Effective inertial sensor quantity and locations on a body for deep learning-based worker's motion recognition
Automation in Construction(2020)
摘要
Construction tasks involve various activities composed of one or more body motions. As construction projects are labor-intensive and heavily rely on manual tasks, understanding the ever-changing behavior and activities is essential to manage construction workers effectively regarding their safety and productivity. While several research efforts have shown promising results in automated motion and activity recognition of the workers using motion sensors, there is still a lack of understanding about how motion sensors' numbers and their locations affect the performance of the recognition, which can contribute to improving the recognition performance and reducing the implementation cost. Moreover, further research is necessary to seek the motion recognition model that accurately identifies various motions using motion sensors attached to the workers' bodies. This study proposes a construction worker's motion recognition model using the Long Short-Term Memory (LSTM) network based on an evaluation of the effectiveness of motion sensors' numbers and locations to maximize motion recognition performance. The evaluation is conducted by generating different datasets containing motion sensor data collected from the sensors located on different body parts. Comparing the performance of five machine learning models trained using the datasets, the desired numbers and locations of motion sensors are identified. The quasi-experimental test with multiple subjects is conducted to validate the findings of the evaluation. Based on the findings, the LSTM network for recognizing construction workers' motions is developed. The LSTM network classifies various motions of the workers that can be utilized as primitive elements for monitoring the workers regarding their safety and productivity.
更多查看译文
关键词
Construction worker,Motion recognition,Monitoring,Deep learning,Long short-term memory
AI 理解论文
溯源树
样例
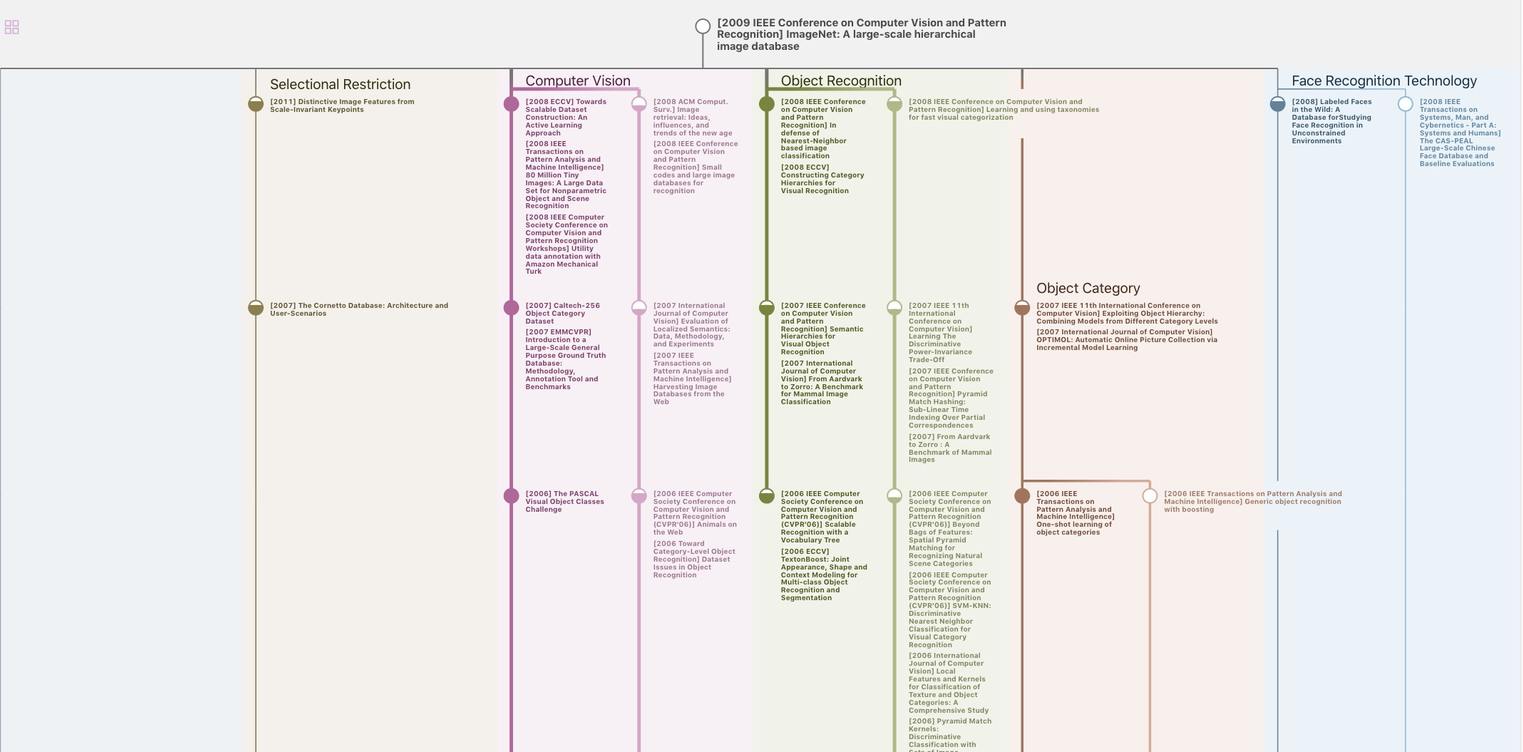
生成溯源树,研究论文发展脉络
Chat Paper
正在生成论文摘要