Siamese Deformable Cross-Correlation Network for Real-Time Visual Tracking
Neurocomputing(2020)
摘要
In recent years, SiamFC-based trackers have received much attention because of their great potentials in balancing tracking accuracy and speed. However, the robustness of most such trackers is greatly affected by the large deformations of targets. We argue that in the cross-correlation operation which is widely used by modern SiamFC-based trackers, the static correlation between the template kernel and the feature maps of test sample is difficult to adapt to the large deformation of the target object. In this paper, we propose a Siamese deformable cross-correlation network (SiamDCN), which introduces the deformable cross-correlation operation into SiamFC in an online self-adaptive way, for robust visual tracking. Compared to the previous SiamFC-based trackers, our SiamDCN is more robust to the large deformations of targets through dynamically and adaptively adjusting the location of correlation calculation for each element of the template kernel in the cross-correlation operation. Moreover, we build a twofold Siamese network, named SiamDCN+, which consists of a SiamDCN branch and a SiamFC branch, for accurate and real-time visual tracking after observing that the features learned in SiamFC are static and discriminative, whereas those in SiamDCN are dynamic and robust, and they complement each other. Extensive experiments on three public benchmarks, OTB2015, VOT2016, and VOT2017, show that the proposed SiamDCN achieves superior localization accuracy than its baseline tracker SiamFC and the proposed SiamDCN+ achieves competitive performance compared to state-of-the-art real-time trackers, while running beyond 40 FPS.
更多查看译文
关键词
Visual Tracking,Convolutional Neural Networks,Siamese network,Deformable Convolutional Network
AI 理解论文
溯源树
样例
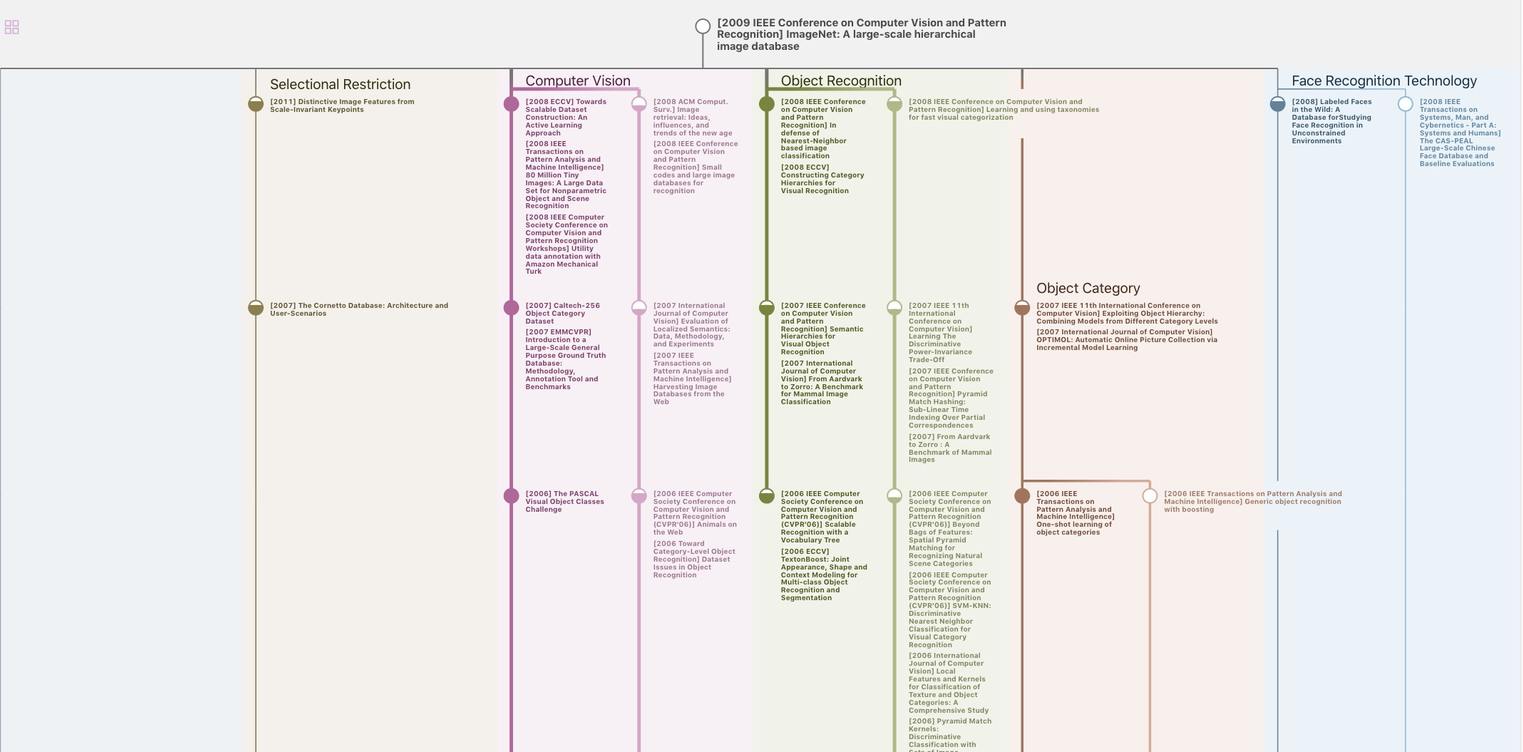
生成溯源树,研究论文发展脉络
Chat Paper
正在生成论文摘要