Budgeted multi-armed bandit models for sample-based grasp planning in the presence of uncertainty
IEEE ICRA(2015)
摘要
Sampling perturbations in shape, state, and control can facilitate grasp planning in the presence of uncertainty arising from noise, occlusions, and surface properties such as transparency and specularities. Monte-Carlo sampling is computationally demanding, even for planar models. We consider an alternative based on the multi-armed bandit (MAB) model for making sequential decisions, which can apply to a variety of uncertainty models. We formulate grasp planning as a “budgeted multi-armed bandit model”(BMAB) with finite stopping time to minimize “simple regret”, the difference between the expected quality of the best grasp and the expected quality of the grasp evaluated at the stopping time. To evaluate MAB-based sampling, we compare it with Monte-Carlo sampling for grasping an uncertain planar object defined by a Gaussian process implicit surface (GPIS), but the method is applicable to other models of uncertainty. We derive distributions on contact points, surface normal, and center of mass and use these solve the associated MAB model, finding that it computes grasps of similar quality and can reduce computation time by an order of magnitude. This suggests a number of new research questions about how MAB can be applied to other models of uncertainty and how different MAB solution techniques can be applied to further reduce computation.
更多查看译文
AI 理解论文
溯源树
样例
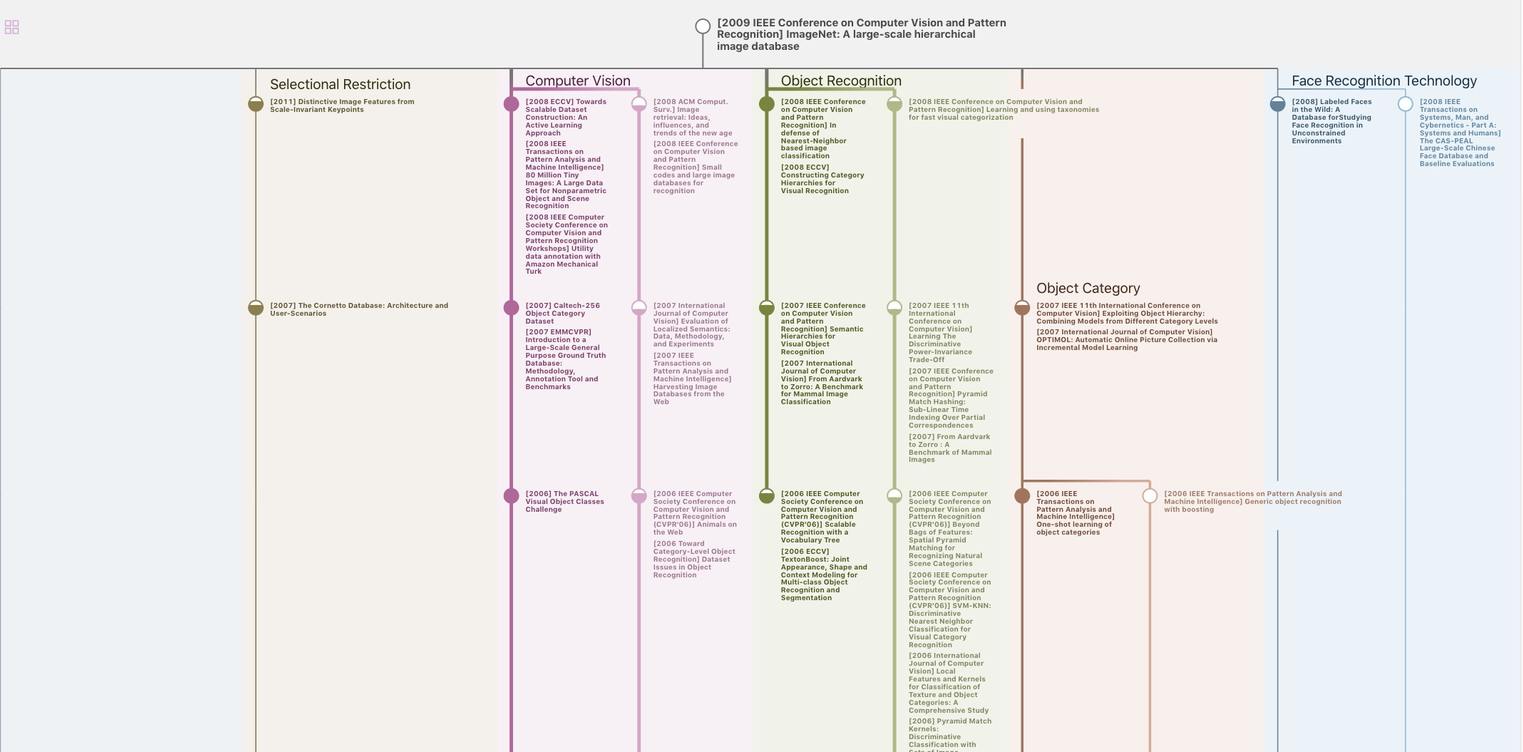
生成溯源树,研究论文发展脉络
Chat Paper
正在生成论文摘要