Using A Low Correlation High Orthogonality Feature Set And Machine Learning Methods To Identify Plant Pentatricopeptide Repeat Coding Gene/Protein
NEUROCOMPUTING(2021)
摘要
Motivation: Identifying whether a pentatricopeptide repeat (PPR) exists in an amino acid is a significant task in the field of bioinformatics. To address this problem, an identification method that combines an optimal feature set selection framework and machine learning algorithms is proposed to recognize the PPR coding genes and proteins in the sequence of amino acid. The original 188-dimensional (D) features are obtained using a feature extraction method, which is successively optimised through a covariance analysis, max-relevant-max-distance processing, and principal component analysis to reduce it to an optimal feature set that has fewer but more expressive features. Four machine learning methods are then used to serve as the classifiers for the identification task.Results: The final number of feature data dimensions is reduced from 188 to only 10, and according to the experimental results from support vector machine methods, the loss of the AUC and the F-1 values are only 3.26% and 10.1%, respectively. Moreover, after applying the J48, random forest, and naive Bayes methods as classifiers, it was also found that the optimal feature set with 10 dimensions has an almost equivalent performance for a 10-fold validation test. (c) 2020 Elsevier B.V. All rights reserved.
更多查看译文
关键词
Pentatricopeptide repeat, Amino acid, Correlation analysis, Max-relevance-max-distance, Principal component analysis, T test
AI 理解论文
溯源树
样例
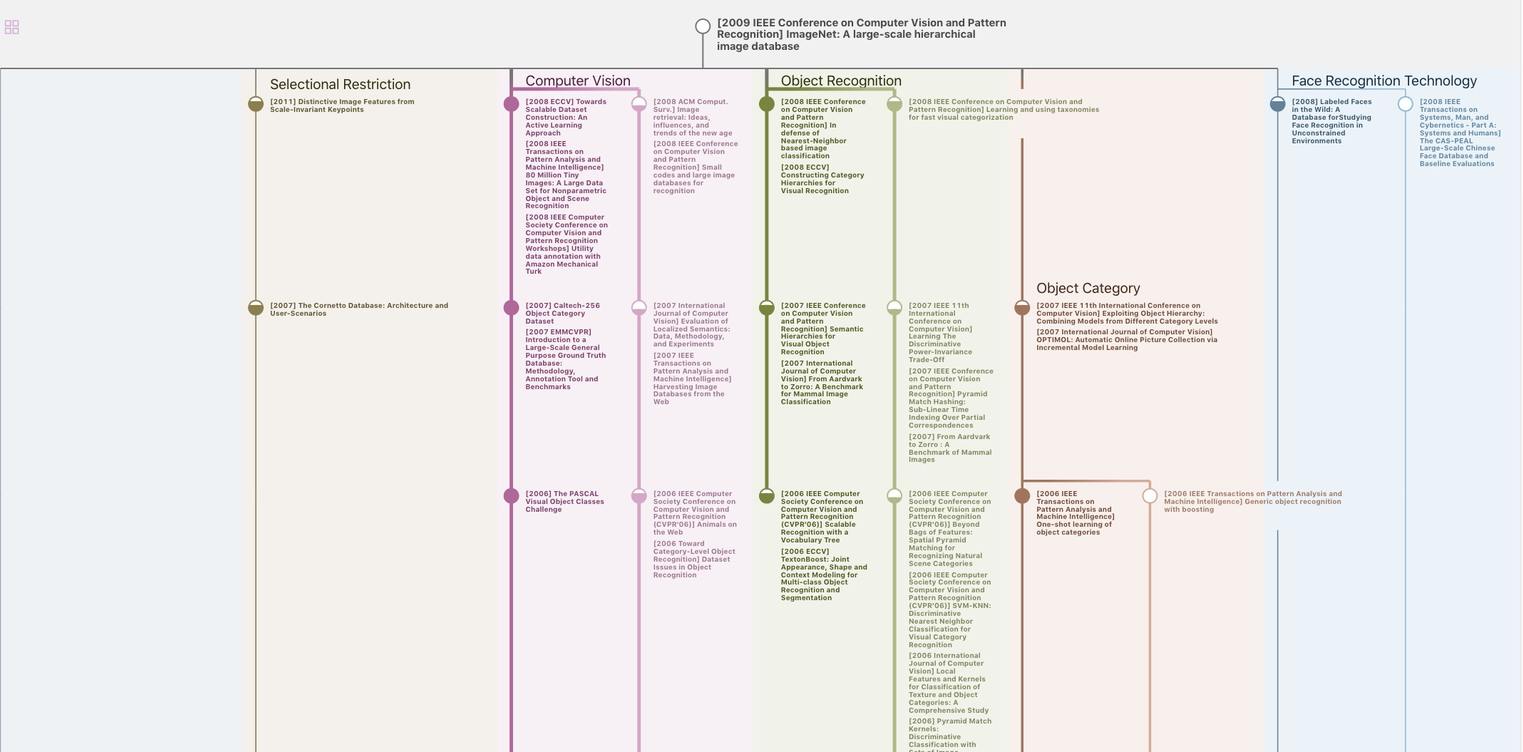
生成溯源树,研究论文发展脉络
Chat Paper
正在生成论文摘要