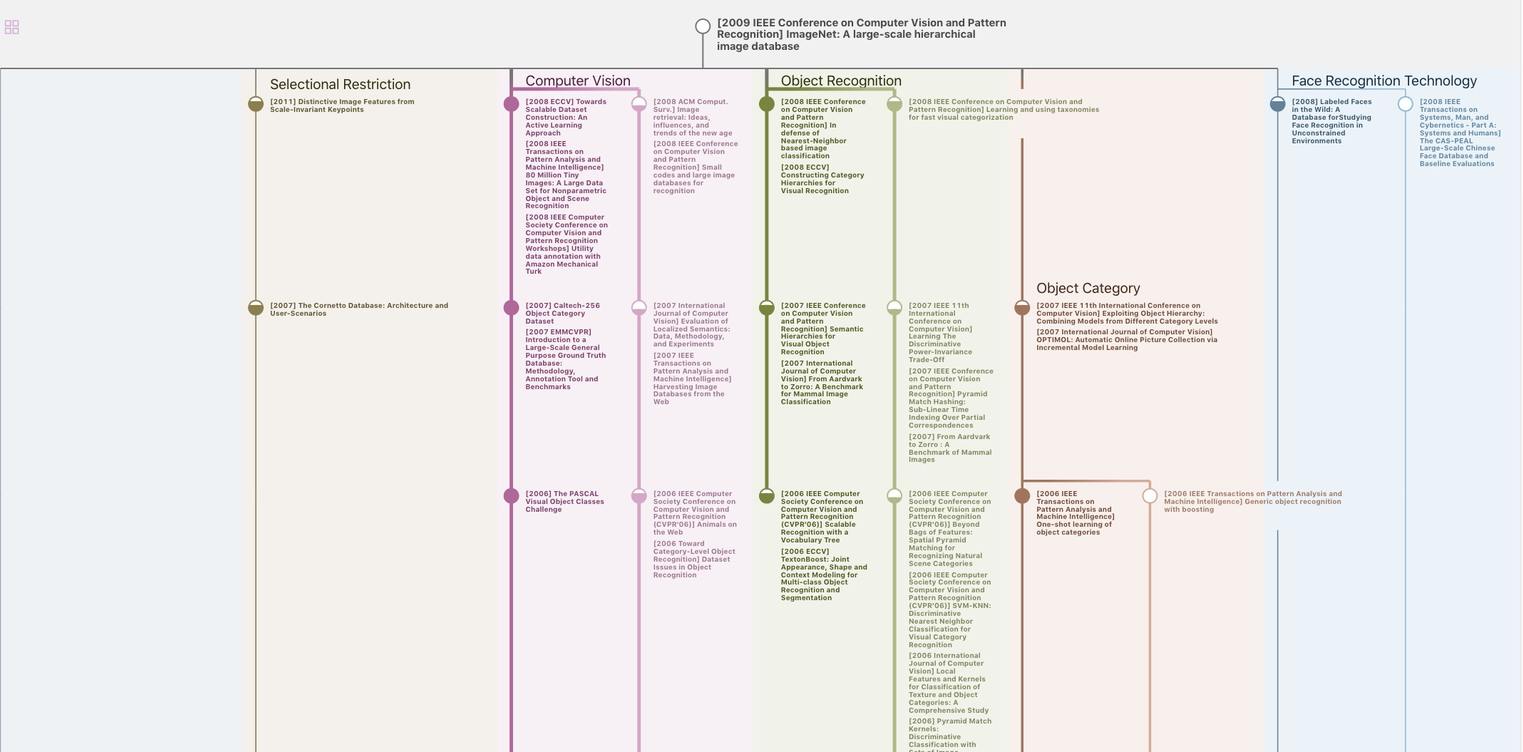
生成溯源树,研究论文发展脉络
Chat Paper
正在生成论文摘要