Learning visual policies for building 3D shape categories
IROS(2020)
摘要
Manipulation and assembly tasks require non-trivial planning of actions depending on the environment and the final goal. Previous work in this domain often assembles particular instances of objects from known sets of primitives. In contrast, we here aim to handle varying sets of primitives and to construct different objects of the same shape category. Given a single object instance of a category, e.g. an arch, and a binary shape classifier, we learn a visual policy to assemble other instances of the same category. In particular, we propose a disassembly procedure and learn a state policy that discovers new object instances and their assembly plans in state space. We then render simulated states in the observation space and learn a heatmap representation to predict alternative actions from a given input image. To validate our approach, we first demonstrate its efficiency for building object categories in state space. We then show the success of our visual policies for building arches from different primitives. Moreover, we demonstrate (i) the reactive ability of our method to re-assemble objects using additional primitives and (ii) the robust performance of our policy for unseen primitives resembling building blocks used during training. Our visual assembly policies are trained with no real images and reach up to 95% success rate when evaluated on a real robot.
更多查看译文
关键词
particular instances,shape category,single object instance,arch,binary shape classifier,visual policy,state policy,discovers new object instances,assembly plans,state space,simulated states,observation space,alternative actions,given input image,object categories,different primitives,re-assemble objects,additional primitives,unseen primitives resembling building blocks,visual assembly policies,building 3D shape categories,assembly tasks
AI 理解论文
溯源树
样例
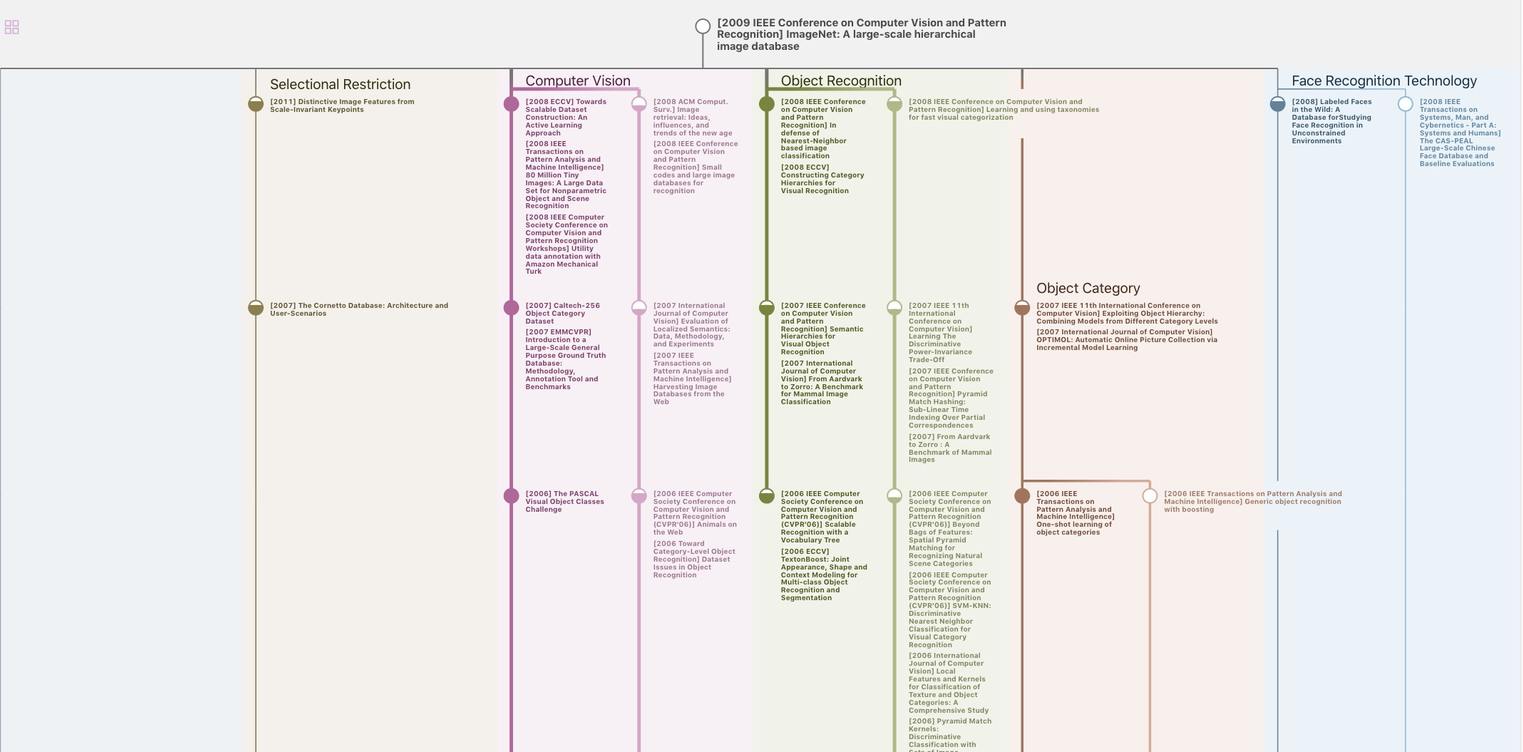
生成溯源树,研究论文发展脉络
Chat Paper
正在生成论文摘要