Multistate Encoding With End-To-End Speech Rnn Transducer Network
2020 IEEE INTERNATIONAL CONFERENCE ON ACOUSTICS, SPEECH, AND SIGNAL PROCESSING(2020)
摘要
Recurrent Neural Network Transducer (RNN-T) models [1] for automatic speech recognition (ASR) provide high accuracy speech recognition. Such end-to-end (E2E) models combine acoustic, pronunciation and language models (AM, PM, LM) of a conventional ASR system into a single neural network, dramatically reducing complexity and model size.In this paper, we propose a technique for incorporating contextual signals, such as intelligent assistant device state or dialog state, directly into RNN-T models. We explore different encoding methods and demonstrate that RNN-T models can effectively utilize such context. Our technique results in reduction in Word Error Rate (WER) of up to 10.4% relative on a variety of contextual recognition tasks. We also demonstrate that proper regularization can be used to model context independently for improved overall quality.
更多查看译文
关键词
E2E ASR, contextual ASR, sequence to sequence
AI 理解论文
溯源树
样例
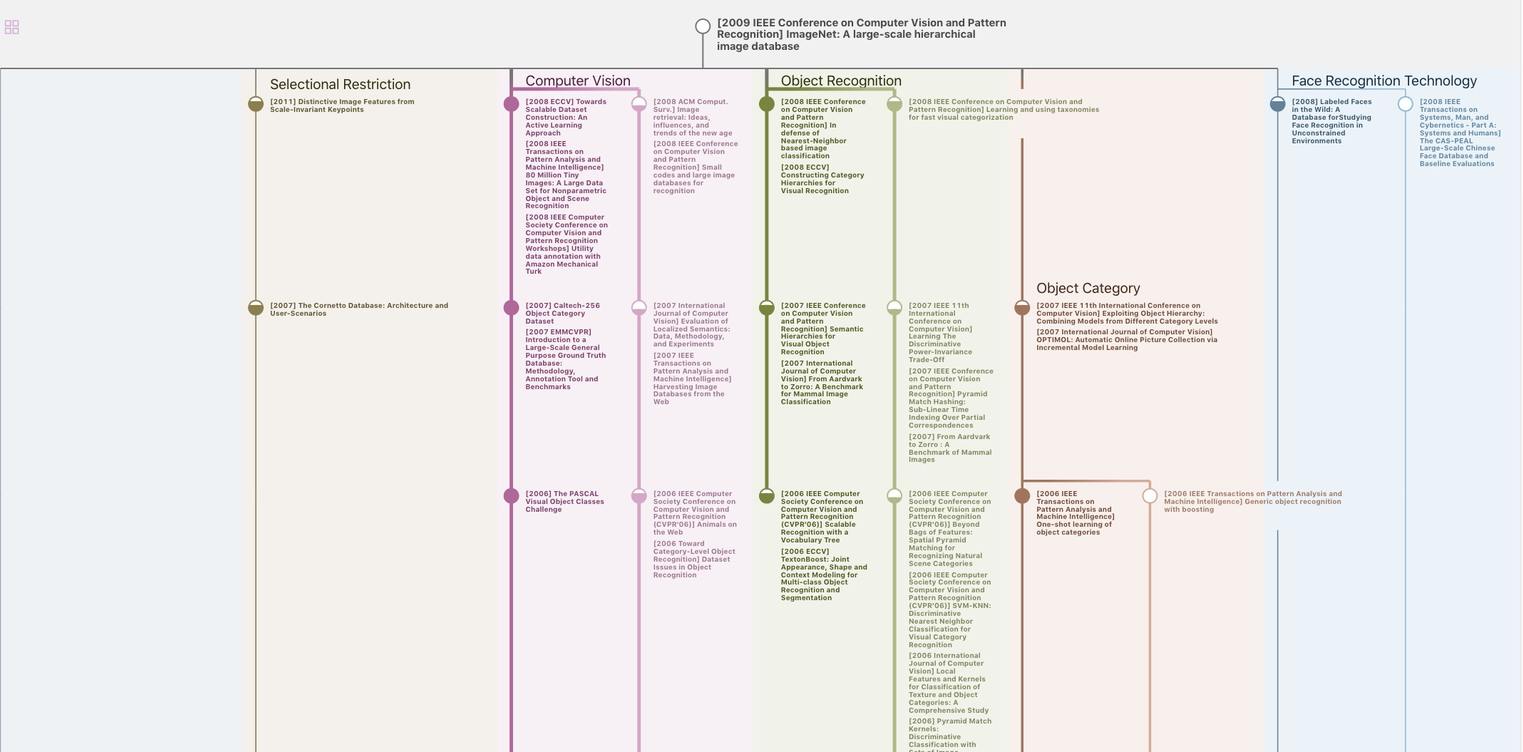
生成溯源树,研究论文发展脉络
Chat Paper
正在生成论文摘要