Fusing Animal Biometrics with Autonomous Robotics: Drone-based Search and Individual ID of Friesian Cattle (Extended Abstract)
2020 IEEE Winter Applications of Computer Vision Workshops (WACVW)(2020)
摘要
This work covers the robotic drone integration of a re-identification system for Friesian Cattle. We have built a computationally-enhanced M100 UAV platform with an on-board deep learning inference system for integrated computer vision and navigation able to autonomously find and visually identify by coat pattern individual Holstein Friesian cattle in freely moving herds. For autonomous drone-based identification we describe an approach that utilises three deep convolutional neural network architectures running live onboard the aircraft; that is, a YoloV2-based species detector, a dual-stream Convolutional Neural Network (CNN) delivering exploratory agency and an InceptionV3-based biometric Long-term Recurrent Convoluational Network (LRCN) for individual animal identification. We evaluate the performance of components offline, and also online via real-world field tests of autonomous low-altitude flight in a farm environment. The presented proof-of-concept system is a successful step towards autonomous biometric identification of individual animals from the air in open pasture environments and inside farms for tag-less AI support in farming and ecology. The work is published in full in IROS 2019 [4].
更多查看译文
关键词
deep convolutional neural network architectures,YoloV2-based species detector,proof-of-concept system,autonomous biometric identification,animal biometrics,autonomous robotics,drone-based search,robotic drone integration,re-identification system,UAV platform,on-board deep learning inference system,computer vision,navigation,coat pattern individual Holstein Friesian cattle,autonomous drone-based identification,inceptionv3-based biometric long-term recurrent convoluational network,farm environment,farming
AI 理解论文
溯源树
样例
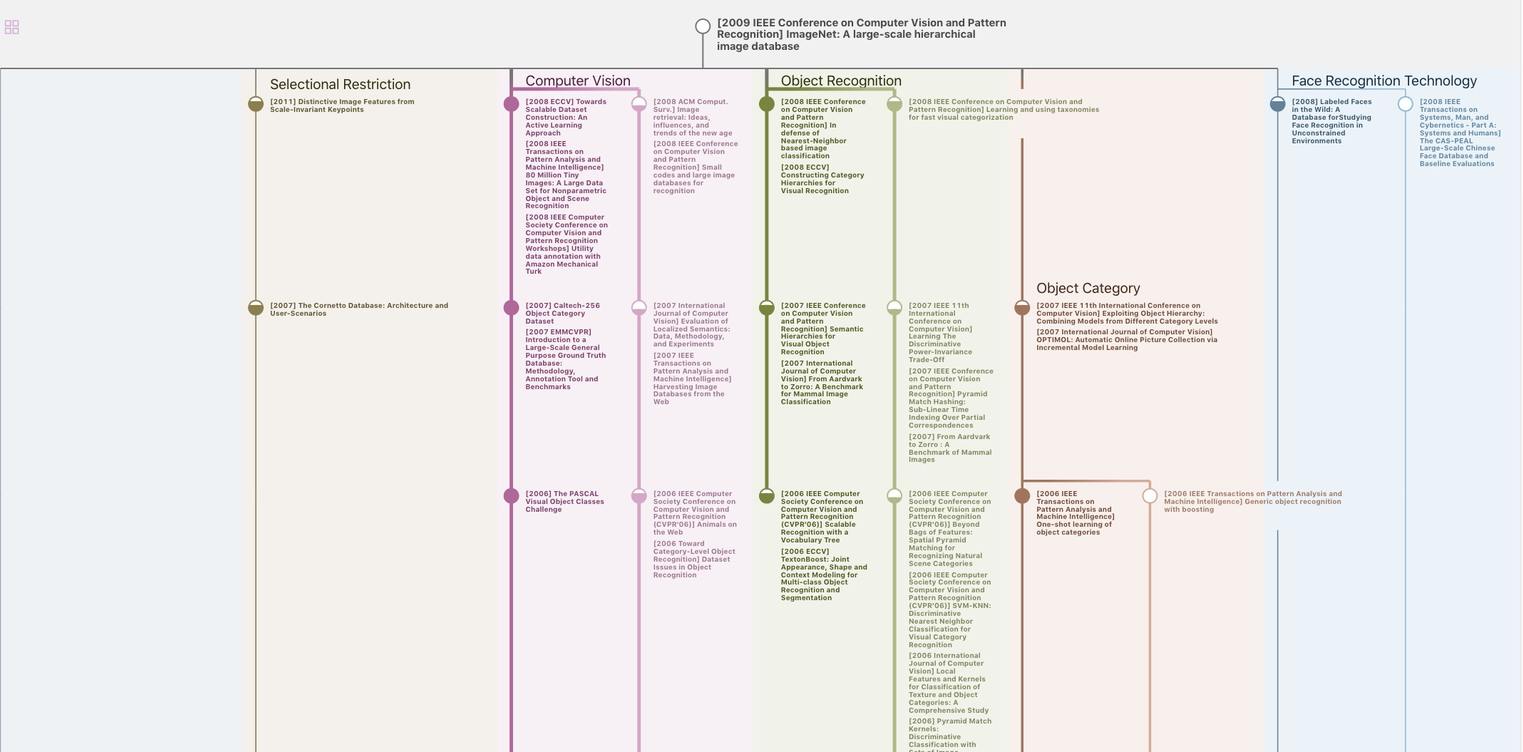
生成溯源树,研究论文发展脉络
Chat Paper
正在生成论文摘要