Multimodal Ground-Based Remote Sensing Cloud Classification via Learning Heterogeneous Deep Features
IEEE Transactions on Geoscience and Remote Sensing(2020)
摘要
Recently, multimodal cloud samples are utilized to learn completed feature representations for cloud classification. However, the existing methods neglect the related information from other multimodal cloud samples in the learning process, which leads to inadequate learning. In this article, we propose a novel deep model to learn heterogeneous deep features (HDFs) for multimodal ground-based remote sensing cloud classification. Specifically, we first design the convolutional neural network (CNN) extractor to combine the visual information and the multimodal information (MI) to obtain the CNN-based features of multimodal cloud samples. Afterward, we treat the CNN-based features of multimodal cloud samples as the nodes of graph, and utilize the similarity between nodes as the adjacency matrix. We feed the graph and the adjacency matrix into the graph convolutional network (GCN) extractor to obtain the GCN-based features that could capture correlations among multimodal cloud samples using graph convolutional layers. After obtaining CNN-based features and GCN-based features, we concatenate the two kinds of heterogeneous features to represent the multimodal cloud samples. As a result, the concatenated feature contains the visual information, the MI and the related information among multimodal cloud samples. We conduct a series of experiments on the multimodal ground-based cloud database (MGCD), and the experimental results verify that the proposed HDF outperforms state-of-the-art methods.
更多查看译文
关键词
Convolutional neural network (CNN),graph convolutional network (GCN),heterogeneous features,multimodal ground-based remote sensing cloud classification
AI 理解论文
溯源树
样例
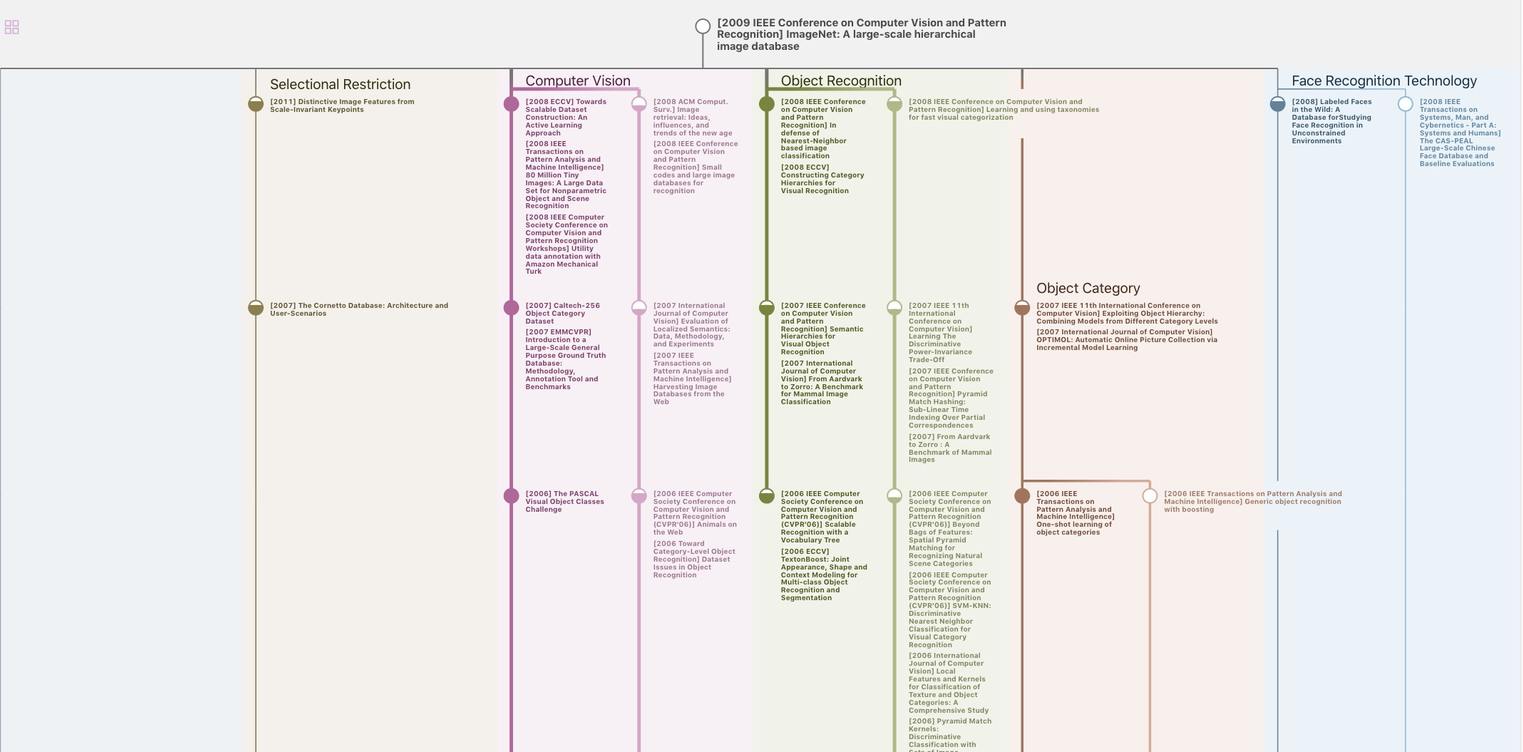
生成溯源树,研究论文发展脉络
Chat Paper
正在生成论文摘要