Database-Centric Method for Automated High-Throughput Deconvolution and Analysis of Kinetic Antibody Screening Data
SLAS TECHNOLOGY(2017)
摘要
The state-of-the-art industrial drug discovery approach is the empirical interrogation of a library of drug candidates against a target molecule. The advantage of high-throughput kinetic measurements over equilibrium assessments is the ability to measure each of the kinetic components of binding affinity. Although high-throughput capabilities have improved with advances in instrument hardware, three bottlenecks in data processing remain: (1) intrinsic molecular properties that lead to poor biophysical quality in vitro are not accounted for in commercially available analysis models, (2) processing data through a user interface is time-consuming and not amenable to parallelized data collection, and (3) a commercial solution that includes historical kinetic data in the analysis of kinetic competition data does not exist. Herein, we describe a generally applicable method for the automated analysis, storage, and retrieval of kinetic binding data. This analysis can deconvolve poor quality data on-the-fly and store and organize historical data in a queryable format for use in future analyses. Such database-centric strategies afford greater insight into the molecular mechanisms of kinetic competition, allowing for the rapid identification of allosteric effectors and the presentation of kinetic competition data in absolute terms of percent bound to antigen on the biosensor.
更多查看译文
关键词
allosteric effector,HTS,high-throughput screening,automated biology,monoclonal antibody,drug discovery,BLI
AI 理解论文
溯源树
样例
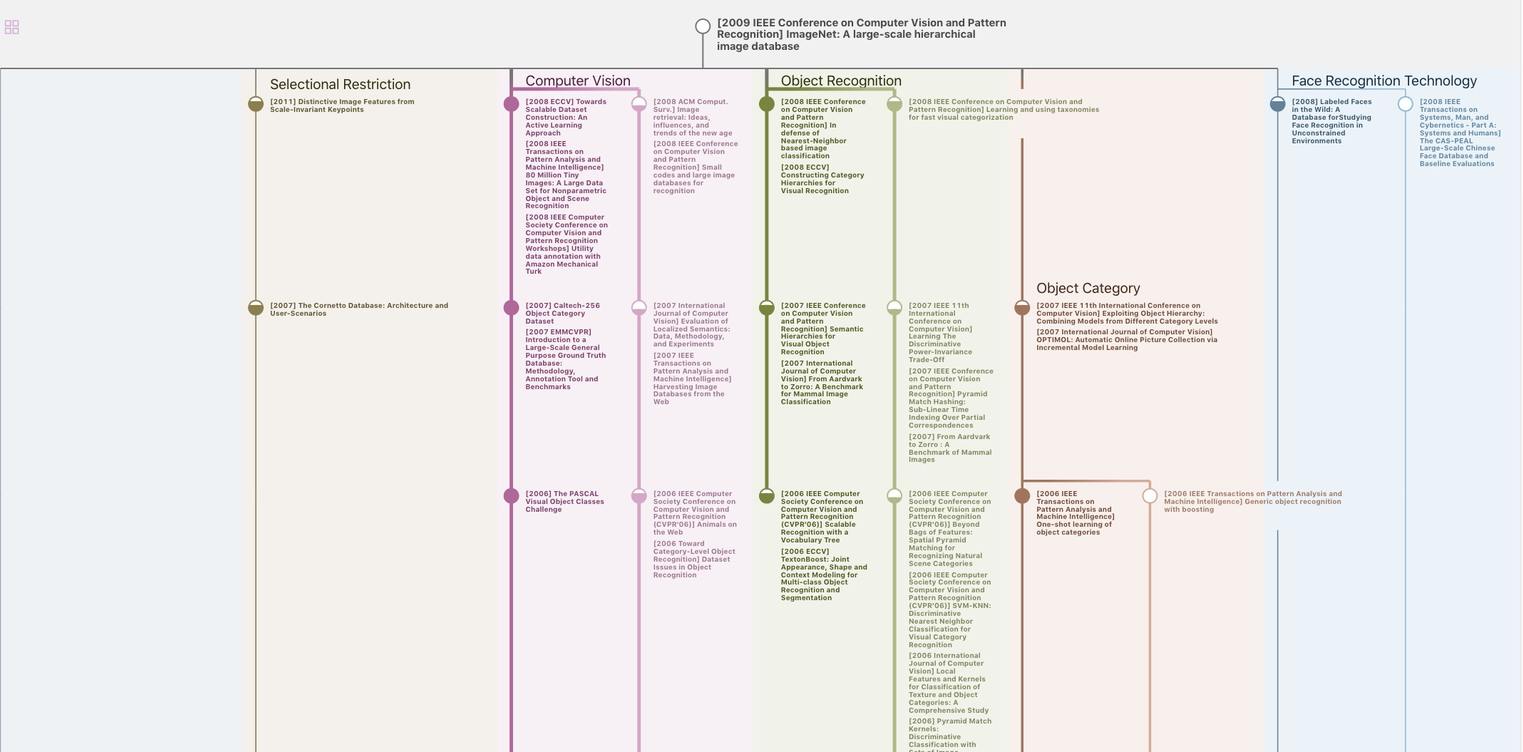
生成溯源树,研究论文发展脉络
Chat Paper
正在生成论文摘要