Adverse Drug Reaction Discovery from Electronic Health Records with Deep Neural Networks.
ACM CHIL '20: ACM Conference on Health, Inference, and Learning Toronto Ontario Canada April, 2020(2020)
摘要
Adverse drug reactions (ADRs) are detrimental and unexpected clinical incidents caused by drug intake. The increasing availability of massive quantities of longitudinal event data such as electronic health records (EHRs) has redefined ADR discovery as a big data analytics problem, where data-hungry deep neural networks are especially suitable because of the abundance of the data. To this end, we introduce neural self-controlled case series (NSCCS), a deep learning framework for ADR discovery from EHRs. NSCCS rigorously follows a self-controlled case series design to adjust implicitly and efficiently for individual heterogeneity. In this way, NSCCS is robust to time-invariant confounding issues and thus more capable of identifying associations that reflect the underlying mechanism between various types of drugs and adverse conditions. We apply NSCCS to a large-scale, real-world EHR dataset and empirically demonstrate its superior performance with comprehensive experiments on a benchmark ADR discovery task.
更多查看译文
关键词
Adverse Drug Reaction Discovery,Deep Neural Networks,Electronic Health Records,Self-Controlled Case Series
AI 理解论文
溯源树
样例
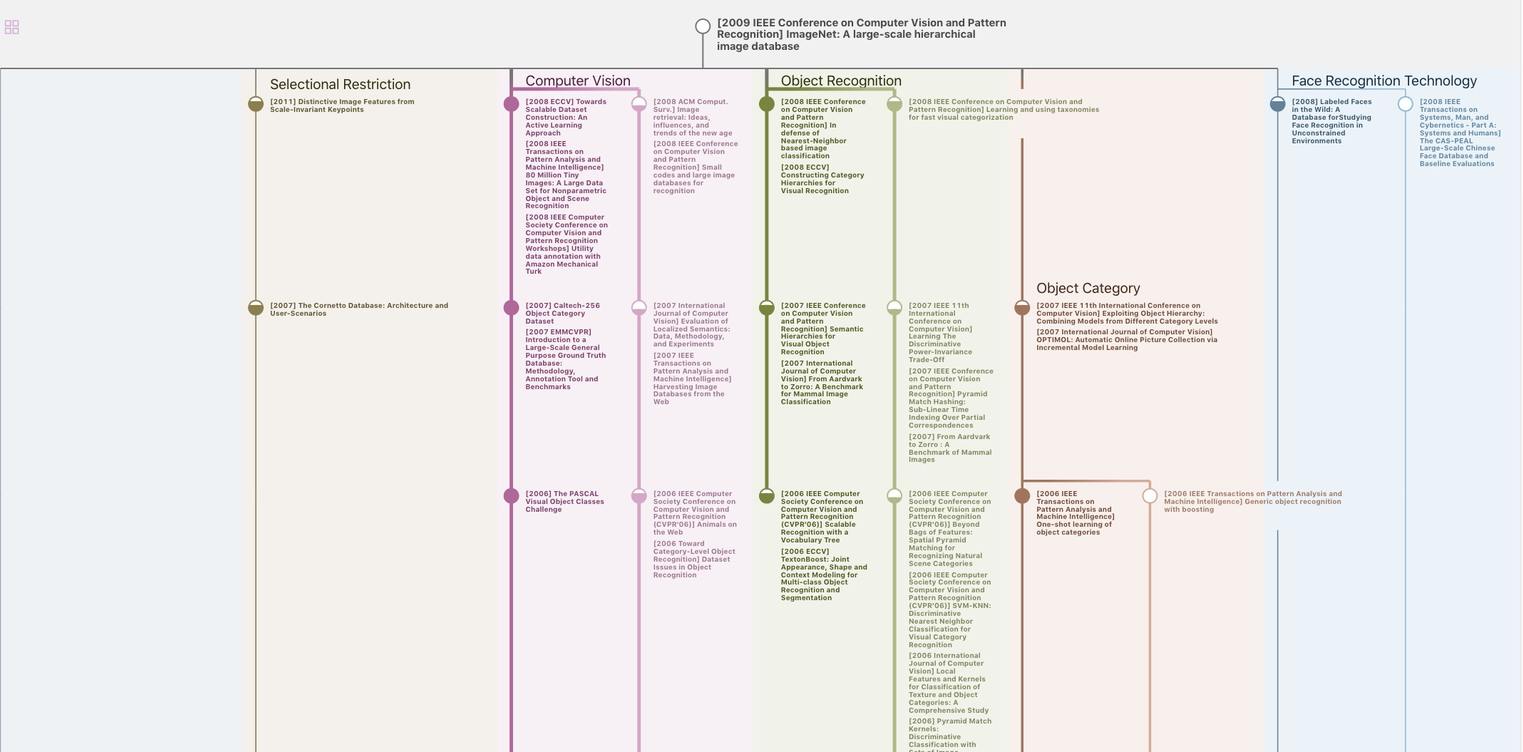
生成溯源树,研究论文发展脉络
Chat Paper
正在生成论文摘要