Learning color space adaptation from synthetic to real images of cirrus clouds
The Visual Computer(2020)
摘要
Cloud segmentation plays a crucial role in image analysis for climate modeling. Manually labeling the training data for cloud segmentation is time-consuming and error-prone. We explore to train segmentation networks with synthetic data due to the natural acquisition of pixel-level labels. Nevertheless, the domain gap between synthetic and real images significantly degrades the performance of the trained model. We propose a color space adaptation method to bridge the gap, by training a color-sensitive generator and discriminator to adapt synthetic data to real images in color space. Instead of transforming images by general convolutional kernels, we adopt a set of closed-form operations to make color-space adjustments while preserving the labels. We also construct a synthetic-to-real cirrus cloud dataset SynCloud and demonstrate the adaptation efficacy on the semantic segmentation task of cirrus clouds. With our adapted synthetic data for training the semantic segmentation, we achieve an improvement of 6.59% when applied to real images, superior to alternative methods.
更多查看译文
关键词
Color space,Synthetic-to-real,Domain adaptation,Cirrus clouds,Segmentation,Style transfer
AI 理解论文
溯源树
样例
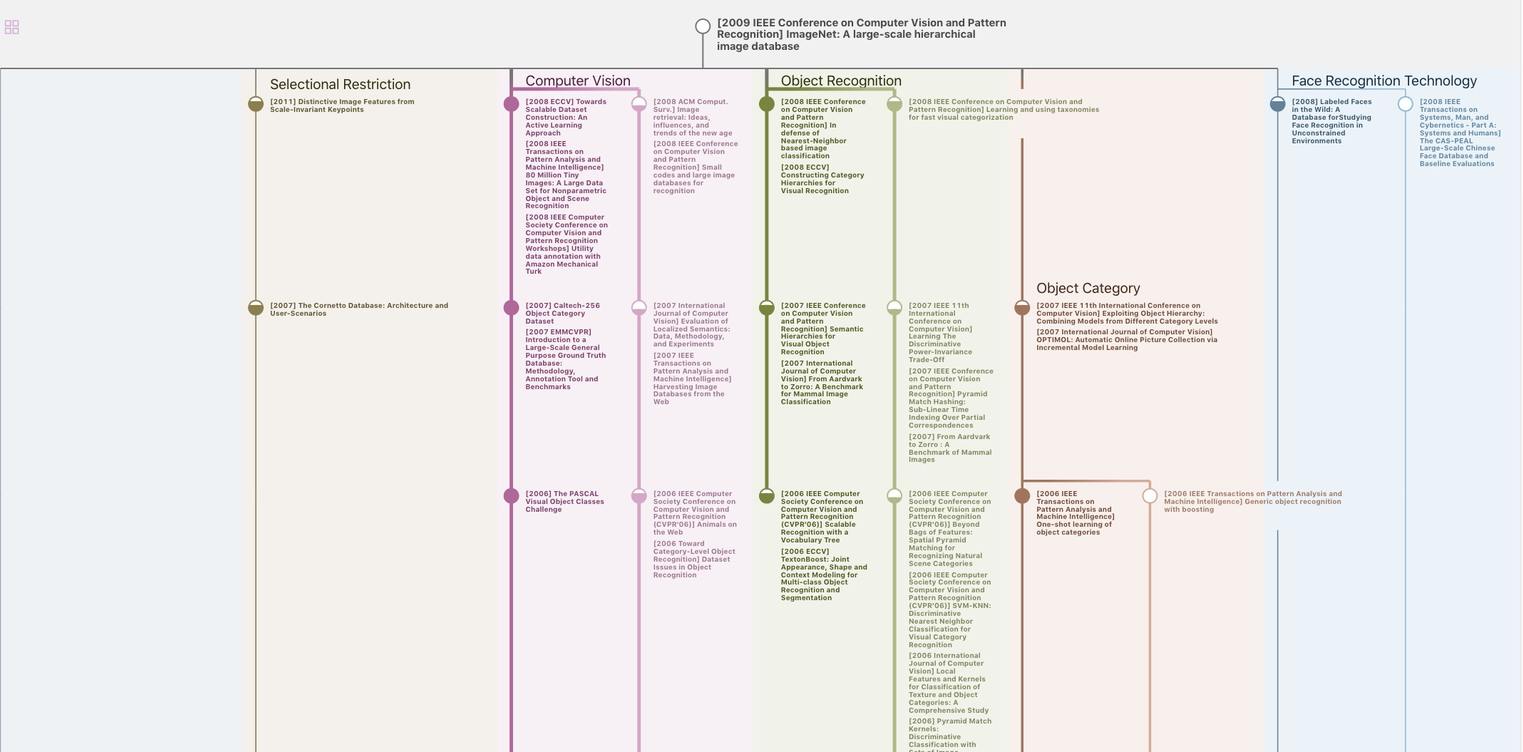
生成溯源树,研究论文发展脉络
Chat Paper
正在生成论文摘要