Classification of Exogenous Anomalies and Self-Diagnosis in Autonomous Robots
2019 IEEE CHILEAN Conference on Electrical, Electronics Engineering, Information and Communication Technologies (CHILECON)(2019)
摘要
This paper presents methods for the autonomous identification and classification of disturbances that have negative effects on a robot's performance. The proposed methods have been implemented in a walking hexapod robot provided with a number of sensors. Both, the robot's sensorial information and a quantitative measure of the robot's performance are obtained. This information is used for detecting, identifying and classifying obstructive conditions that have a strong impact on the robot's performance. Once the cause of a lack of progress in the robot's mission has been identified, suitable compensatory actions are found, executed and recorded. Then, when previously experienced detrimental situations arise, the associated compensatory measures are immediately taken without involving a searching process. As a result, the recovery from abnormal conditions is accelerated and the robot can promptly continue with its mission. In order to evaluate the performance of the proposed methods, a number of different sets of experiments addressing the robot's hardware faults, abnormal situations generated in the robot's environment and a combination of both, were conducted. In this process, two indicators were utilized: the number of attempts before a correct identification of the robot's hardware fault was achieved, and a discrepancy measure. Results showed a good identification rate inside the range of considered abnormal situations.
更多查看译文
关键词
Self-diagnosis,Fault-detection,Multi-sensor data classification,Biologically inspired walking robots
AI 理解论文
溯源树
样例
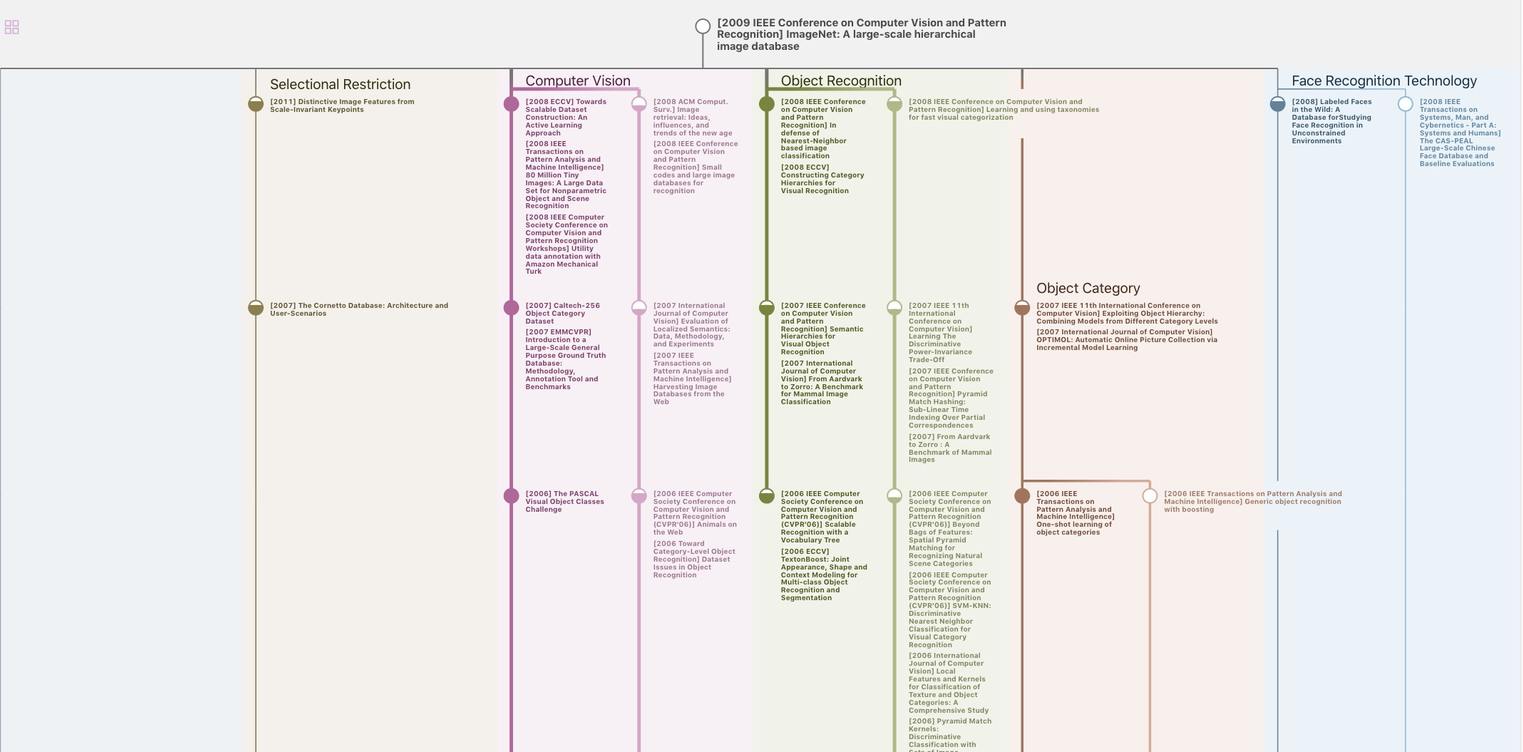
生成溯源树,研究论文发展脉络
Chat Paper
正在生成论文摘要