Semi-supervised Trajectory Understanding with POI Attention for End-to-End Trip Recommendation.
ACM Transactions on Spatial Algorithms and Systems(2020)
摘要
Trip planning/recommendation is an important task for a plethora of applications in urban settings (e.g., tourism, transportation, social outings), relying on services provided by Location-Based Social Networks (LBSN). To provide greater context-awareness in trajectory planning, LBSNs combine historical trajectories of users for generating various hand-crafted features—e.g., geo-tags of photos taken by tourists and textual characteristics derived from reviews. Those features are used to learn tourists’ preferences, which are then used to generate a travel plan recommendation. However, many such features are extracted based on prior knowledge or empirical analysis specific to particular datasets, rendering the corresponding solutions not to be generalizable to diverse data sources. Thus, one important question for managing mobility is how to learn an accurate tour planning model based solely on POI visits or user check-ins and without the efforts of hand-crafted feature engineering. Inspired by recent successes of deep learning in sequence learning, we develop a solution to the tour planning problem based on the semi-supervised learning paradigm. An important aspect of our solution is that it does not involve any feature engineering. Specifically, we propose the Trip Recommendation method via trajectory Encoder and Decoder—a novel end-to-end approach encoding historical trajectories into vectors, while capturing both the intrinsic characteristics of individual POIs and the transition patterns among POIs. We also incorporate historical attention mechanism in our sequence-to-sequence trip recommendation task to improve the effectiveness. Experiments conducted on multiple publicly available LBSN datasets demonstrate significantly superior performance of our method.
更多查看译文
关键词
Trip recommendation,attention mechanism,encoder-decoder,recurrent neural networks,semi-supervised learning
AI 理解论文
溯源树
样例
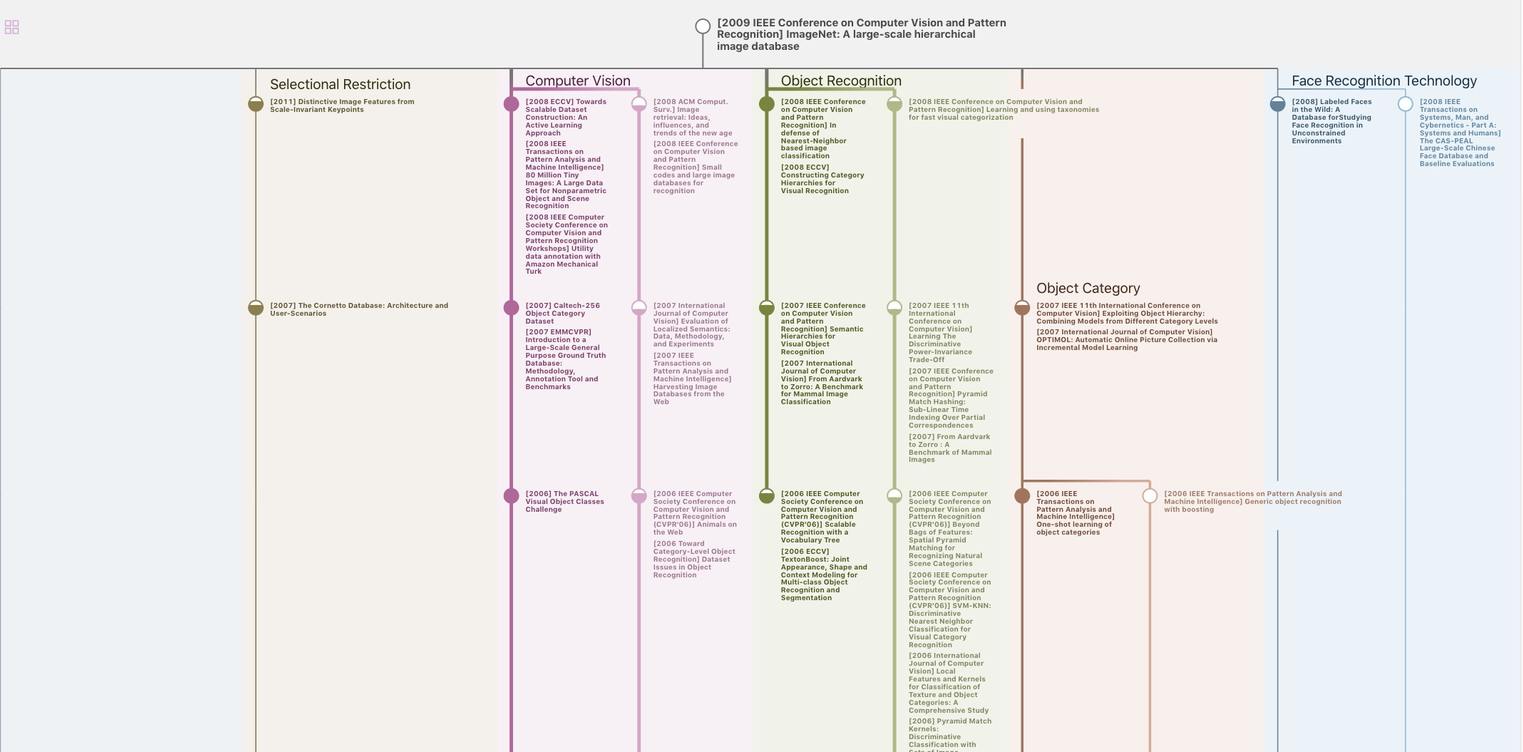
生成溯源树,研究论文发展脉络
Chat Paper
正在生成论文摘要