Hybrid first and second order attention Unet for building segmentation in remote sensing images
Science China Information Sciences(2020)
摘要
Recently, building segmentation (BS) has drawn significant attention in remote sensing applications. Convolutional neural networks (CNNs) have become the mainstream analysis approach in this field owing to their powerful representative ability. However, owing to the variation in building appearance, designing an effective CNN architecture for BS still remains a challenging task. Most of CNN-based BS methods mainly focus on deep or wide network architectures, neglecting the correlation among intermediate features. To address this problem, in this paper we propose a hybrid first and second order attention network (HFSA) that explores both the global mean and the inner-product among different channels to adaptively rescale intermediate features. As a result, the HFSA can not only make full use of first order feature statistics, but also incorporate the second order feature statistics, which leads to more representative feature. We conduct a series of comprehensive experiments on three widely used aerial building segmentation data sets and one satellite building segmentation data set. The experimental results show that our newly developed model achieves better segmentation performance over state-of-the-art models in terms of both quantitative and qualitative results.
更多查看译文
关键词
building segmentation (BS), convolutional neural networks (CNNs), remote sensing, high order pooling, attention
AI 理解论文
溯源树
样例
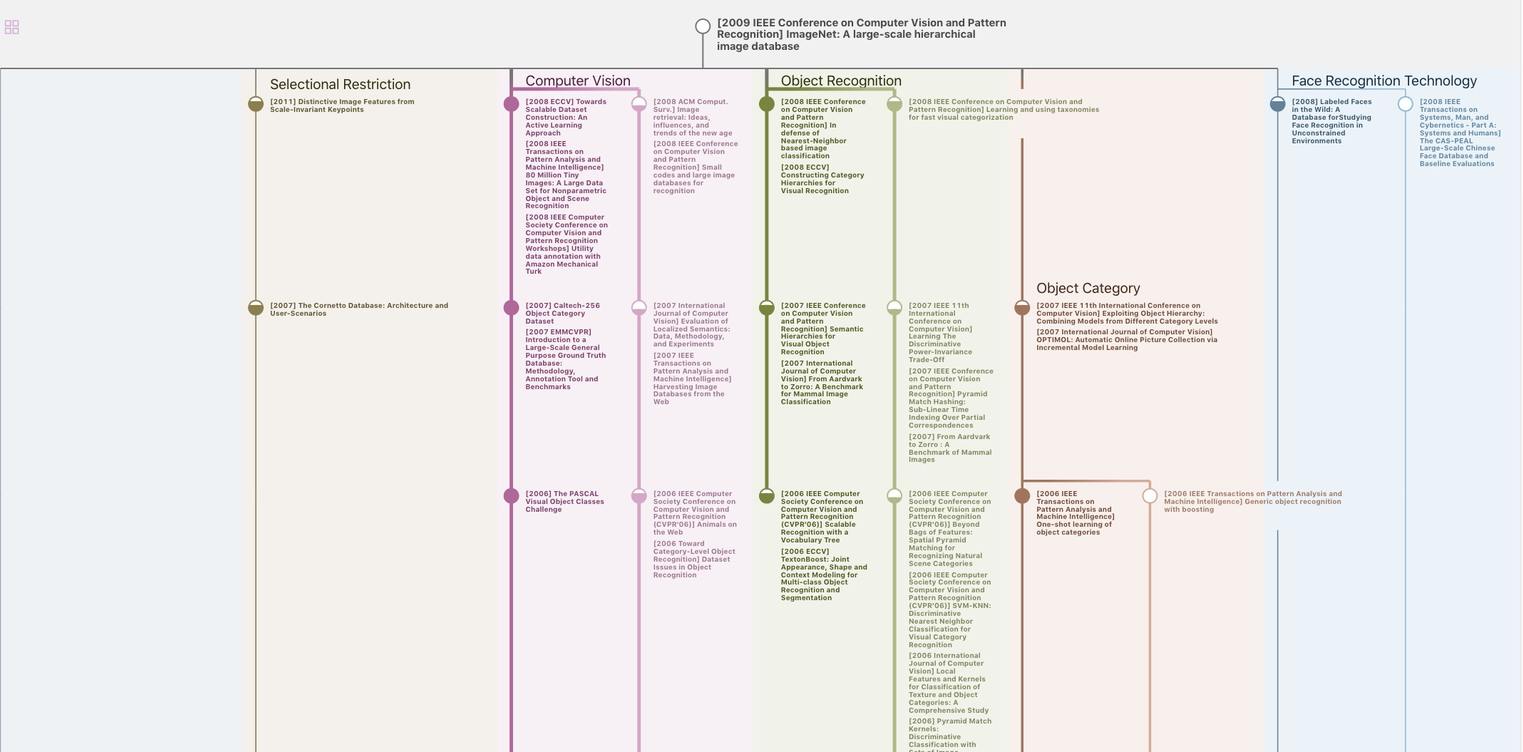
生成溯源树,研究论文发展脉络
Chat Paper
正在生成论文摘要