Normalizing Flows with Multi-Scale Autoregressive Priors
CVPR(2020)
Abstract
Flow-based generative models are an important class of exact inference models that admit efficient inference and sampling for image synthesis. Owing to the efficiency constraints on the design of the flow layers, e.g. split coupling flow layers in which approximately half the pixels do not undergo further transformations, they have limited expressiveness for modeling long-range data dependencies compared to autoregressive models that rely on conditional pixel-wise generation. In this work, we improve the representational power of flow-based models by introducing channel-wise dependencies in their latent space through multi-scale autoregressive priors (mAR). Our mAR prior for models with split coupling flow layers (mAR-SCF) can better capture dependencies in complex multimodal data. The resulting model achieves state-of-the-art density estimation results on MNIST, CIFAR-10, and ImageNet. Furthermore, we show that mAR-SCF allows for improved image generation quality, with gains in FID and Inception scores compared to state-of-the-art flow-based models.
MoreTranslated text
Key words
multiscale autoregressive priors,generative models,inference models,image synthesis,efficiency constraints,split coupling flow layers,long-range data dependencies,autoregressive models,conditional pixel-wise generation,channel-wise dependencies,mAR-SCF,improved image generation quality,flow-based models,normalized flows
AI Read Science
Must-Reading Tree
Example
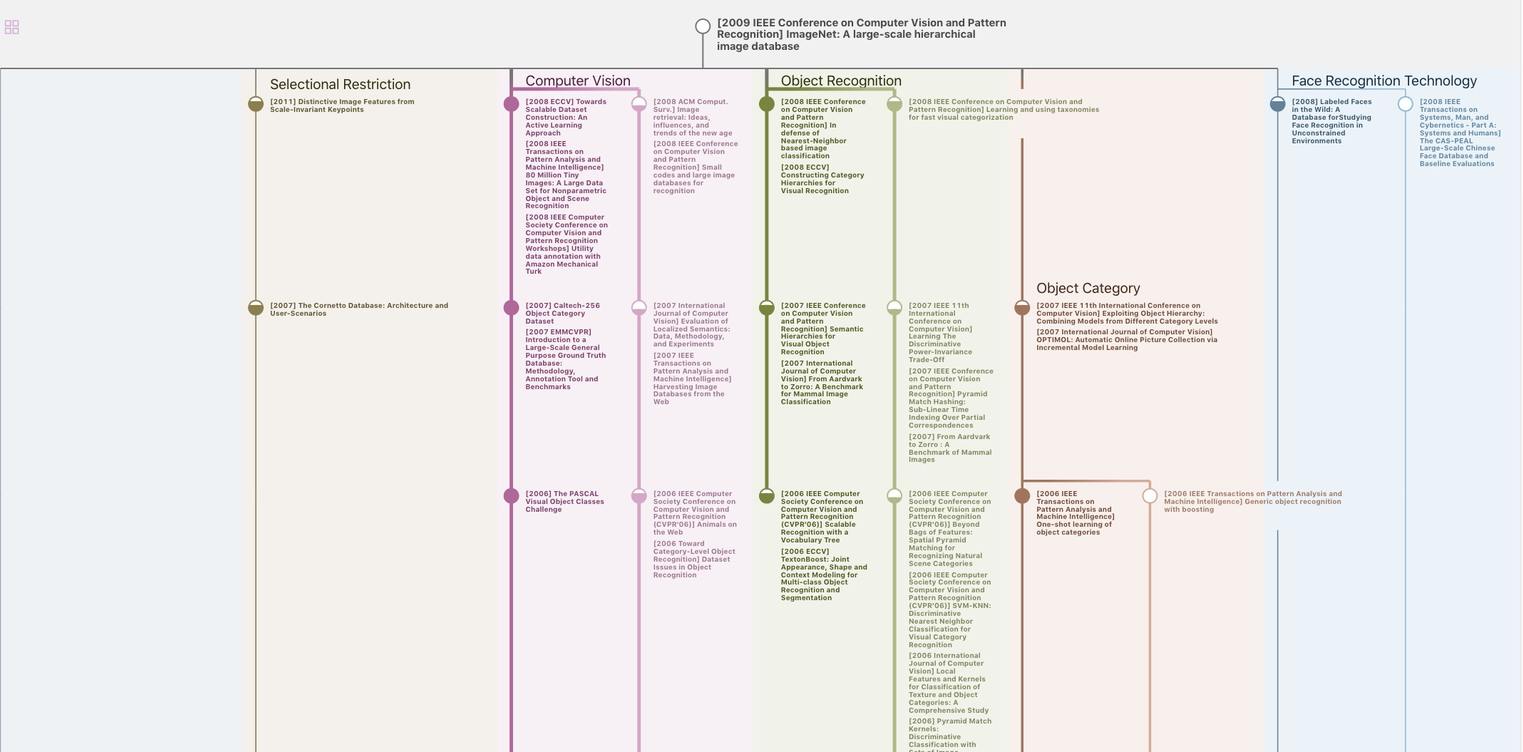
Generate MRT to find the research sequence of this paper
Chat Paper
Summary is being generated by the instructions you defined