Overlap Sampler for Region-Based Object Detection
2020 IEEE Winter Conference on Applications of Computer Vision (WACV)(2020)
摘要
The top accuracy of object detection to date is led by region-based approaches, where the per-region stage is responsible for recognizing proposals generated by the region proposal network. In that stage, sampling heuristics (e.g., OHEM, IoU-balanced sampling) is always applied to select a part of examples during training. But nowadays, existing samplers ignore the overlaps among examples, which may result in some low-quality predictions preserved. To mitigate the issue, we propose Overlap Sampler that selects examples according to the overlaps among examples, which enables the training to focus on the important examples. Benefitted from it, the Faster R-CNN could obtain impressively 1.5 points higher Average Precision (AP) on the challenging COCO benchmark, a state-of-the-art result among existing samplers for region-based detectors. Moreover, the proposed sampler also yields considerable improvements for the instance segmentation task. Our code is released at https://github.com/ChenJoya/overlap-sampler.
更多查看译文
关键词
region-based object detection,region proposal network,sampling heuristics,IoU-balanced sampling,important examples,region-based detectors,overlap sampler,OHEM,Faster R-CNN,instance segmentation
AI 理解论文
溯源树
样例
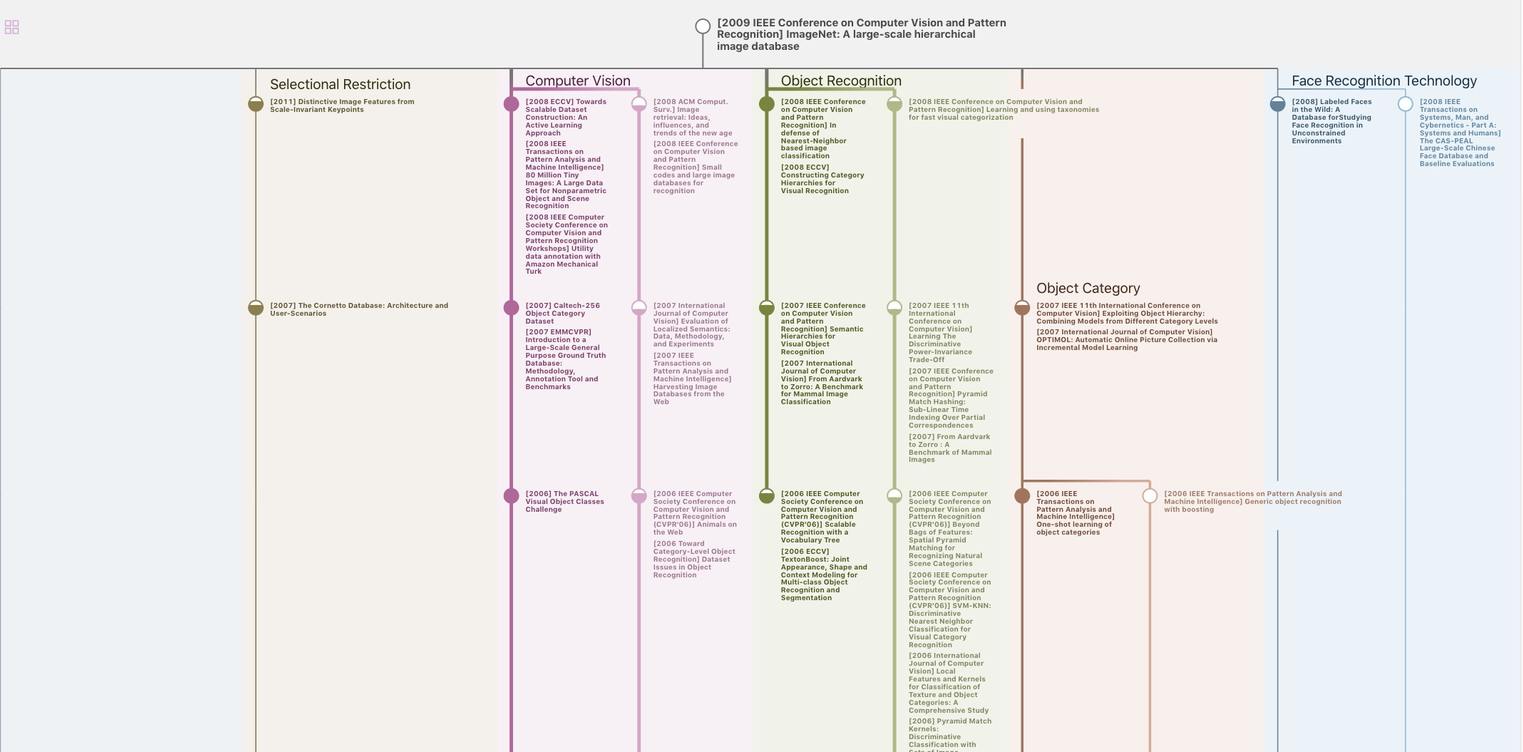
生成溯源树,研究论文发展脉络
Chat Paper
正在生成论文摘要