Optimising topic coherence with Weighted Po'lya Urn scheme.
Neurocomputing(2020)
摘要
Topic models have been widely used to mine hidden topics from documents. However, one limitation of such topic models is that they are prone to generate incoherent topics. To address this limitation, many approaches have been proposed to incorporate the prior knowledge of word semantic relatedness into the topic inference process. One example is the Generalized Po´lya Urn (GPU) scheme. However, GPU-based topic models often require sophisticated algorithms to acquire domain-specific knowledge from data. Moreover, prior knowledge is incorporated into the topic inference process without considering its impact on the intermediate topic sampling results. In this paper, we propose a novel Weighted Po´lya Urn scheme and incorporate it into Latent Dirichlet Allocation framework to build the self-enhancement topic model and generate coherent topics. In specific, semantic prior knowledge based on word embedding is employed to measure the semantic coherence of a word to different topics, which is incorporated into the Weighted Po´lya Urn scheme. Moreover, semantic coherence is updated dynamically based on the semantic similarity between a word and the representative words in different topics. Experiments have been conducted on seven public corpora from different domains to evaluate the effectiveness of the proposed approach. Experimental results show that compared to the state-of-the-art baselines, the proposed approach can generate more coherent topics.
更多查看译文
关键词
Po´lya urn scheme,Unsupervised learning,Topic model,Sentiment analysis
AI 理解论文
溯源树
样例
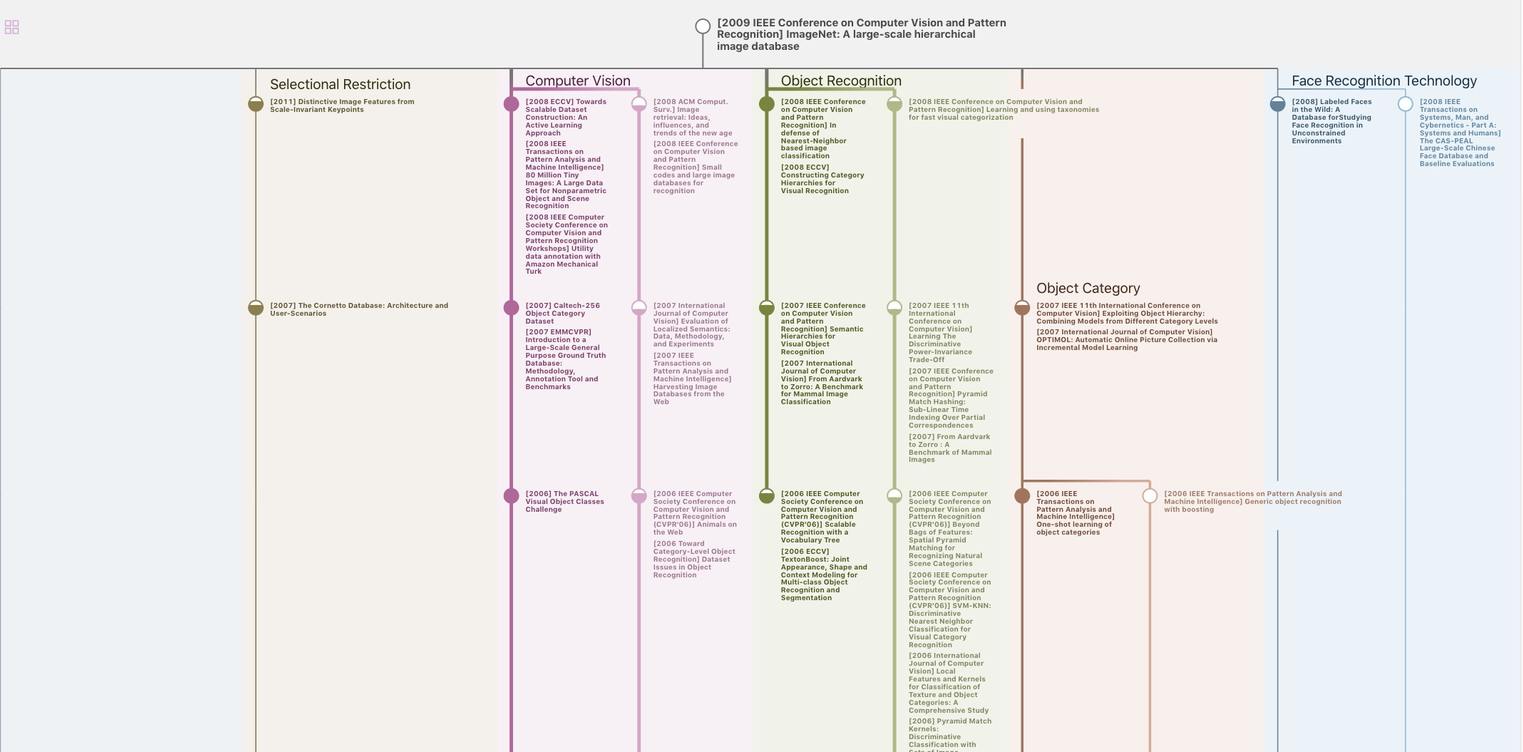
生成溯源树,研究论文发展脉络
Chat Paper
正在生成论文摘要