Evaluation of neural networks with data quantization in low power consumption devices
2020 IEEE 11th Latin American Symposium on Circuits & Systems (LASCAS)(2020)
摘要
Image recognition is frequently carried out using a particular type of neural network called Convolutional Neural Networks (CNNs). Still, in general, any neural network requires powerful computational resources that are expensive and demand very high power consumption. It is a challenge to determine the best low power consumption devices and configuration parameters to train and run CNNs for image processing and recognition. These devices do not have the same capabilities as a computer, and the design and implementation of CNN algorithms should be adapted to reduce their size and computation time without affecting the performance and accuracy of results. Therefore, this research evaluates four CNN models with different data quantization to check their accuracy, processing, memory usage, model complexity, and inference time in four different low-power hardware platforms with varied computational capacity. The results showed that the efficiency obtained by each neural network in the classification tasks increases as the model complexity also increases, but the reduction of the model could also produce acceptable outcomes without critically affecting precision.
更多查看译文
关键词
Convolutional neural networks,FPGA,image recognition
AI 理解论文
溯源树
样例
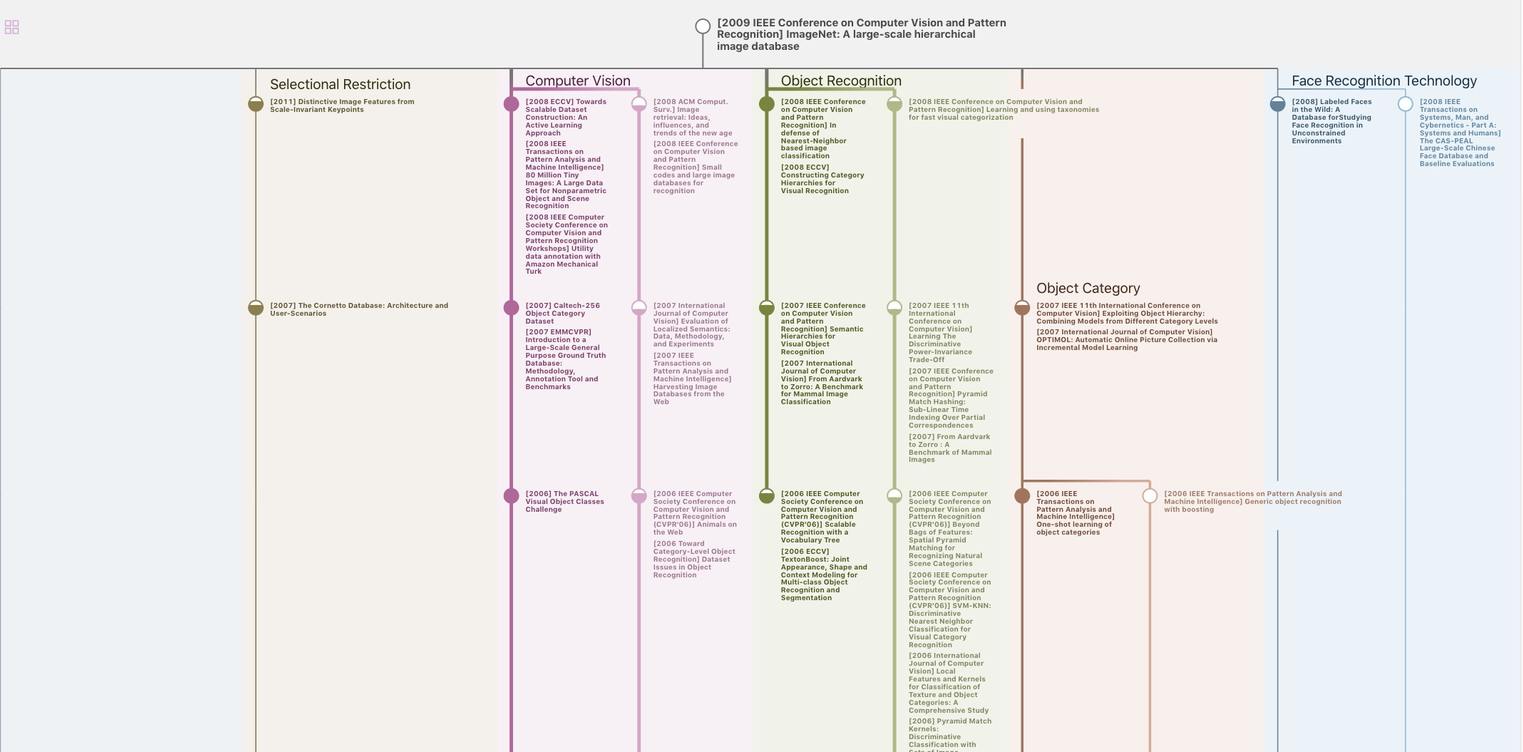
生成溯源树,研究论文发展脉络
Chat Paper
正在生成论文摘要