DRM-SLAM: Towards Dense Reconstruction of Monocular SLAM with Scene Depth Fusion
Neurocomputing(2020)
摘要
Monocular visual SLAM methods can accurately track the camera pose and infer the scene structure by building sparse correspondence between two/multiple views of the scene. However, the reconstructed 3D maps of these methods are extremely sparse. On the other hand, deep learning is widely used to predict dense depth maps from single-view color images, but the results are subject to blurry depth boundaries, which severely deform the structure of 3D scene. Therefore, this paper proposes a dense reconstruction method under the monocular SLAM framework (DRM-SLAM), in which a novel scene depth fusion scheme is designed to fully utilize both the sparse depth samples from monocular SLAM and predicted dense depth maps from convolutional neural network (CNN). In the scheme, a CNN architecture is carefully designed for robust depth estimation. Besides, our approach also accounts for the problem of scale ambiguity existing in the monocular SLAM. Extensive experiments on benchmark datasets and our captured dataset demonstrate the accuracy and robustness of the proposed DRM-SLAM. The evaluation of runtime and adaptability under challenging environments also verify the practicability of our method.
更多查看译文
关键词
Depth fusion,SLAM,Monocular,Depth estimation
AI 理解论文
溯源树
样例
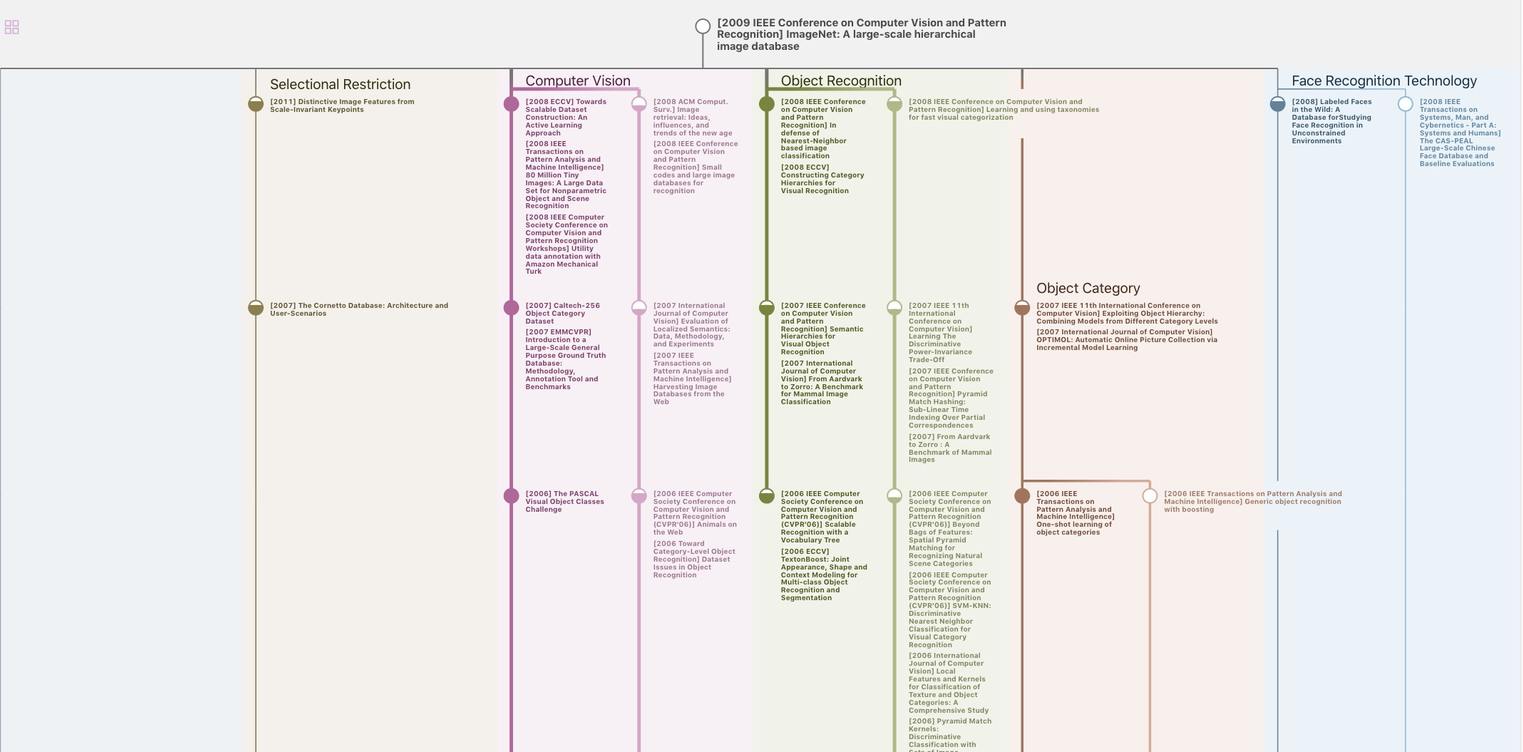
生成溯源树,研究论文发展脉络
Chat Paper
正在生成论文摘要