Climate Adaptation: Reliably Predicting from Imbalanced Satellite Data
2020 IEEE/CVF Conference on Computer Vision and Pattern Recognition Workshops (CVPRW)(2020)
摘要
The utility of aerial imagery (Satellite, Drones) has become an invaluable information source for cross-disciplinary applications, especially for crisis management. Most of the mapping and tracking efforts are manual which is resource-intensive and often lead to delivery delays. Deep Learning methods have boosted the capacity of relief efforts via recognition, detection, and are now being used for non-trivial applications. However the data commonly available is highly imbalanced (similar to other real-life applications) which severely hampers the neural network's capabilities, this reduces robustness and trust. We give an overview on different kinds of techniques being used for handling such extreme settings and present solutions aimed at maximizing performance on minority classes using a diverse set of methods (ranging from architectural tuning to augmentation) which as a combination generalizes for all minority classes. We hope to amplify cross-disciplinary efforts by enhancing model reliability.
更多查看译文
关键词
robustness,minority classes,climate adaptation,aerial imagery,drones,crisis management,tracking efforts,neural network,imbalanced satellite data,deep learning methods
AI 理解论文
溯源树
样例
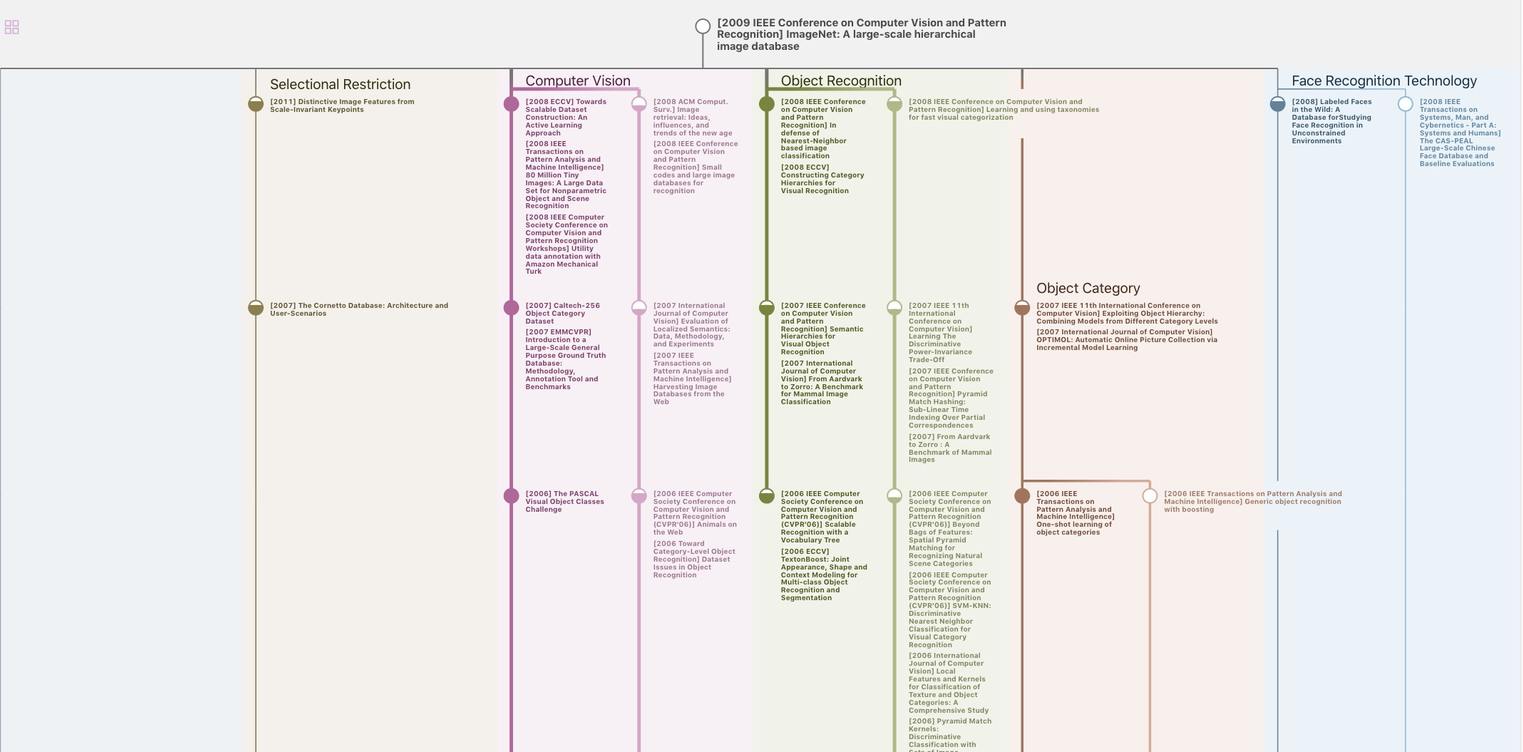
生成溯源树,研究论文发展脉络
Chat Paper
正在生成论文摘要