DETECT: A Hierarchical Clustering Algorithm for Behavioural Trends in Temporal Educational Data
artificial intelligence in education(2020)
摘要
Techniques for clustering student behaviour offer many opportunities to improve educational outcomes by providing insight into student learning. However, one important aspect of student behaviour, namely its evolution over time, can often be challenging to identify using existing methods. This is because the objective functions used by these methods do not explicitly aim to find cluster trends in time, so these trends may not be clearly represented in the results. This paper presents ‘DETECT’ (Detection of Educational Trends Elicited by Clustering Time-series data), a novel divisive hierarchical clustering algorithm that incorporates temporal information into its objective function to prioritise the detection of behavioural trends. The resulting clusters are similar in structure to a decision tree, with a hierarchy of clusters defined by decision rules on features. DETECT is easy to apply, highly customisable, applicable to a wide range of educational datasets and yields easily interpretable results. Through a case study of two online programming courses (\\(N\u003e600\\)), this paper demonstrates two example applications of DETECT: 1) to identify how cohort behaviour develops over time and 2) to identify student behaviours that characterise exercises where many students give up.
更多查看译文
关键词
hierarchical clustering algorithm,behavioural trends,data
AI 理解论文
溯源树
样例
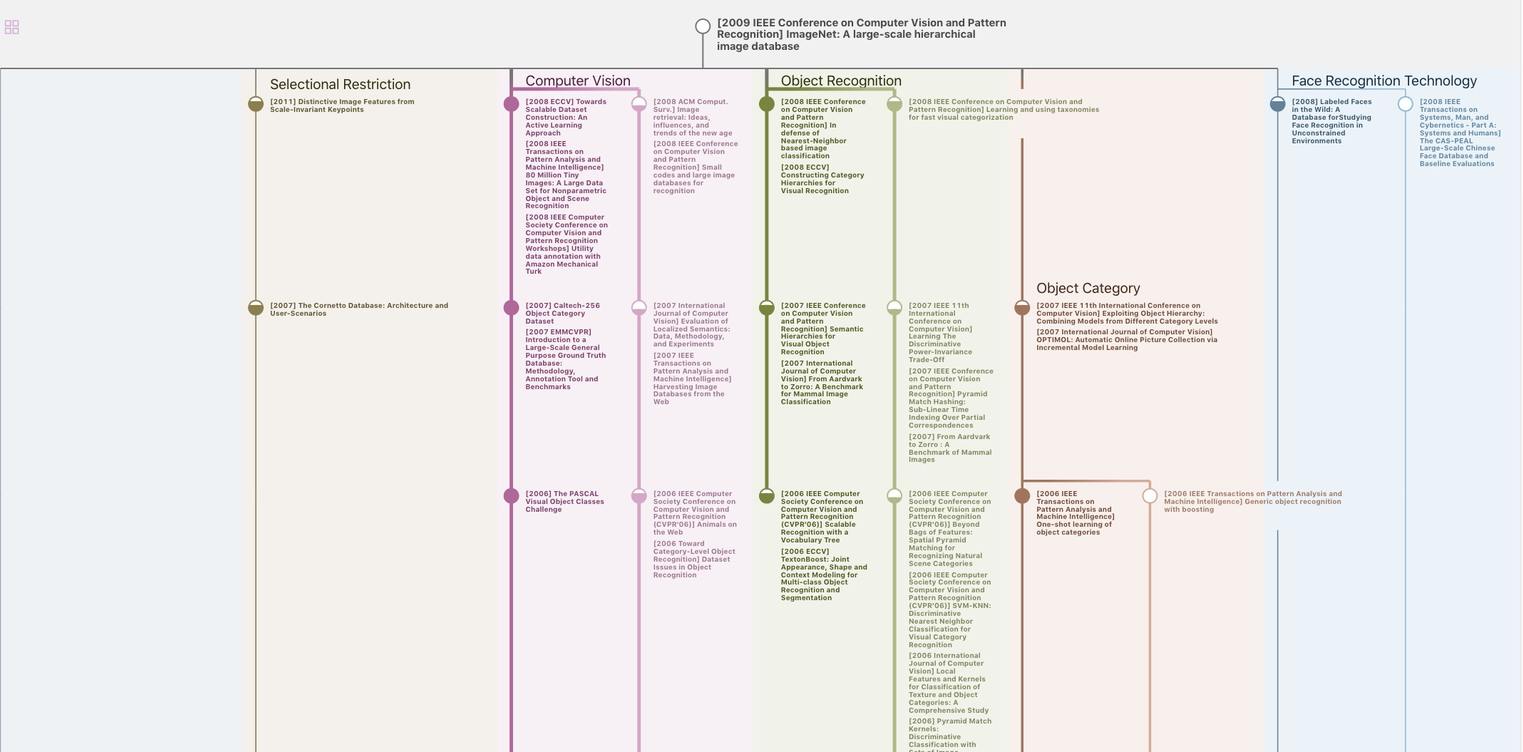
生成溯源树,研究论文发展脉络
Chat Paper
正在生成论文摘要