Fish detection and species classification in underwater environments using deep learning with temporal information
Ecological Informatics(2020)
摘要
It is important for marine scientists and conservationists to frequently estimate the relative abundance of fish species in their habitats and monitor changes in their populations. As opposed to laborious manual sampling, various automatic computer-based fish sampling solutions in underwater videos have been presented. However, an optimal solution for automatic fish detection and species classification does not exist. This is mainly because of the challenges present in underwater videos due to environmental variations in luminosity, fish camouflage, dynamic backgrounds, water murkiness, low resolution, shape deformations of swimming fish, and subtle variations between some fish species. To overcome these challenges, we propose a hybrid solution to combine optical flow and Gaussian mixture models with YOLO deep neural network, an unified approach to detect and classify fish in unconstrained underwater videos. YOLO based object detection system are originally employed to capture only the static and clearly visible fish instances. We eliminate this limitation of YOLO to enable it to detect freely moving fish, camouflaged in the background, using temporal information acquired via Gaussian mixture models and optical flow. We evaluated the proposed system on two underwater video datasets i.e., the LifeCLEF 2015 benchmark from the Fish4Knowledge repository and a dataset collected by The University of Western Australia (UWA). We achieve fish detection F-scores of 95.47% and 91.2%, while fish species classification accuracies of 91.64% and 79.8% on both datasets respectively. To our knowledge, these are the best reported results on these datasets, which show the effectiveness of our proposed approach.
更多查看译文
关键词
Automatic fish detection,Fish species classification,Fish sampling,Biomass estimation,Underwater video imagery,Gaussian mixture models,Optical flow,Deep learning
AI 理解论文
溯源树
样例
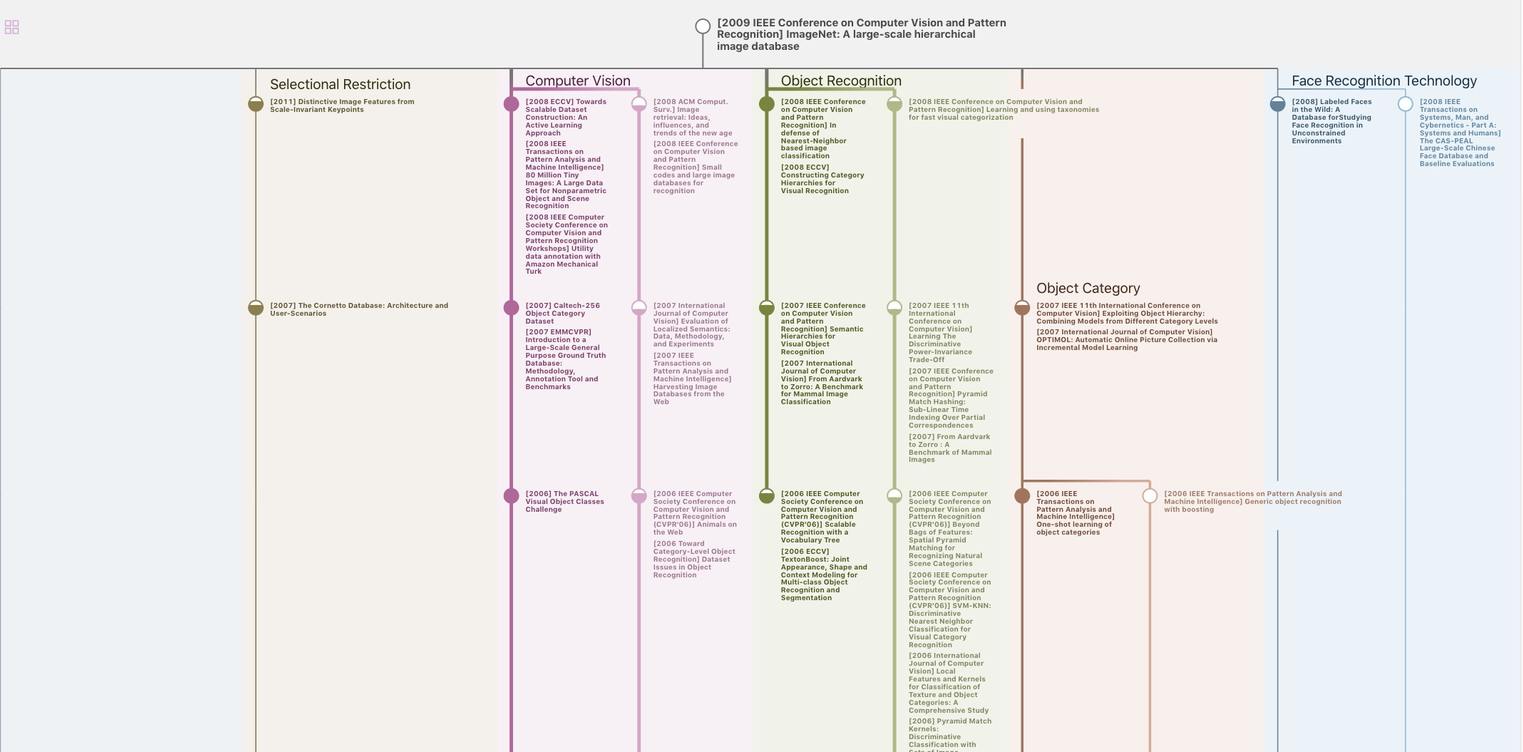
生成溯源树,研究论文发展脉络
Chat Paper
正在生成论文摘要