Learning embeddings for multiplex networks using triplet loss
Applied Network Science(2019)
摘要
Learning low-dimensional representations of graphs has facilitated the use of traditional machine learning techniques to solving classic network analysis tasks such as link prediction, node classification, community detection, etc. However, to date, the vast majority of these learning tasks are focused on traditional single-layer/unimodal networks and largely ignore the case of multiplex networks. A multiplex network is a suitable structure to model multi-dimensional real-world complex systems. It consists of multiple layers where each layer represents a different relationship among the network nodes. In this work, we propose MUNEM, a novel approach for learning a low-dimensional representation of a multiplex network using a triplet loss objective function. In our approach, we preserve the global structure of each layer, while at the same time fusing knowledge among different layers during the learning process. We evaluate the effectiveness of our proposed method by testing and comparing on real-world multiplex networks from different domains, such as collaboration network, protein-protein interaction network, online social network. Finally, in order to deliberately examine the effect of our model’s parameters we conduct extensive experiments on synthetic multiplex networks.
更多查看译文
关键词
Multiplex network, Network embedding, Triplet loss
AI 理解论文
溯源树
样例
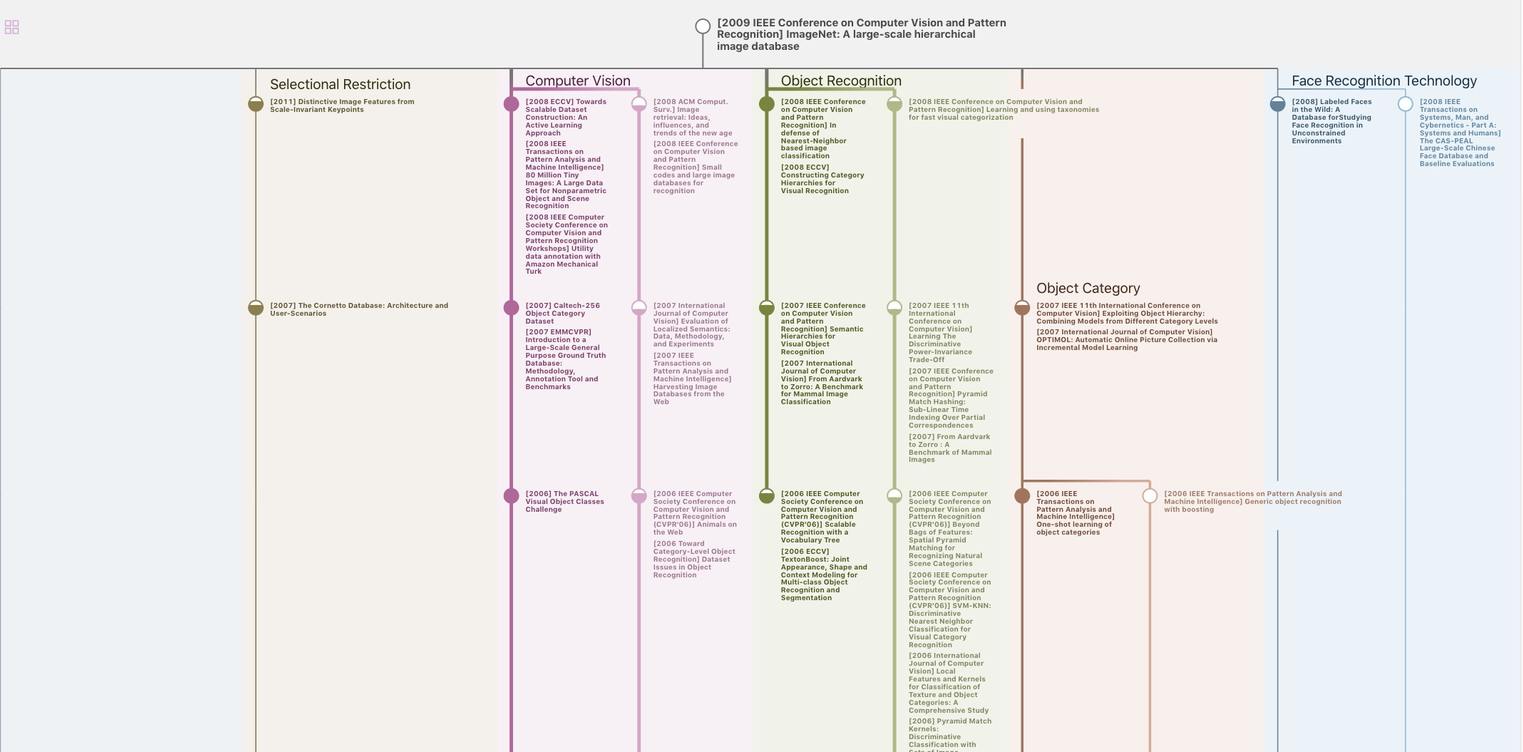
生成溯源树,研究论文发展脉络
Chat Paper
正在生成论文摘要