Spherical Criteria For Fast And Accurate 360 Degrees Object Detection
AAAI(2020)
摘要
With the advance of omnidirectional panoramic technology, 360 degrees imagery has become increasingly popular in the past few years. To better understand the 360 degrees content, many works resort to the 360 degrees object detection and various criteria have been proposed to bound the objects and compute the intersection-over-union (IoU) between bounding boxes based on the common equirectangular projection (ERP) or perspective projection (PSP). However, the existing 360 degrees criteria are either inaccurate or inefficient for real-world scenarios. In this paper, we introduce a novel spherical criteria for fast and accurate 360 degrees object detection, including both spherical bounding boxes and spherical IoU (SphIoU). Based on the spherical criteria, we propose a novel two-stage 360 degrees detector, i.e., Reprojection R-CNN, by combining the advantages of both ERP and PSP, yielding efficient and accurate 360 degrees object detection. To validate the design of spherical criteria and Reprojection R-CNN, we construct two unbiased synthetic datasets for training and evaluation. Experimental results reveal that compared with the existing criteria, the two-stage detector with spherical criteria achieves the best mAP results under the same inference speed, demonstrating that the spherical criteria can be more suitable for 360 degrees object detection. Moreover, Reprojection R-CNN outperforms the previous state-of-the-art methods by over 30% on mAP with competitive speed, which confirms the efficiency and accuracy of the design.
更多查看译文
AI 理解论文
溯源树
样例
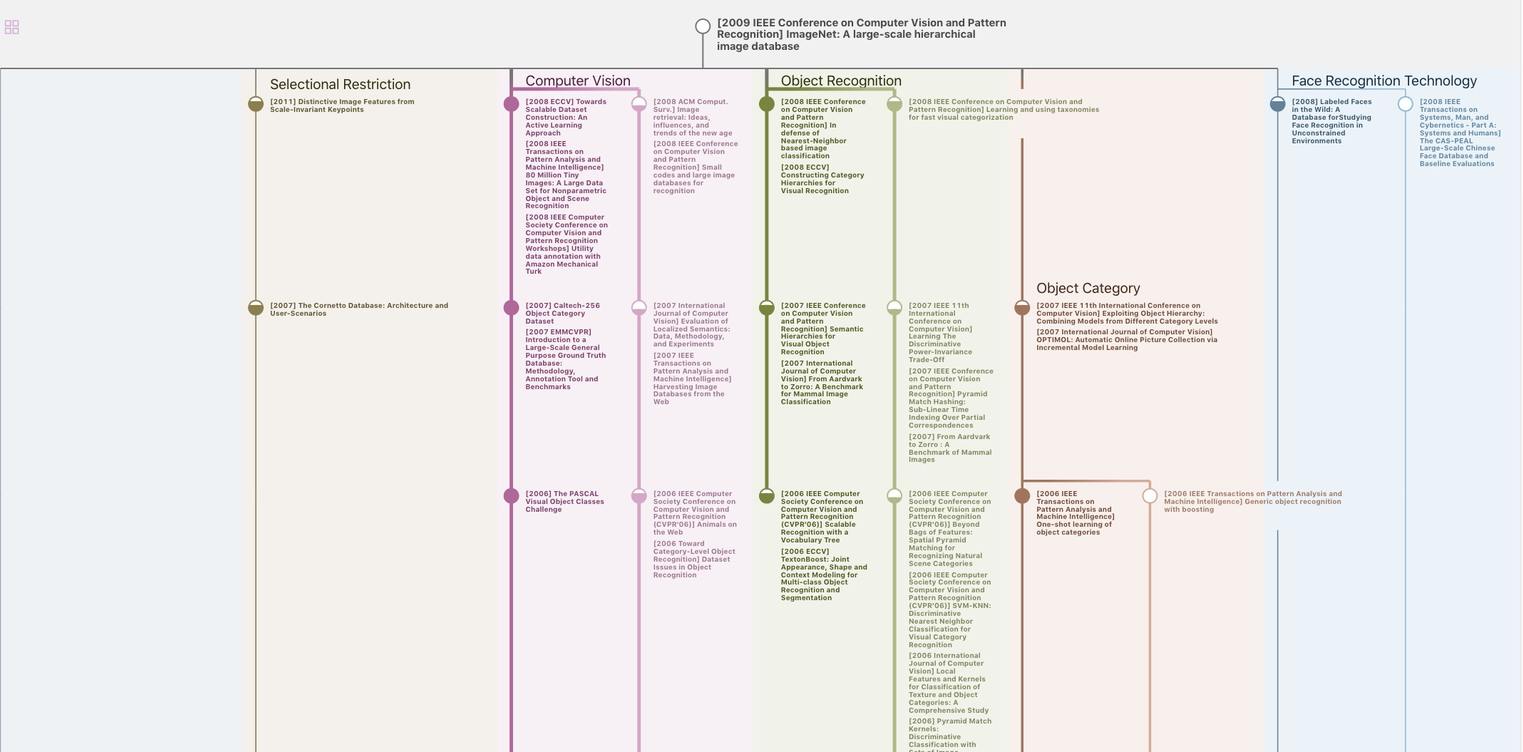
生成溯源树,研究论文发展脉络
Chat Paper
正在生成论文摘要