Robust Subspace Clustering With Low-Rank Structure Constraint
IEEE Transactions on Knowledge and Data Engineering(2022)
摘要
In this paper, a novel low-rank structural model is proposed for segmenting data drawn from a high-dimensional space. Our method is based on the fact that all groups clustered from a high-dimensional dataset are distributed in multiple low-rank subspaces. In general, it’s a very difficult task to find the low-rank structures hidden in data. Different from the classical sparse subspace clustering (SSC) and low-rank representation (LRR) which all take two steps including building the affinity matrix and spectral clustering, we introduce a new rank constraint into our model. This constraint allows our model to learn a subspace indicator which can capture different clusters directly from the data without any postprocessing. To further approximate the rank constraint, a piecewise function is utilized as the relaxing item for the proposed model. Besides, under the subspace indicator constraints, the integer programming problem is avoided, which makes our algorithm more efficient and scalable. In addition, we prove the convergence of the proposed algorithm in theory and further discuss the general case in which subspaces don’t pass through the origin. Experiment results on both synthetic and real-world datasets demonstrate that our algorithm significantly outperforms the state-of-the-art methods.
更多查看译文
关键词
Subspace clustering,low-rank structure,singular value decomposition (SVD),piecewise function
AI 理解论文
溯源树
样例
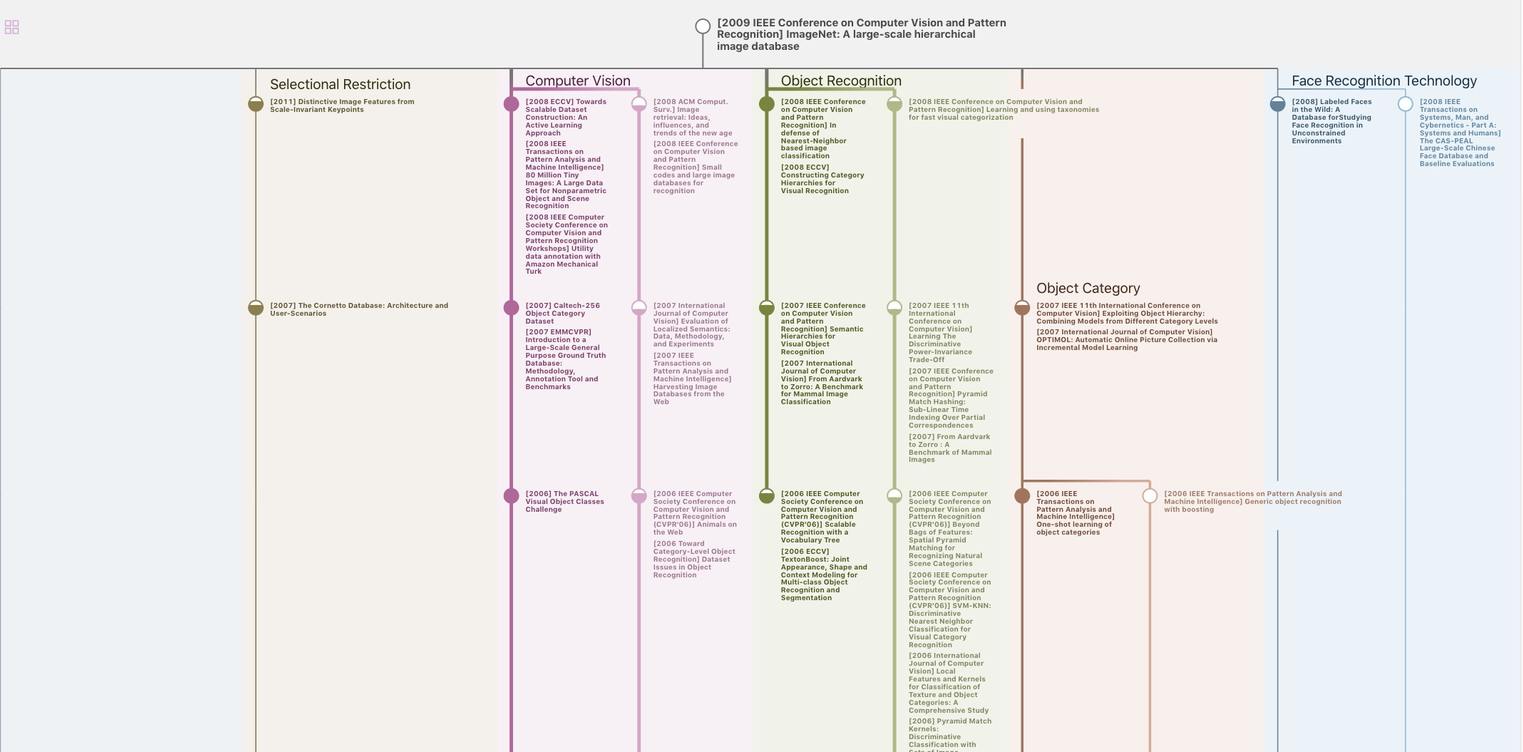
生成溯源树,研究论文发展脉络
Chat Paper
正在生成论文摘要