Model Compression Using Progressive Channel Pruning
IEEE Transactions on Circuits and Systems for Video Technology(2021)
摘要
In this work, we propose a simple but effective channel pruning framework called Progressive Channel Pruning (PCP) to accelerate Convolutional Neural Networks (CNNs). In contrast to the existing channel pruning methods that prune the channels only once per layer in a layer-by-layer fashion, our new progressive framework iteratively prunes a small number of channels from several selected layers, which consists of a three-step attempting-selecting-pruning pipeline in each iteration. In the attempting step, we attempt to prune a pre-defined number of channels from one layer by using any existing channel pruning methods and estimate the accuracy drop for this layer based on the labelled samples in the validation set. In the selecting step, based on the estimated accuracy drops for all layers, we propose a greedy strategy to automatically select a set of layers that will lead to less overall accuracy drop after pruning these layers. In the pruning step, we prune a small number of channels from these selected layers. We further extend our PCP framework to prune channels for the deep transfer learning methods like Domain Adversarial Neural Network (DANN), in which we effectively reduce the data distribution mismatch in the channel pruning process by using both labelled samples from the source domain and pseudo-labelled samples from the target domain. Our comprehensive experiments on two benchmark datasets demonstrate that our PCP framework outperforms the existing channel pruning approaches under both supervised learning and transfer learning settings.
更多查看译文
关键词
Model compression,channel pruning,domain adaptation,transfer learning
AI 理解论文
溯源树
样例
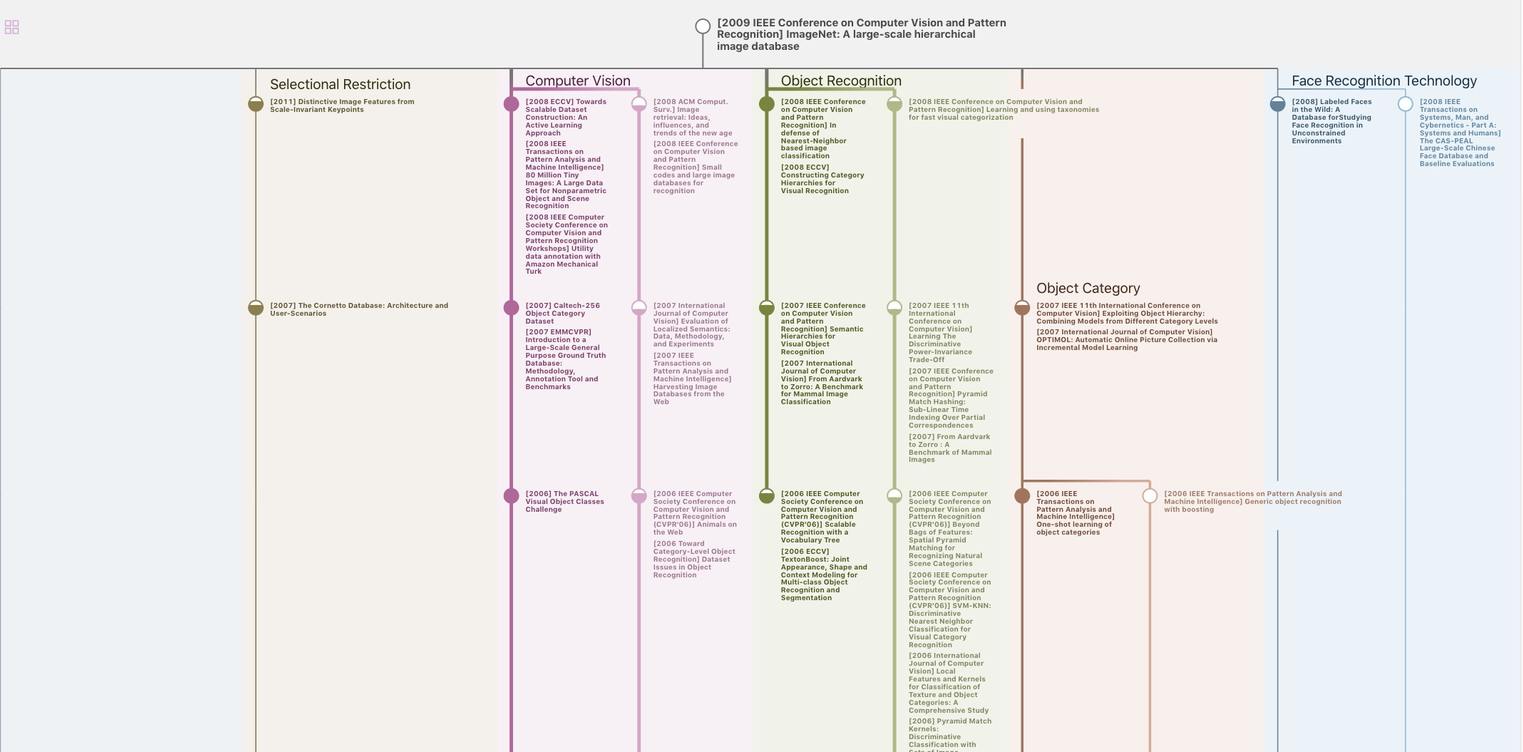
生成溯源树,研究论文发展脉络
Chat Paper
正在生成论文摘要