3:36 PM Abstract No. 36 Machine learning-based radiomic features on pre-ablation magnetic resonance imaging as predictors of pathologic response in patients with hepatocellular carcinoma listed for hepatic transplant
Journal of Vascular and Interventional Radiology(2020)
摘要
To assess the role of pretreatment tumor radiomic features in predicting pathologic response in patients with hepatocellular carcinoma (HCC) listed for liver transplant. In this IRB-approved retrospective study, we enrolled the first 40 consecutive patients from an institutional ablation database that met the following inclusion criteria: a) Age>18 years; b) being listed for liver transplant; b) undergoing an RFA or microwave ablation between 2005 and 2015; and c) having a pre-ablation contrast-enhanced MRI within 3 months prior to ablation. Pathologic response was assessed at the time of transplant. The treated hepatic lesion was identified on the arterial phase T1 postcontrast MRI images and the tumor volume was manually segmented. A vector consisting of 110 radiomic features (shape, first-order, and texture) was extracted from each tumor. Univariate logistic regression analysis was used to assess the contribution of each of the extracted texture features to outcome prediction. A linear support vector machine (SVM) machine learning technique was applied to the extracted feature vector. The dataset was divided into a training and testing set at a ratio of 4:1. The classifiers were trained and tested using leave-one-out cross validation to avoid overfitting. ROC analysis was used to assess classification performance. 40 patients with 55 HCC tumors were enrolled (Age 54.9 ± 8.9; 78% male). The mean tumor size was 2.2 ± 0.66 cm. The mean MELD score was 10 ± 3.5. Mean follow-up time postablation was 348.6 ± 179 days. 29% of patients did not exhibit complete pathologic response on pathology review at the time of transplant. A total of 10 first-order features, 2 GLDM features, 5 GLRM features, 8 GLSZM and 4 shape features were associated with incomplete pathologic response on univariate logistic regression analysis (P <0.05). An SVM-based machine learning model that included the assessed features was predictive of incomplete pathologic response with an AUC of 0.71. Machine learning-based radiomic analysis of pre-ablation MRI may aid in the prediction of tumor pathologic response in patients with HCC undergoing liver transplantation.
更多查看译文
关键词
hepatocellular carcinoma,hepatic transplant,magnetic resonance imaging,radiomic features,learning-based,pre-ablation
AI 理解论文
溯源树
样例
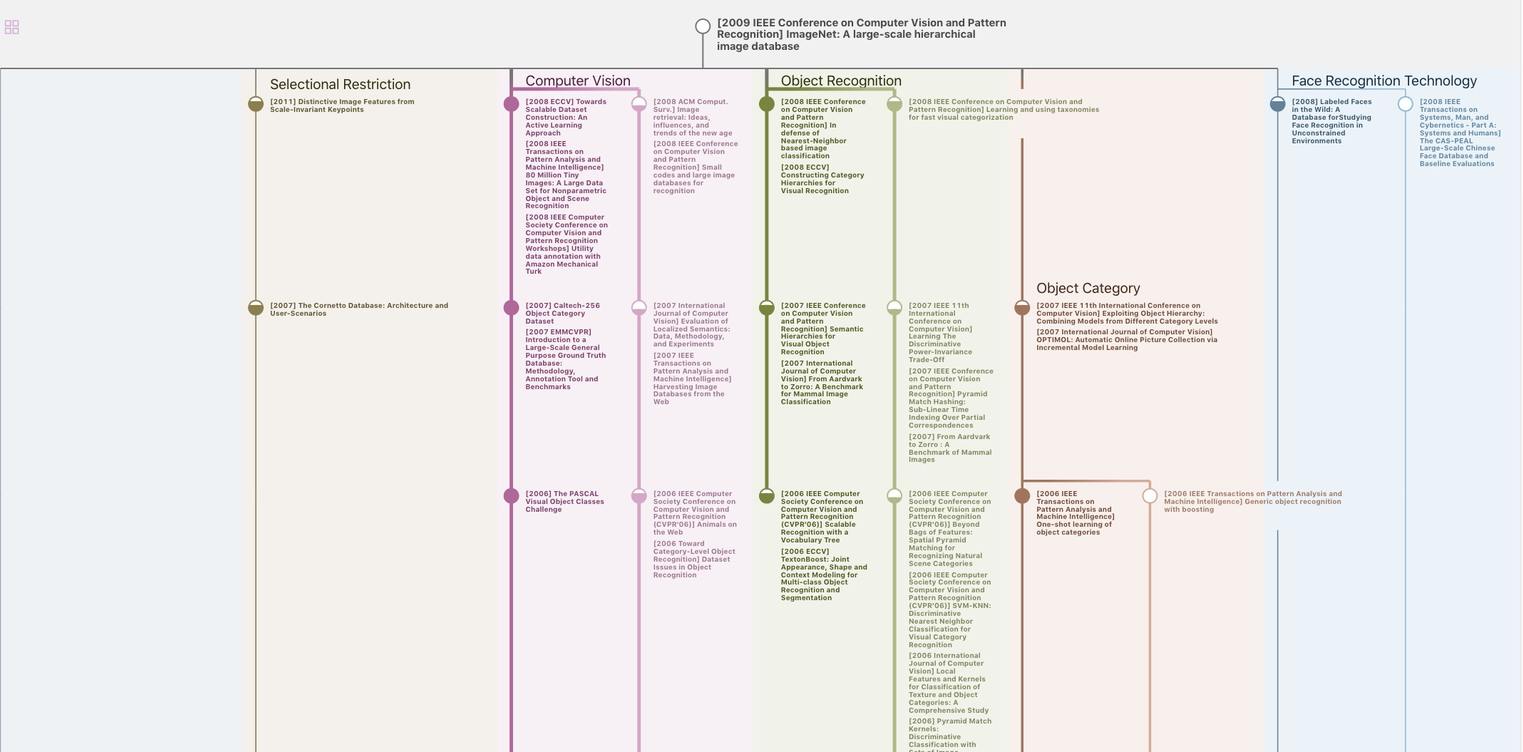
生成溯源树,研究论文发展脉络
Chat Paper
正在生成论文摘要