Vehicular Task Offloading via Heat-Aware MEC Cooperation Using Game-Theoretic Method
IEEE Internet of Things Journal(2020)
摘要
Mobile-edge computing (MEC) has been witnessed as a promising solution for the vehicular task offloading. Due to the limited computing resource of individual MEC servers, it faces challenges when higher requirements are put forward for timely task processing of a large amount of computations in the emerging vehicular applications. In this article, we strive to realize the efficient vehicular task offloading via heat-aware MEC cooperation from the game theory perspective. Here, the heat indicates the vehicle density and is tightly related to the requests of vehicle users when they drive through the hot zones. Specifically, a deep learning-based prediction method is proposed, capturing the dynamic time-varying heat value of the hot zones based on the analysis of the real-world private car trajectory data. To identify the role of MEC in the cooperation, we take the time-delay constraint into consideration for the task offloading. To realize MEC grouping for task offloading in MEC cooperation, we formulate the MEC grouping as a utility maximization problem via designing a noncooperative game-theoretic strategy selection based on regret-matching. Furthermore, we derive the correlated equilibrium and prove that the fast convergence can be achieved. Extensive simulation results validate the effectiveness of the proposed vehicular task offloading approach under various system parameters, such as computation workload, time slots, and MEC servers number. The proposed method outperforms the existing methods, which is able to significantly reduce the task complete delay, and in the meantime enhance the MEC energy efficiency with end users’ quality-of-experience guaranteed.
更多查看译文
关键词
Correlated equilibrium,mobile-edge computing (MEC),private cars,vehicular task offloading
AI 理解论文
溯源树
样例
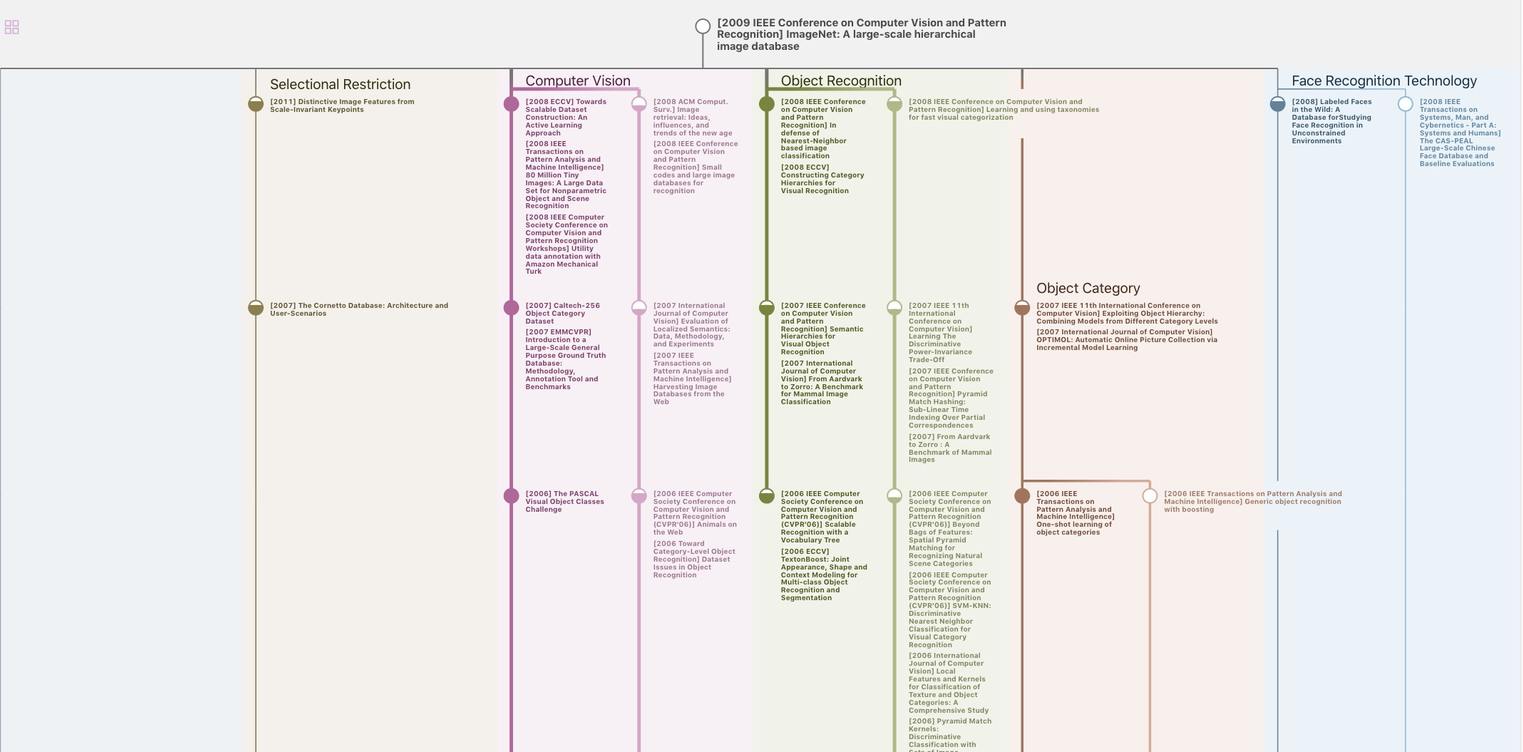
生成溯源树,研究论文发展脉络
Chat Paper
正在生成论文摘要