Modeling user exposure with recommendation influence
SAC '20: The 35th ACM/SIGAPP Symposium on Applied Computing Brno Czech Republic March, 2020(2020)
摘要
Recommender systems learn users' preferences from their explicit feedback, such as ratings, and implicit feedback, such as purchases. Learning a user's preference from implicit feedback is difficult since inaction in such data can be because of either dislike for an item or unawareness. Exposure modeling has been proposed recently to address this ambiguity by decomposing inaction into dislike and unawareness. A user's awareness of items can be affected by several factors, and exposure modeling has been extended to include social and temporal influences on exposure to provide better recommendations. However, recommendation itself should also affect a user's awareness of items. Recommendations boost awareness differently for different users; while some users are attentive to and trust recommendations, others neglect or distrust recommendations. Furthermore, if a user purchases an item from a recommendation list, the user would have likely noticed other items on the same list. In this paper, we extend exposure modeling by considering the influence of recommendations on exposure. We accomplish this by incorporating: (1) user-dependent increase in awareness of recommended items and (2) indirect increase in awareness of an item by the purchase of other items from the same recommendation list. Through experiments using public datasets with recommendation logs, we demonstrate that considering recommendation influence improves purchase prediction.
更多查看译文
关键词
Collaborative Filtering, Missing Not At Random, Treatment Effect
AI 理解论文
溯源树
样例
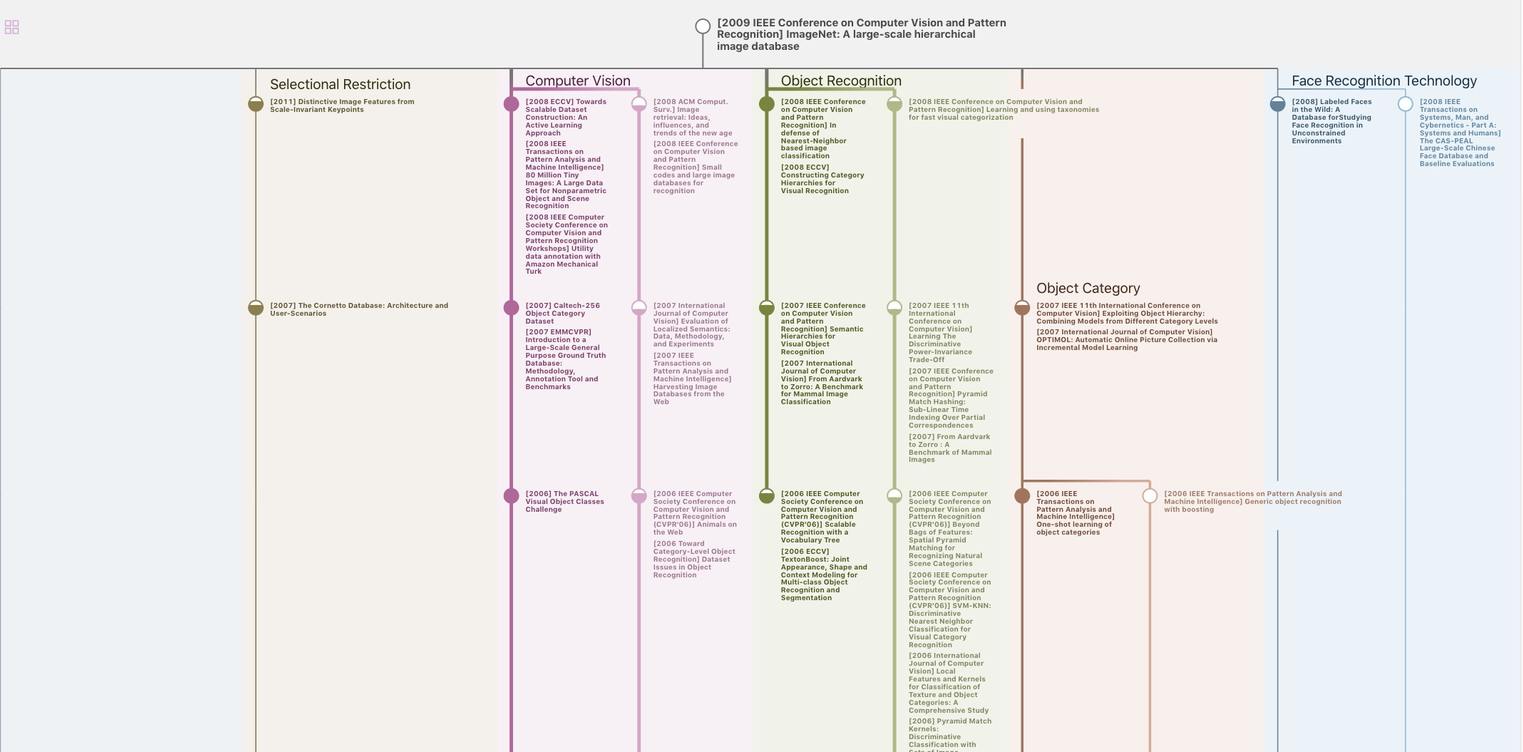
生成溯源树,研究论文发展脉络
Chat Paper
正在生成论文摘要