Deep Semantic Reconstruction Hashing for Similarity Retrieval
Periodicals(2021)
摘要
AbstractHashing has shown enormous potentials in preserving semantic similarity for large-scale data retrieval. Existing methods widely retain the similarity within two binary codes towards their discrete semantic affinity, i.e., 1 or −1. However, such a discrete reconstruction approach has obvious drawbacks. First, two unrelated dissimilar samples would have similar binary codes when both of them are the most dissimilar with an anchor sample. Second, the fine-grained semantic similarity cannot be shown in the generated binary codes among data with multiple semantic concepts. Furthermore, existing approaches generally adopt a point-wise error-minimizing strategy to enforce the real-valued codes close to its associated discrete codes, resulting in the well-learned paired semantic similarity being unintentionally damaged when performing quantization. To address these issues, we propose a novel deep hashing method with pairwise similarity-preserving quantization constraint, termed Deep Semantic Reconstruction Hashing (DSRH), which defines a high-level semantic affinity within each data pair to learn compact binary codes. Specifically, DSRH is expected to learn the specific binary codes whose similarity can reconstruct their high-level semantic similarity. Besides, we adopt a pairwise similarity-preserving quantization constraint instead of the traditional point-wise quantization technique, which is conducive to maintain the well-learned paired semantic similarity when performing quantization. Extensive experiments are conducted on four representative image retrieval benchmarks, and the proposed DSRH outperforms the state-of-the-art deep-learning methods with respect to different evaluation metrics.
更多查看译文
关键词
Semantics, Quantization (signal), Binary codes, Image reconstruction, Hamming distance, Marine vehicles, Airplanes, Deep hashing, high-level semantic similarity, similarity-preserving quantization, similarity retrieval
AI 理解论文
溯源树
样例
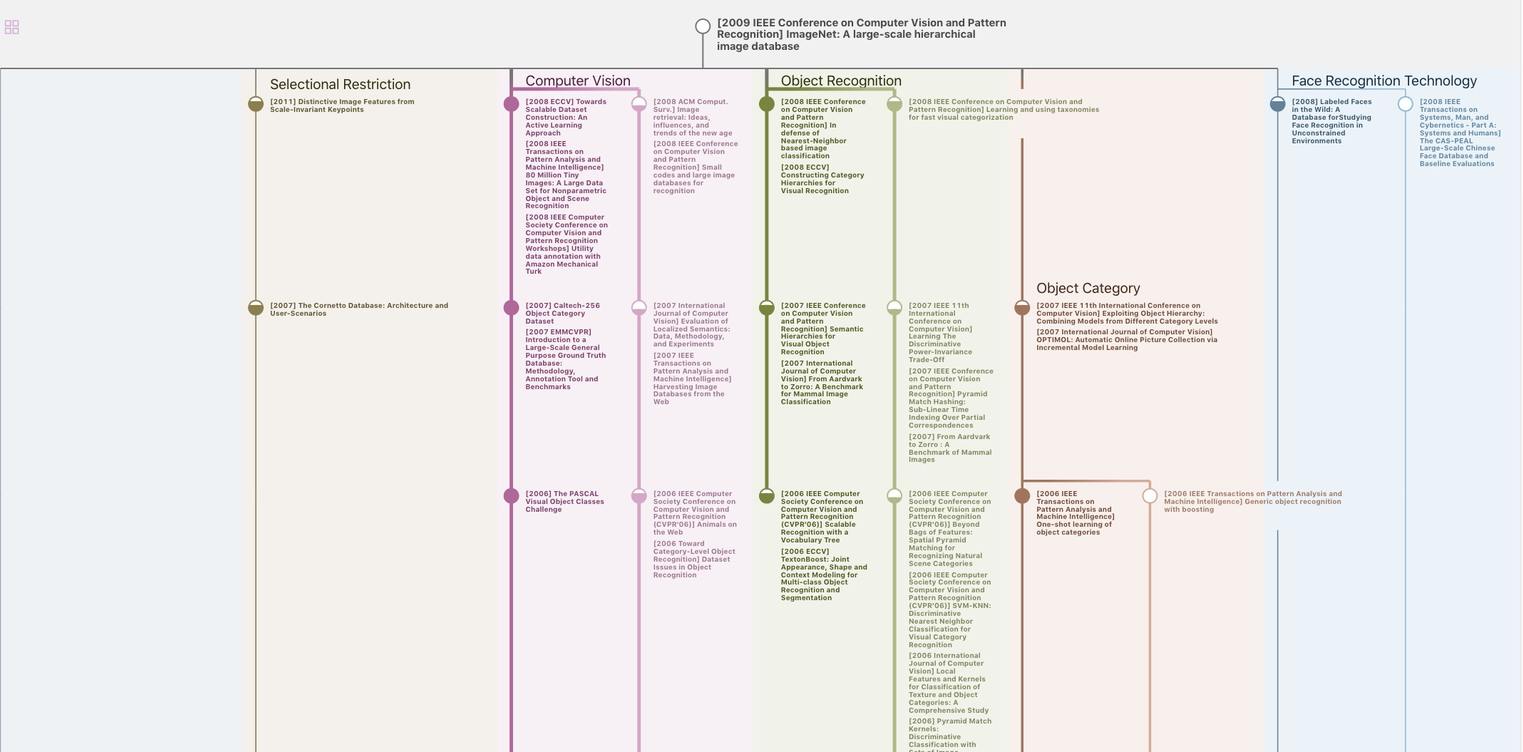
生成溯源树,研究论文发展脉络
Chat Paper
正在生成论文摘要