PERCEIVE: deep learning-based cellular uplink prediction using real-time scheduling patterns
MobiSys '20: The 18th Annual International Conference on Mobile Systems, Applications, and Services Toronto Ontario Canada June, 2020(2020)
摘要
As video calls and personal broadcasting become popular, the demand for mobile live streaming over cellular uplink channels is growing fast. However, current live streaming solutions are known to suffer from frequent uplink throughput fluctuations causing unnecessary video stalls and quality drops. As a remedy to this problem, we propose PERCEIVE, a deep learning-based uplink throughput prediction framework. PERCEIVE exploits a 2-stage LSTM (Long Short Term Memory) design and makes throughput predictions for the next 100ms. Our extensive evaluations show that PERCEIVE, trained with LTE network traces from three major operators in the U.S., achieves high accuracy in the uplink throughput prediction with only 7.67% mean absolute error and outperforms existing prediction techniques. We integrate PERCEIVE with WebRTC, a popular video streaming platform from Google, as a rate adaptation module. Our implementation on the Android phone demonstrates that it can improve PSNR by up to 6dB (4x) over the default WebRTC while providing less streaming latency.
更多查看译文
AI 理解论文
溯源树
样例
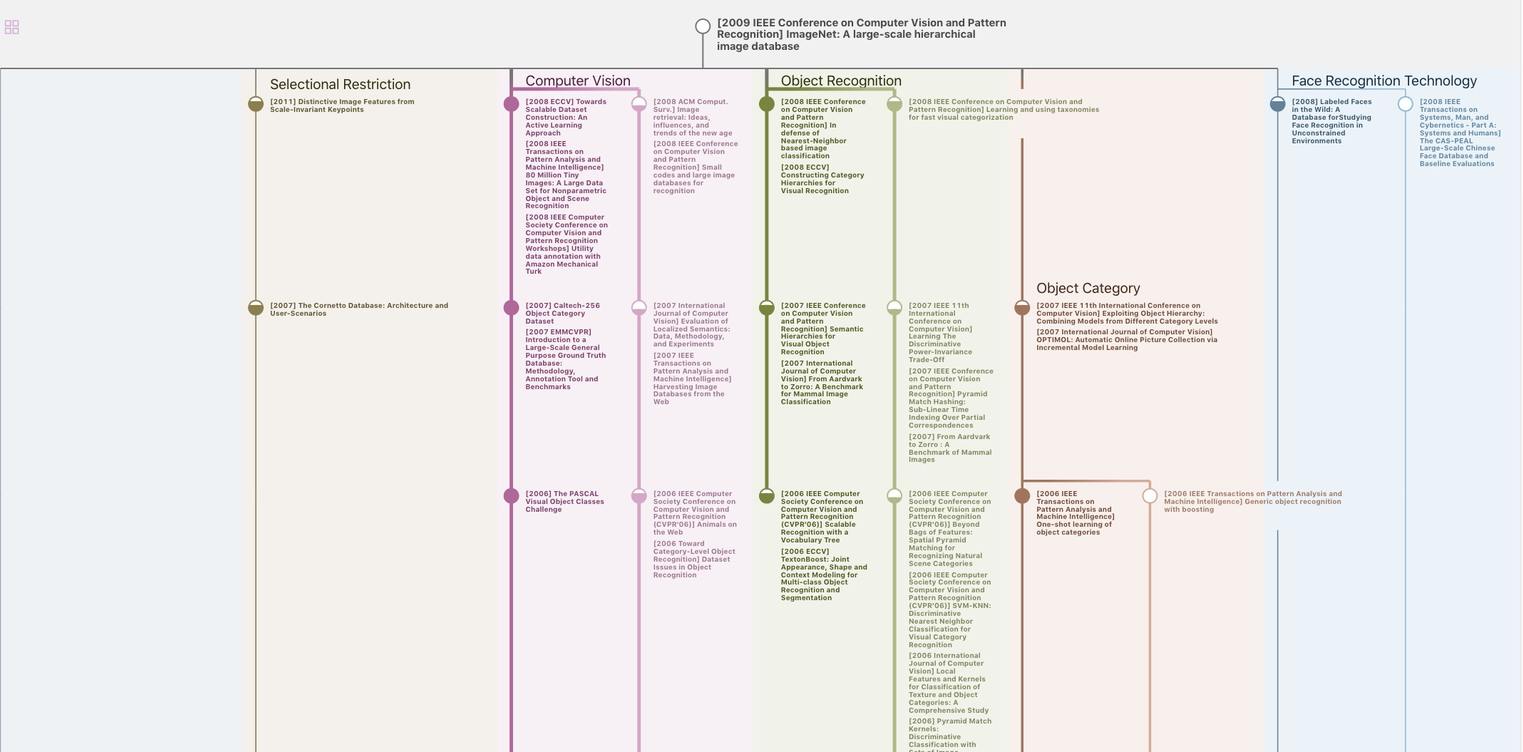
生成溯源树,研究论文发展脉络
Chat Paper
正在生成论文摘要