Atomic column heights detection in metallic nanoparticles using deep convolutional learning
Computational Materials Science(2020)
摘要
The physico-chemical and mechanical properties of metallic (e.g., Au, Pt) nanoparticle complexes highly depend on the order and the distribution of their atomic structure. While transmission electron microscopy (TEM) provides the highest spatial resolution to an image of the nanoparticle’s atomic structure, it is time consuming and cumbersome to estimate the atomic column height from the two-dimensional projected TEM images. With continued progress of in-situ or operando TEM techniques in discovery of nanoscale science, it is paramount to develop artificial intelligence approaches that can be integrated with real-time TEM imaging. In this work, we present a modeling framework based upon deep learning approach (i.e., convolutional neural network – CNN) for the detection of the atomic column heights in the experimental high-resolution transmission electron microscopy (HRTEM) images of gold nanoparticles of different sizes. For this purpose, we propose a method for the generation of the training dataset based on the Wulff construction, in order to bring a physically realistic treatment to the network’s learning process. Moreover, we introduce a model based on the regression scheme, as a valid alternative to a classification approach reported in the prior literature. In addition to counting atoms in verity of columns and nanoparticles, the model also provides insights concerning the experimental conditions suitable for the appropriate identification of atomic column heights by the neural network. Thus, the developed modeling approach establishes a basis for accelerated or ‘on-the-fly’ analysis of nanoparticles as well as a framework for extending deep learning models to broad applications in nanoscience.
更多查看译文
关键词
Deep learning,Convolutional neural networks,Atomic column heights,Metallic nanoparticles
AI 理解论文
溯源树
样例
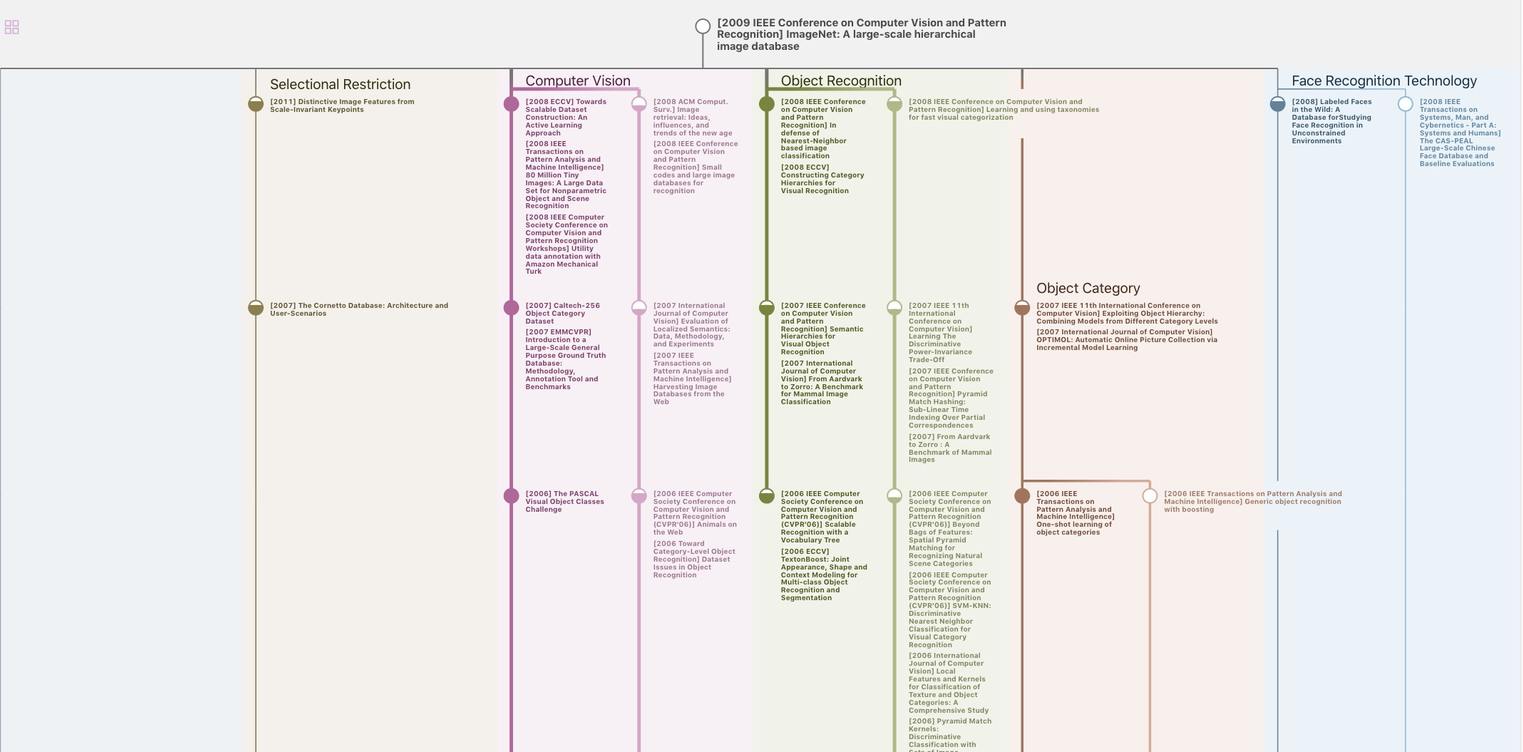
生成溯源树,研究论文发展脉络
Chat Paper
正在生成论文摘要