Deep-Learning based Global and Semantic Feature Fusion for Indoor Scene Classification
2020 IEEE International Conference on Autonomous Robot Systems and Competitions (ICARSC)(2020)
摘要
This paper focuses on the task of RGB indoor scene classification. A single scene may contain various configurations and points of view, but there are a small number of objects that can characterize the scene. In this paper we propose a deep-learning based Global and Semantic Feature Fusion Approach (GSF
2
App) with two branches. In the first branch (top branch), a CNN model is trained to extract global features from RGB images, taking leverage from the ImageNet pre-trained model to initialize our CNN's weights. In the second branch (bottom branch), we develop a semantic feature vector that represents the objects in the image, which are detected and classified through the COCO dataset pre-trained YOLOv3 model. Then, both global and semantic features are combined in an intermediate feature fusion stage. The proposed approach was evaluated on the SUN RGB-D Dataset and NYU Depth Dataset V2 achieving state-of-the-art results on both datasets.
更多查看译文
关键词
Indoor Scene Classification,Deep Learning,RGB,Semantic Features
AI 理解论文
溯源树
样例
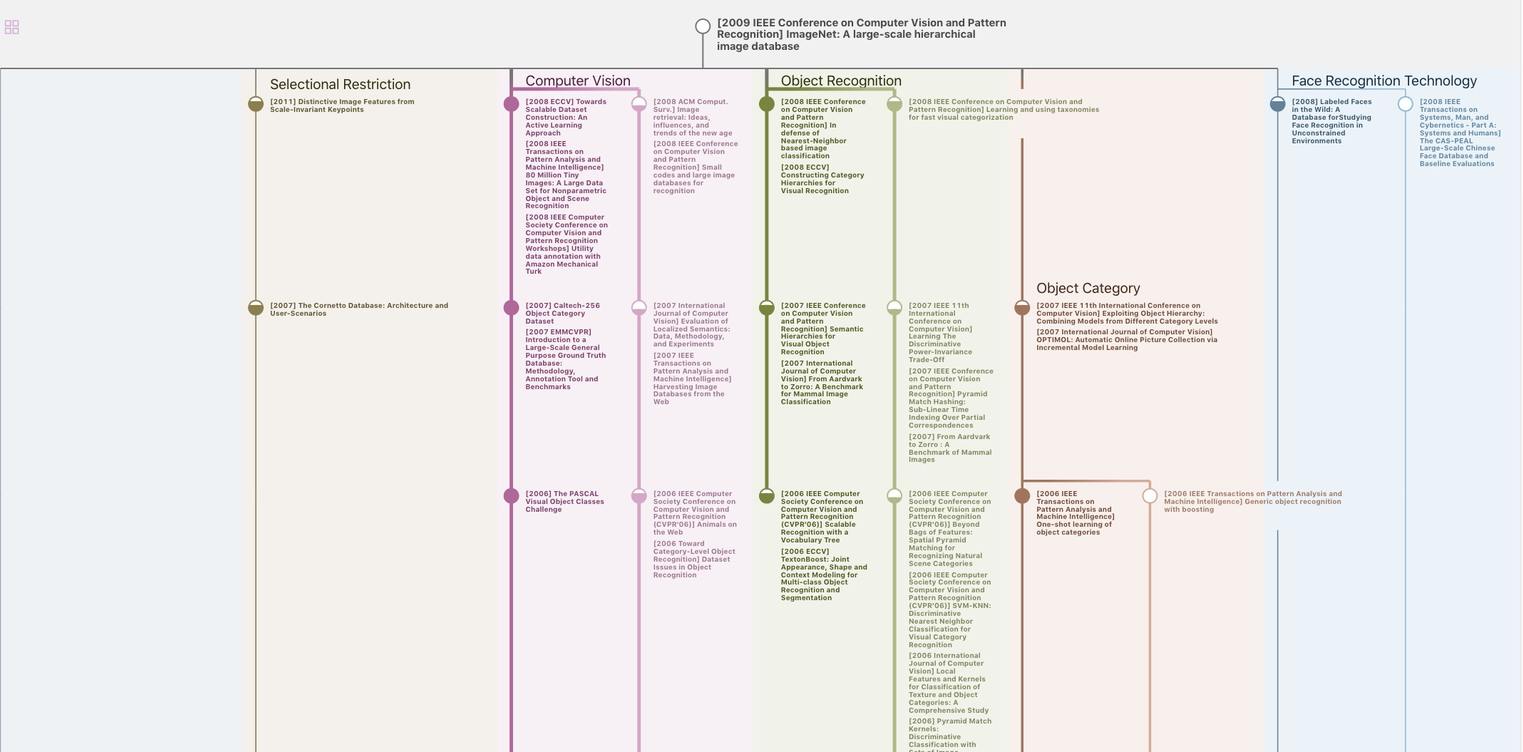
生成溯源树,研究论文发展脉络
Chat Paper
正在生成论文摘要