Efficient and flexible representation of higher-dimensional cognitive variables with grid cells.
PLOS COMPUTATIONAL BIOLOGY(2020)
摘要
Author summary Entorhinal grid cells in mammals are defined by the periodic arrangement of their firing fields and play a major role in the representation of 2D spatial information. At the same time, they represent a variety of non-spatial cognitive variables. It is thus natural to ask what kinds and dimensions of variables it is theoretically possible for grid cells to represent. We show that grid cells can provide a neural vector space for unambiguous integration, memory, and representation of a variety of different (Euclidean) variables of much higher dimension than two, without requiring higher-dimensional grid-like responses. The same circuit can flexibly represent variables of different dimensions, doing so with high dynamic range and at low neural cost, without any reconfiguration of the recurrent circuitry. We shed light on the potential of entorhinal grid cells to efficiently encode variables of dimension greater than two, while remaining faithful to empirical data on their low-dimensional structure. Our model constructs representations of high-dimensional inputs through a combination of low-dimensional random projections and "classical" low-dimensional hexagonal grid cell responses. Without reconfiguration of the recurrent circuit, the same system can flexibly encode multiple variables of different dimensions while maximizing the coding range (per dimension) by automatically trading-off dimension with an exponentially large coding range. It achieves high efficiency and flexibility by combining two powerful concepts, modularity and mixed selectivity, in what we call "mixed modular coding". In contrast to previously proposed schemes, the model does not require the formation of higher-dimensional grid responses, a cell-inefficient and rigid mechanism. The firing fields observed in flying bats or climbing rats can be generated by neurons that combine activity from multiple grid modules, each representing higher-dimensional spaces according to our model. The idea expands our understanding of grid cells, suggesting that they could implement a general circuit that generates on-demand coding and memory states for variables in high-dimensional vector spaces.
更多查看译文
AI 理解论文
溯源树
样例
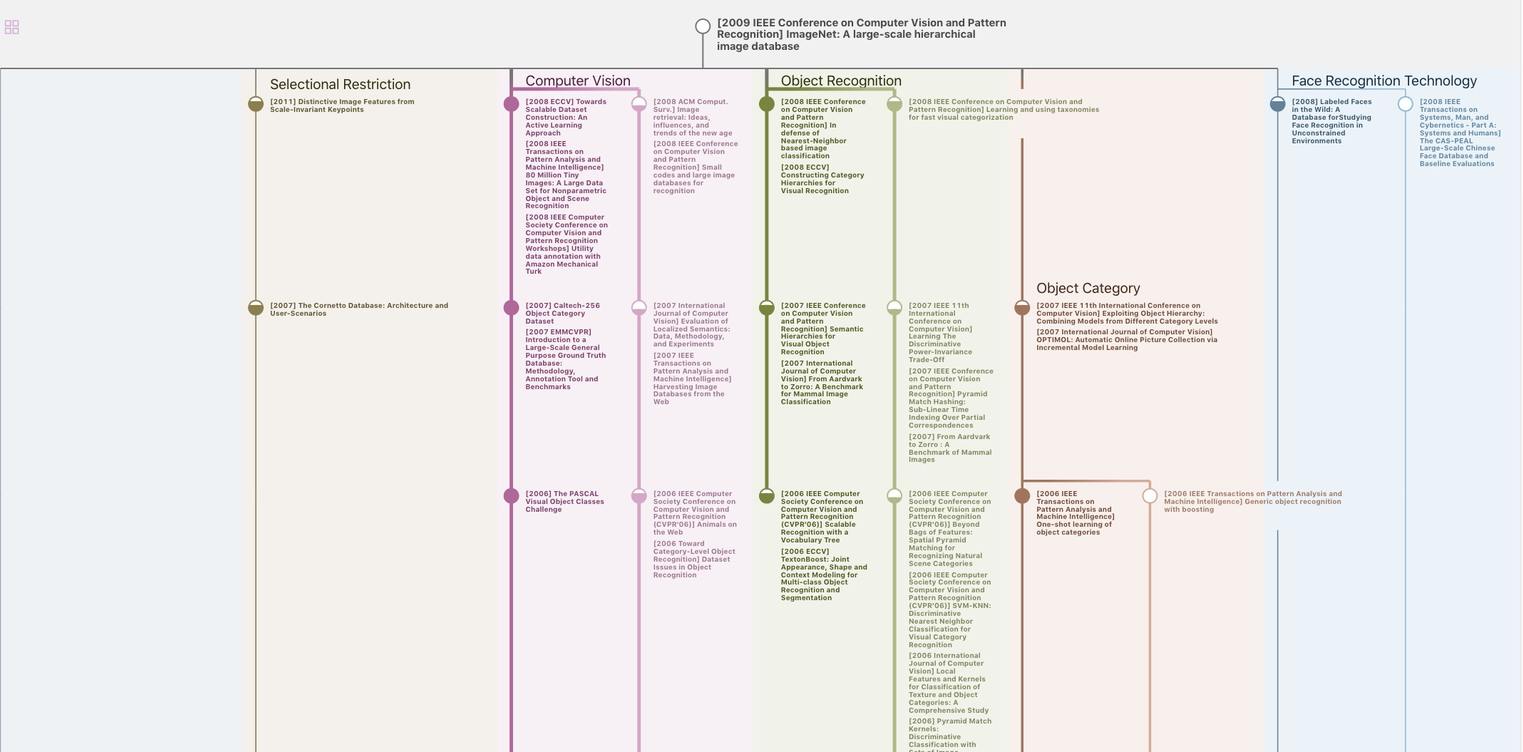
生成溯源树,研究论文发展脉络
Chat Paper
正在生成论文摘要