An End to End Framework With Adaptive Spatio-Temporal Attention Module for Human Action Recognition.
IEEE ACCESS(2020)
摘要
Human action recognition is a challenging task in computer vision. Modeling spatial-temporal information in videos effectively is crucial for the performance improvement of action recognition. In this paper, we introduce an end to end framework, Spatio-Temporal Attention ConvNet (STACNet), which combines two novel attention modules and convolutional neural networks for action recognition. Two novel attention modules, Spatial Attention Module (SAM) and Temporal Attention Module (TAM), are proposed respectively. Spatial Attention Module is established by fusing the value feature and the gradient feature of the feature map, making the representation of ConvNet for action recognition focus on the informative motion regions of actions. Temporal Attention Module is built by combining global average pooling and global max pooling to explore key frames in videos. With the two attention modules, STACNet can adaptively distinguish key frames in a sequence and selectively pay different levels of attention to different spatial motion regions of human actions, at virtually negligible increase in computation cost. We demonstrate the effectiveness of SAM and TAM for action recognition, respectively. The experimental results show that STACNet can obtain superior performance on the datasets of HMDB51 and UCF101.
更多查看译文
关键词
Action recognition,spatial attention,temporal attention,end to end,convolutional neural network
AI 理解论文
溯源树
样例
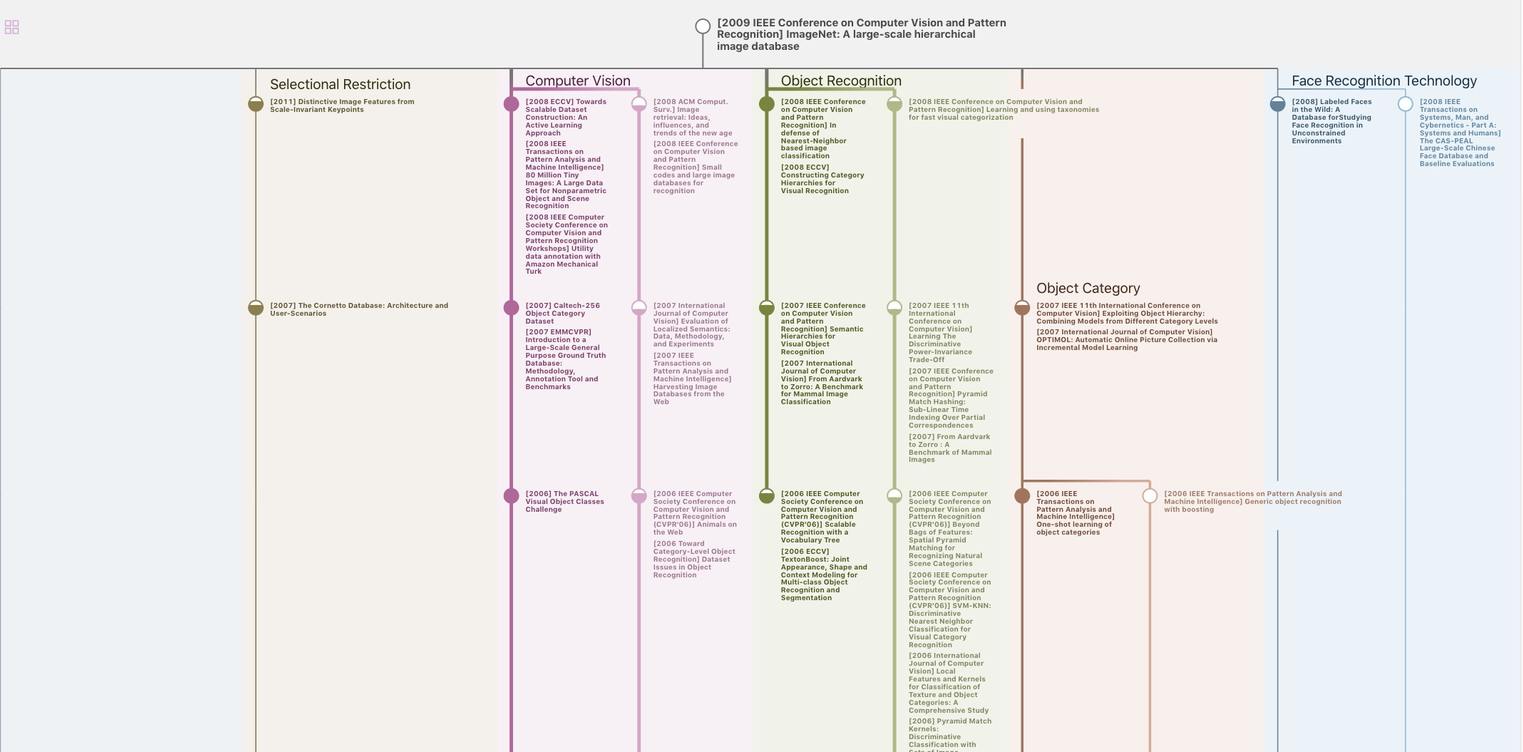
生成溯源树,研究论文发展脉络
Chat Paper
正在生成论文摘要