Switching cluster membership in cluster randomized control trials: Implications for design and analysis.
PSYCHOLOGICAL METHODS(2020)
摘要
Randomized control trials (RCTs) often use clustered designs. where intact clusters (such as classroom. schools, or treatment centers) are randomly assigned to treatment and control conditions. Hierarchical linear models (HLMs) are used almost universally to estimate the effects in such experiments. While study designs that utilize intact clusters have many potential advantages, there is little guidance in the literature on how to respond when cluster switching induces noncompliance with the randomization protocol. In the presence of noncompliance the intent-to-treat (ITT) effect becomes the estimand of interest. When fitting the HLM, these individuals who switch clusters can be assigned to either their as-assigned cluster (the cluster they belonged to at the time of randomization) or their as-treated cluster (the cluster they belonged to at the time the outcome was collected). We show analytically and via simulation. that using the as-treated cluster in HLM will bias the estimate of the ITT effect and using the as-assigned cluster will bias the standard error estimates when heterogeneity among clusters is because of heterogeneity in treatment effects. We show that using linear regression with two-way cluster adjusted standard errors can yield unbiased ITT estimates and consistent standard errors regardless of the source of the random effects. We recommend this method replace HLM as the method of choice for testing intervention effects with cluster-randomized trials with noncompliance and cluster switching. Translational Abstract Randomized control trials (RCTs) often use clustered designs, where intact clusters (such as classrooms. schools, clinics, or treatment centers) are randomly assigned to treatment and control conditions. Hierarchical linear models (HLMs) are used almost universally to estimate the impact of a program or intervention in such experiments. While study designs that utilize intact clusters have many potential advantages, there is little guidance for researchers or practitioners on how to respond when some individuals move between clusters and "break" the randomization protocol. In this case, individuals who switch clusters can be assigned to either their as-assigned cluster (the cluster they belonged to at the time of randomization) or their as-treated cluster (the cluster they belonged to at the time the outcome was collected). We show that using the as-treated cluster in HLM will bias treatment effect estimates and using the as-assigned cluster will bias standard error estimates when there are differences in treatment effects across clusters. We propose an alternative strategy that yields unbiased estimates and appropriate standard errors for cluster-randomized trials with cluster switching.
更多查看译文
关键词
compliance,cluster-randomized trials,intent-to-treat
AI 理解论文
溯源树
样例
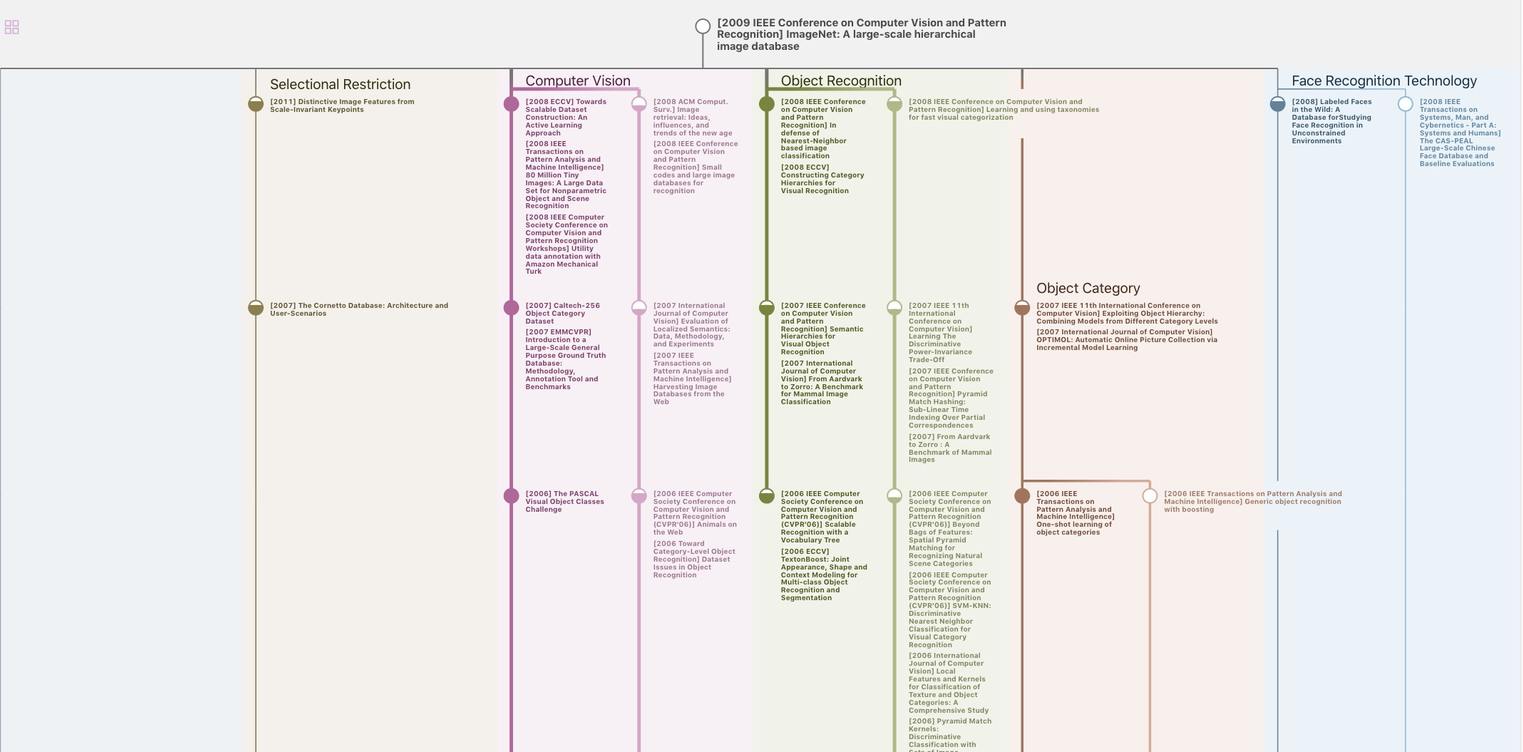
生成溯源树,研究论文发展脉络
Chat Paper
正在生成论文摘要