PBEM: A Pattern-Based Embedding Model for User Location Category Prediction
2019 Twelfth International Conference on Mobile Computing and Ubiquitous Network (ICMU)(2019)
摘要
With the rapid popularity of mobile devices, a vast amount of trajectory-based check-in data are shared in many social network applications, which is an important data source for user location prediction. The location category prediction, a branch of location prediction, is a vital task in a wide range of areas, including urban planning, advertising and recommendation systems. In this paper, we propose a novel two-step Pattern-Based Embedding Model (PBEM) for predicting the next location category that user will go to. Based on the observation that some users behave frequently in a similarity pattern, a new feature termed as user cluster label is defined. In order to mine user's behavior patterns and extract the cluster label, a Category-Importance-Decay learning strategy is proposed and implemented, which provides a quantitative standard for evaluating the importance of each category. Thus, a comprehensive feature set is obtained including user, time, historical location category, text content, and user cluster label, which greatly enhances the robustness of data representation and contains more knowledge. Then the extracted feature set is fed into Recurrent Neural Network (RNN) in a unified framework, which improves the prediction accuracy. We evaluate the performance of PBEM on two real-life trajectory-based check-in datasets. Experimental results demonstrate that the proposed model can outperform the state-of-the-art methods.
更多查看译文
关键词
location category prediction,recurrent neural network,sequence pattern mining,social network,cluster
AI 理解论文
溯源树
样例
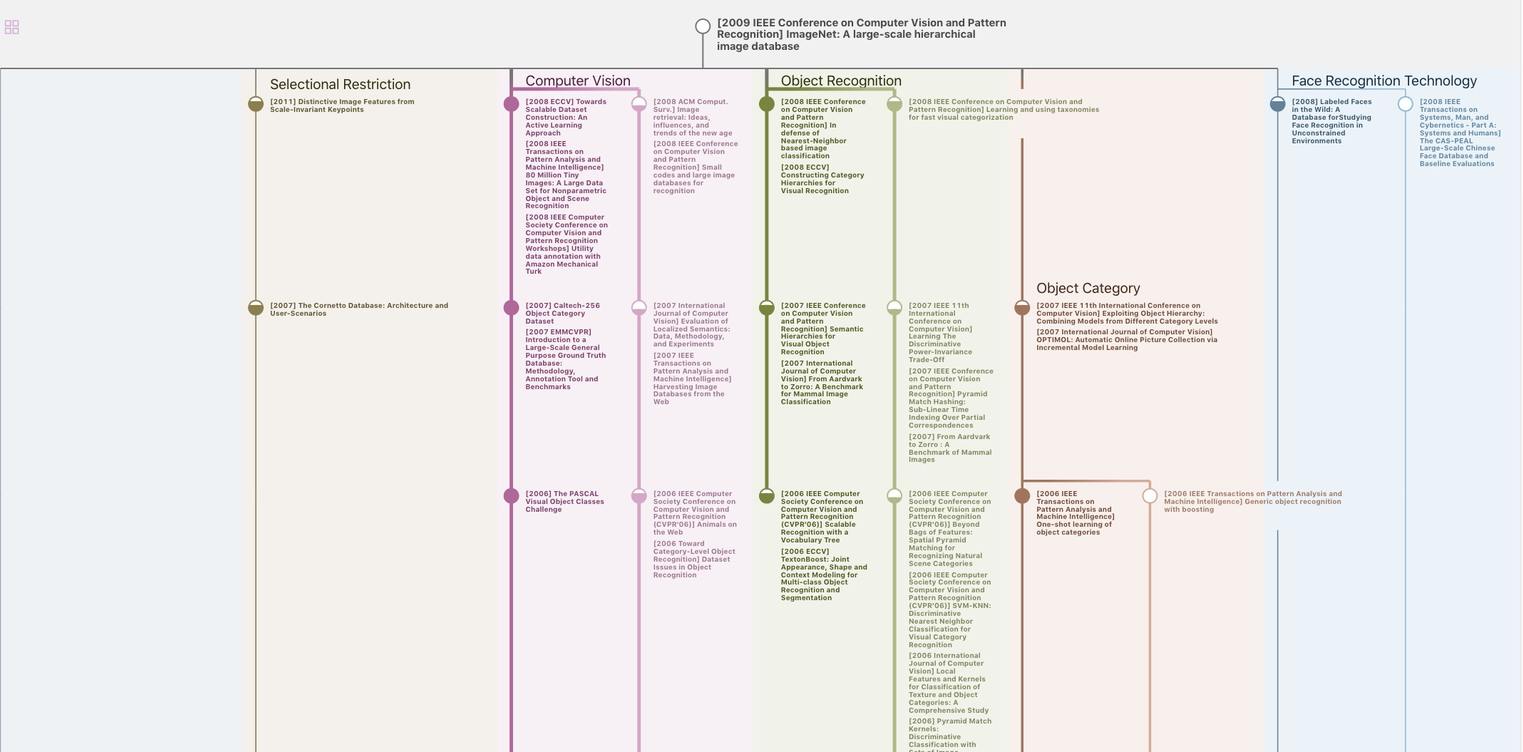
生成溯源树,研究论文发展脉络
Chat Paper
正在生成论文摘要