Challenges in Interpretability of Neural Networks for Eye Movement Data
ETRA '20: 2020 Symposium on Eye Tracking Research and Applications Stuttgart Germany June, 2020(2020)
摘要
Many applications in eye tracking have been increasingly employing neural networks to solve machine learning tasks. In general, neural networks have achieved impressive results in many problems over the past few years, but they still suffer from the lack of interpretability due to their black-box behavior. While previous research on explainable AI has been able to provide high levels of interpretability for models in image classification and natural language processing tasks, little effort has been put into interpreting and understanding networks trained with eye movement datasets. This paper discusses the importance of developing interpretability methods specifically for these models. We characterize the main problems for interpreting neural networks with this type of data, how they differ from the problems faced in other domains, and why existing techniques are not sufficient to address all of these issues. We present preliminary experiments showing the limitations that current techniques have and how we can improve upon them. Finally, based on the evaluation of our experiments, we suggest future research directions that might lead to more interpretable and explainable neural networks for eye tracking.
更多查看译文
关键词
Eye tracking, visualization, deep learning, explainable AI
AI 理解论文
溯源树
样例
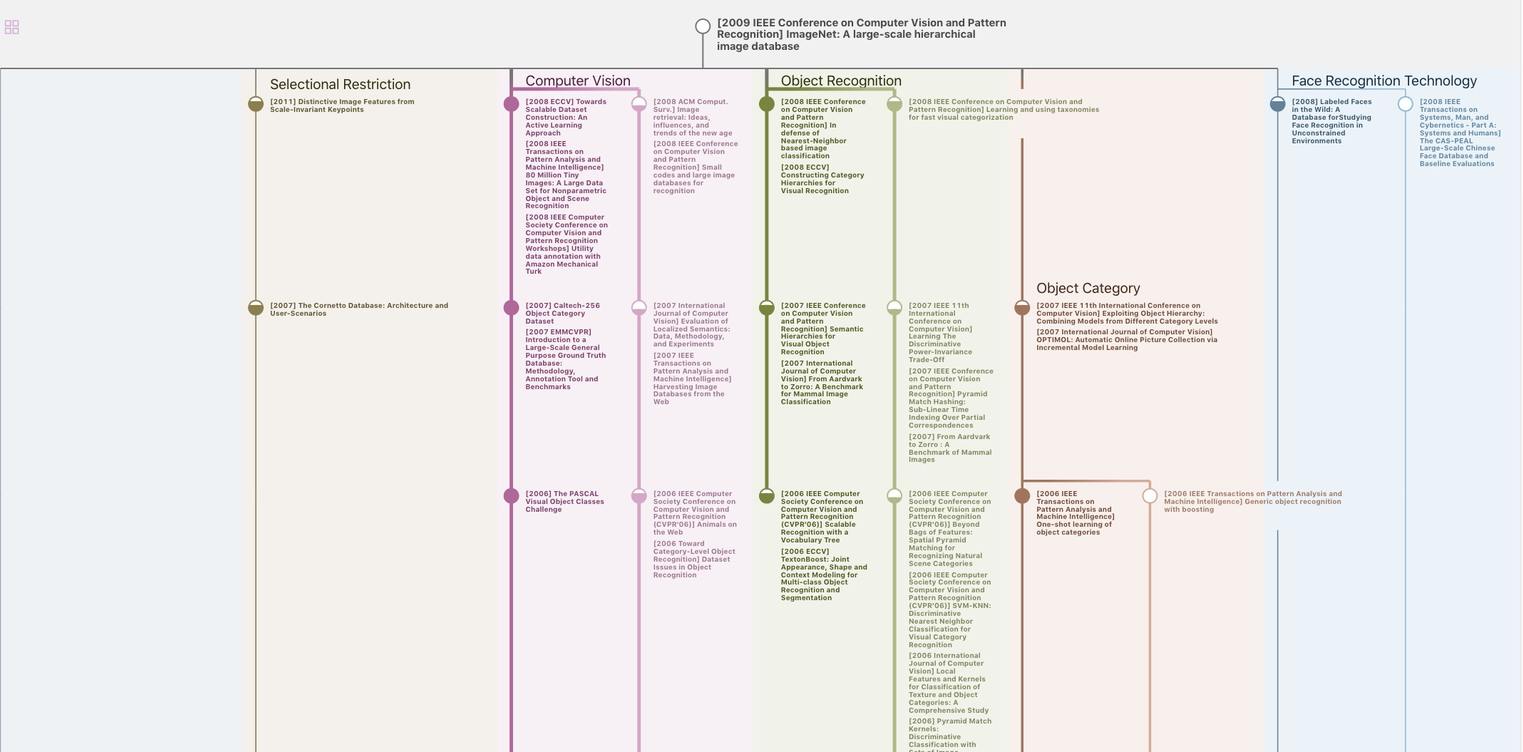
生成溯源树,研究论文发展脉络
Chat Paper
正在生成论文摘要