Improved Flooding Molecular Dynamics Analysis
BIOPHYSICAL JOURNAL(2020)
摘要
Many proteins, regardless of their function, can be regulated by a myriad of small molecules. Knowing the details of these interactions in molecular scale is a key point to understand the mechanism of biological processes. However, protein modulation by small ligands have complex features: there may exist multiple binding sites, with different occupancy states, many ligand configurations and varying affinities. A good description of these phenomena can be obtained by flooding Molecular Dynamics (flooding-MD), which is a simulation with a complete protein system added a fixed number of ligands. Usually, the main information obtained are high-probability ligand regions nearby the receptor. However, truthful results require long computational timescales due to convergence of a huge number of states. Given the effort put into these simulations, here we present an optimized framework to analyze flooding-MD simulations, capable of reconstructing ligand binding constants, which will be able to describe several interaction properties in any desired concentration - occupancy probabilities, spatial probabilities, mean bound ligand numbers and site affinities - without the need to run new simulations. We illustrate the use of the framework for the mammalian voltage-gated potassium channel Kv1.2, and sevoflurane, a positive modulator. For each concentration ranging from 50mM to 150mM sevoflurane, >200ns flooding-MD simulations were performed. From the highest concentrated simulation (150mM), for each site, the theory was applied to estimate the binding constants and other properties at more diluted concentrations. In order to verify accuracy of these projections, they were directly compared to more diluted flooding-MD simulations (<150mM sevoflurane) properties and had satisfying results, within an error margin. This improved way of analyzing flooding-MDs can be applied to diverse protein-ligand interactions, offering a simpler and faster way to study these interactions, without the need of additional simulations.
更多查看译文
关键词
molecular,dynamics
AI 理解论文
溯源树
样例
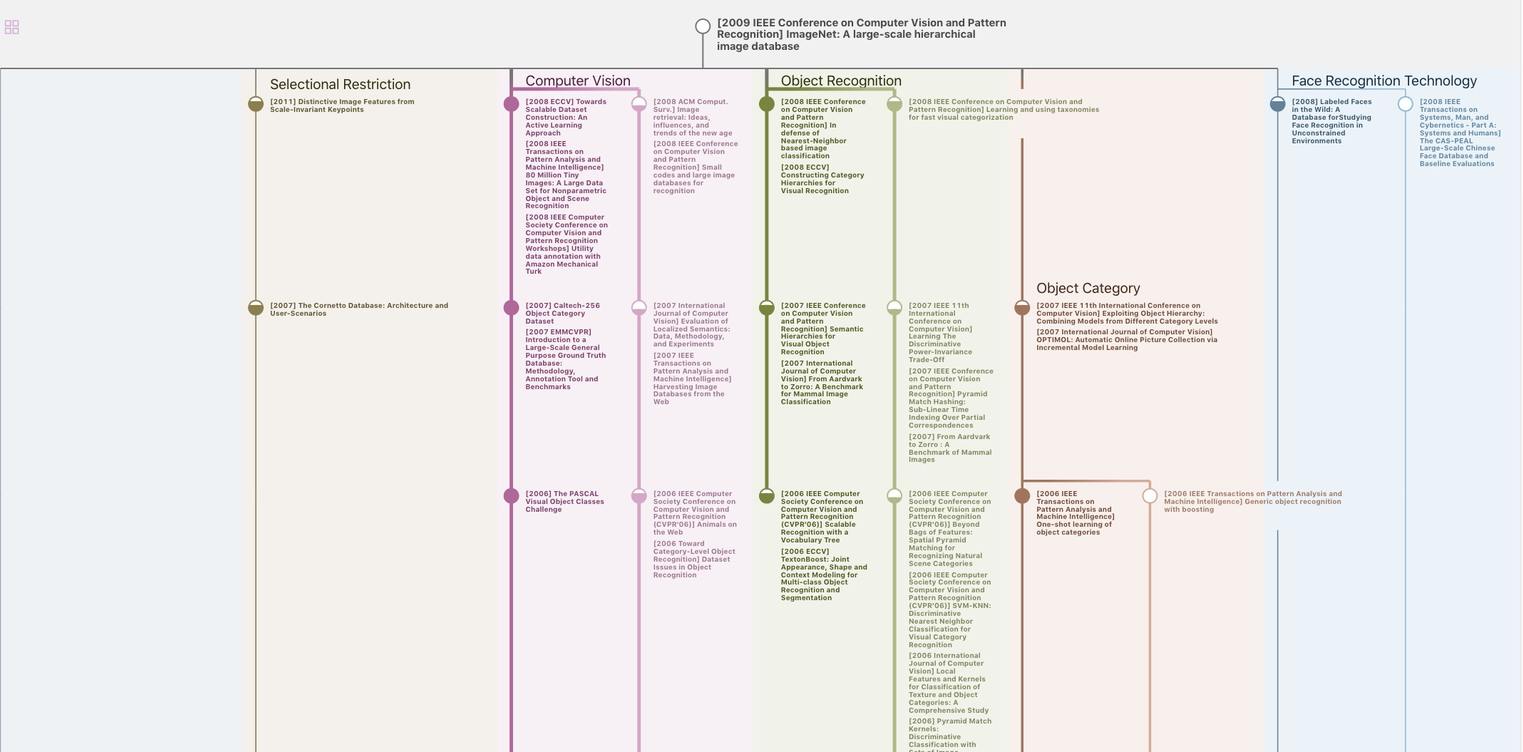
生成溯源树,研究论文发展脉络
Chat Paper
正在生成论文摘要