Clear-Air Anomaly Masking Using Kalman Temporal Filter From Geostationary Multispectral Imagery
IEEE Transactions on Geoscience and Remote Sensing(2020)
摘要
An anomaly detection and estimation technique, using a pixel-based modified version of the Kalman temporal filter, is presented. To detect the anomalies of the observed variable, the proposed Kalman-based anomaly masking (KAM) algorithm relies on the background state models of the expected measurement cycle of each pixel in nominal (abnormal) conditions. If the measurement significantly deviates from its expected value as predicted by a priori state, an anomaly is identified. The KAM algorithm also provides an a priori estimate of the nominal scenario, exploiting the previous Kalman filter states. The product is an equivalent clear-air observation, expected to be measured in the absence of anomaly (e.g., in the absence of cloud coverage). The KAM algorithm exhibits a general applicability, since its estimates are empirically computed from pixel-based models and its thresholds can be set independently of the area of interest. An application of the KAM algorithm to clear-air nominal scenarios is shown using multispectral imagery from the geostationary Spinning Enhanced Visible and Infrared Imager, having 12 visible-infrared channels and repeat cycle of 15 min, on board of the Meteosat satellite. The area of interest covers the whole West Africa for a test period of three months (December 2015 until February 2016). This results in a massive amount of processed pixels (i.e., 1530 × 880 pixels for 96 timeslots per day). A validation of the clear-air KAM algorithm is presented by intercomparing the detection results with the well-known EUMETSAT cloud mask product. The validation shows constant percentages of matching around 90% over the entire period of analysis.
更多查看译文
关键词
Anomaly detection,clear-air masking,environmental monitoring,geostationary satellite observations,global-scale analysis,Kalman filtering,visible-infrared (VIS-IR) measurements
AI 理解论文
溯源树
样例
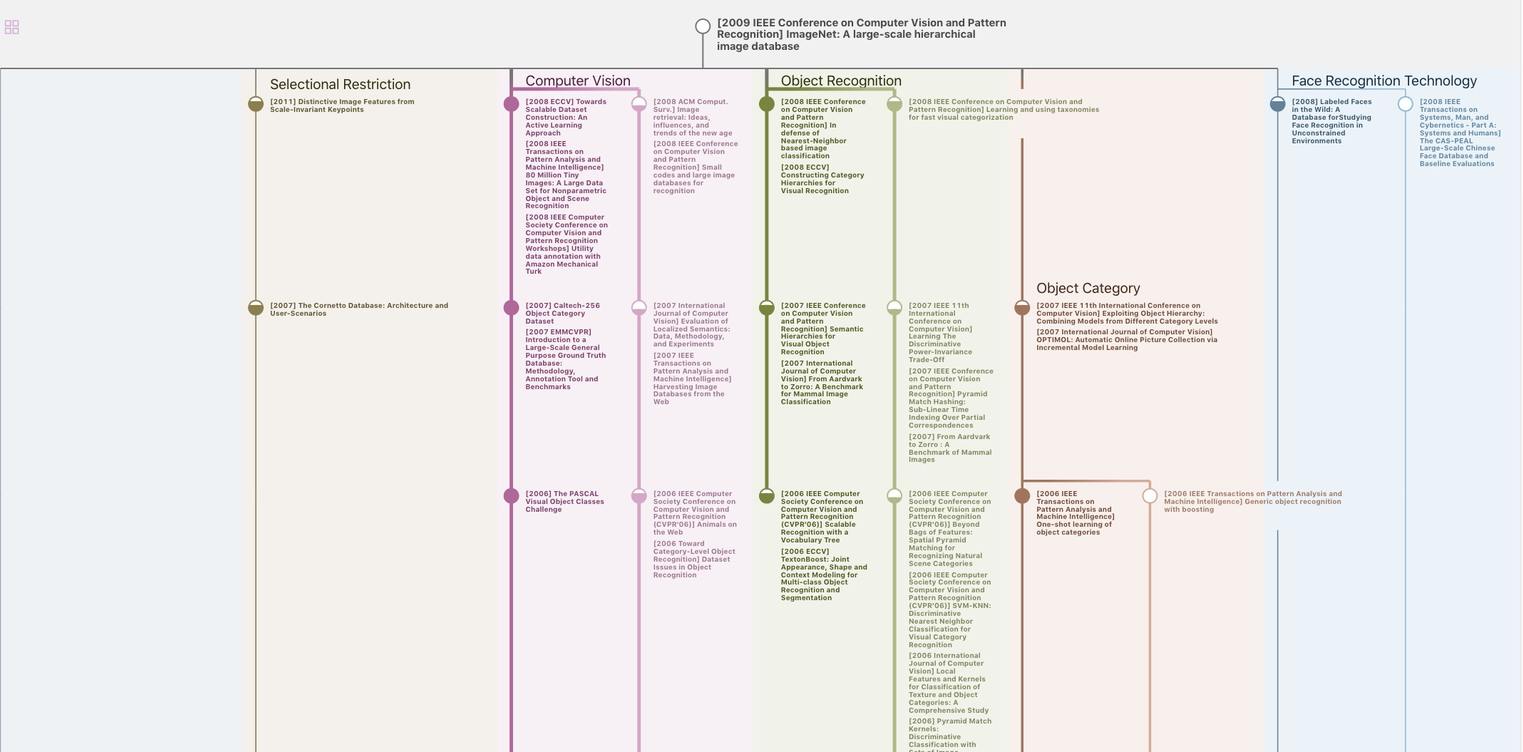
生成溯源树,研究论文发展脉络
Chat Paper
正在生成论文摘要