Unsupervised surgical task segmentation with milestone learning
Proc. Intl Symp. on Robotics Research (ISRR)(2015)
摘要
Surgical tasks are complex multi-step sequences of smaller subtasks (often called surgemes) and it is useful to segment task demonstrations into meaningful subsequences for:(a) extracting finite-state machines for automation,(b) surgical training and skill assessment, and (c) task classification. Existing supervised methods for task segmentation use segment labels from a dictionary of motions to build classifiers. However, as the datasets become voluminous, the labeling becomes arduous and further, this method doesnt generalize to new tasks that dont use the same dictionary. We propose an unsupervised semantic task segmentation framework by learning “milestones”, ellipsoidal regions of the position and feature states at which a task transitions between motion regimes modeled as locally linear. Milestone learning uses a hierarchy of Dirichlet Process Mixture Models, learned through Expectation-Maximization, to cluster the transition points and optimize the number of clusters. It leverages transition information from kinematic state as well as environment state such as visual features. We also introduce a compaction step which removes repetitive segments that correspond to a mid-demonstration failure recovery by retrying an action. We evaluate Milestones Learning on three surgical subtasks: pattern cutting, suturing, and needle passing. Initial results suggest that our milestones qualitatively match manually annotated segmentation. While one-to-one correspondence of milestones with annotated data is not meaningful, the milestones recovered from our method have exactly one annotated surgeme transition in 74%(needle passing) and 66 …
更多查看译文
AI 理解论文
溯源树
样例
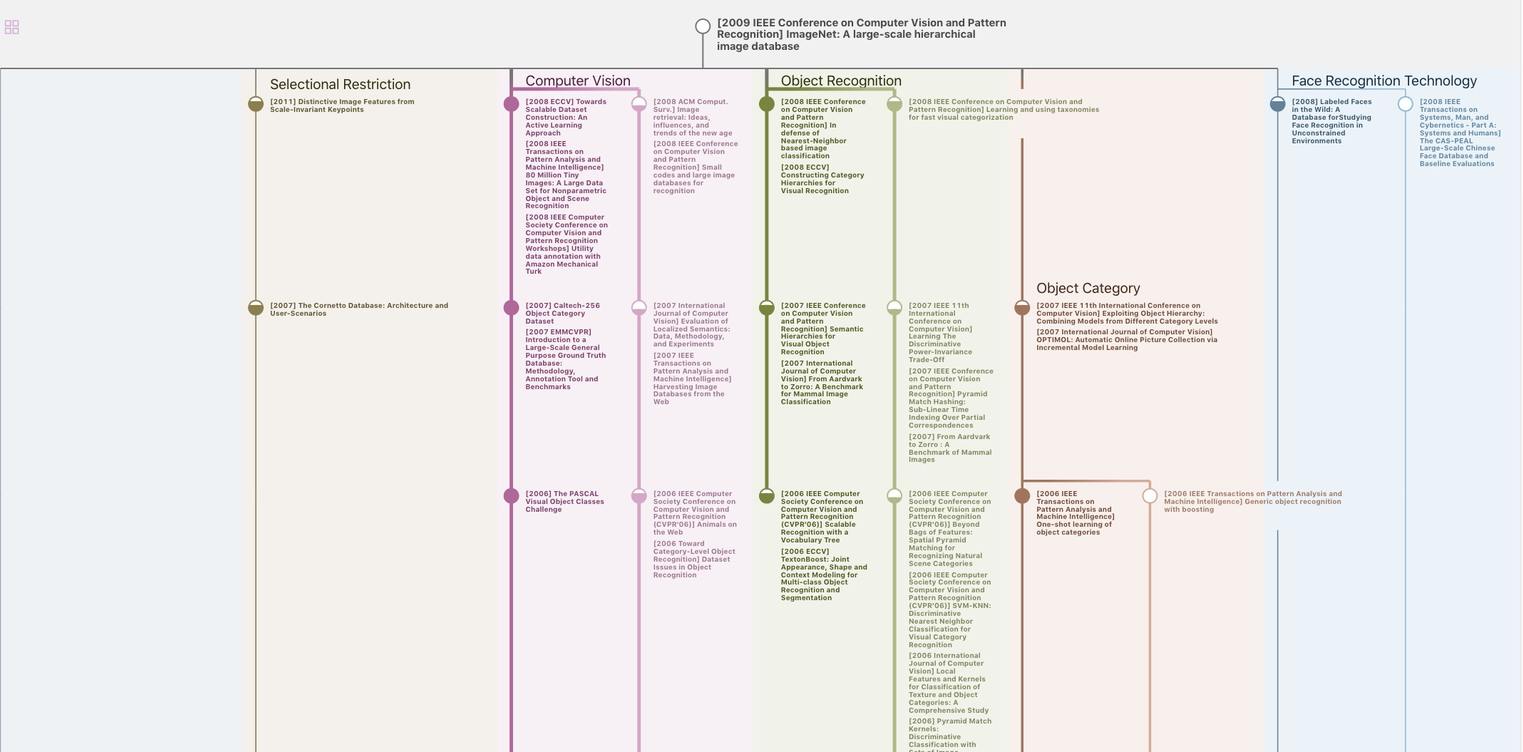
生成溯源树,研究论文发展脉络
Chat Paper
正在生成论文摘要