Geometric Approaches to Discriminative Training
user-5ebe282a4c775eda72abcdce(2016)
摘要
Discriminative training as a general machine learning approach has wide applications in tasks like Natural Language Processing (NLP) and Automatic Speech Recognition (ASR). In this thesis, we are interested in online methods for discriminative training due to their simplicity, efficiency and scalabililty. The novel methods we propose are summarized as follows. First, an interesting subclass of online learning algorithms adopts multiplicative instead of additive strategies to update parameters of linear models, but none of them can be directly used for structured prediction as required by many NLP tasks. We extend the multiplicative Winnow algorithm to a structured version, and the additive MIRA algorithm to a multiplicative version, and apply the them to NLP tasks. We also give interpretations to the relationship between EG and prod, two multiplicative algorithms, from an information geometric perspective. Secondly, although general online learning frameworks, notably the Online Mirror Descent (OMD), exist and subsume many specific algorithms, they are not suitable for deriving multiplicative algorithms. We therefore propose a new general framework named Generalized Multiplicative Update (GMU) that is multiplicative in nature and easily derives many specific multiplicative algorithms. We then propose a subclass of GMU, named the q-Exponentiated Gradient (qEG) method, that elucidates the relationship among several of the algorithms. To better understand the difference between OMD and GMU, we give further analysis of these algorithms from a Riemannian geometric perspective. We also extend OMD and GMU to accelerated versions …
更多查看译文
AI 理解论文
溯源树
样例
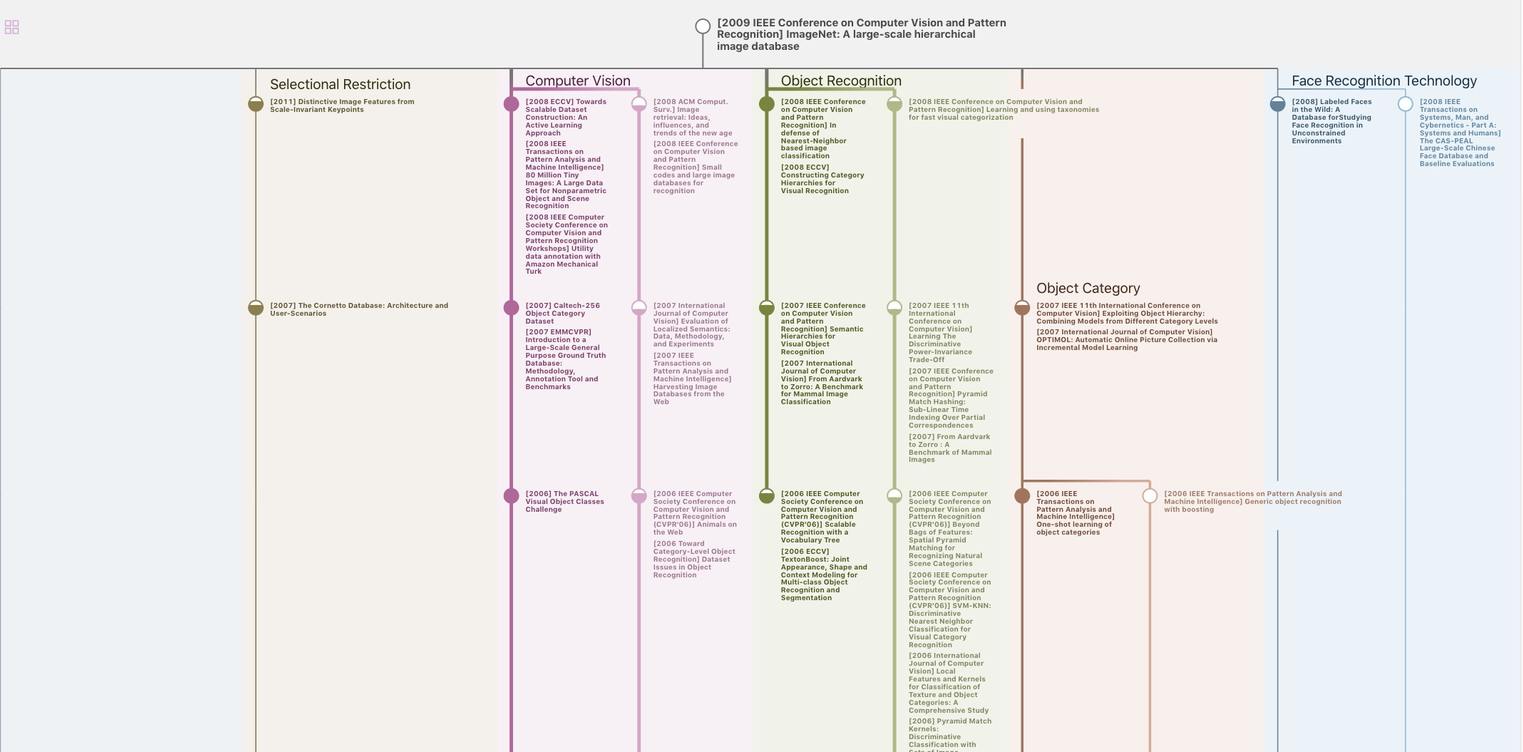
生成溯源树,研究论文发展脉络
Chat Paper
正在生成论文摘要