Efficient Discovery of Weighted Frequent Neighborhood Itemsets in Very Large Spatiotemporal databases
IEEE Access(2020)
摘要
Weighted Frequent Itemset (WFI) mining is an important model in data mining. It aims to discover all itemsets whose weighted sum in a transactional database is no less than the user-specified threshold value. Most previous works focused on finding WFIs in a transactional database and did not recognize the spatiotemporal characteristics of an item within the data. This paper proposes a more flexible model of Weighted Frequent Neighborhood Itemsets (WFNI) that may exist in a spatiotemporal database. The recommended patterns may be found very useful in many real-world applications. For instance, an WFNI generated from an air pollution database indicates a geographical region where people have been exposed to high levels of an air pollutant, say PM 2.5. The generated WFNIs do not satisfy the anti-monotonic property. Two new measures have been presented to effectively reduce the search space and the computational cost of finding the desired patterns. A pattern-growth algorithm, called Spatial Weighted Frequent Pattern-growth, has also been presented to find all WFNIs in a spatiotemporal database. Experimental results demonstrate that the proposed algorithm is efficient. We also describe a case study in which our model has been used to find useful information in air pollution database.
更多查看译文
关键词
Data mining,weighted frequent itemset,pattern-growth technique,spatiotemporal database
AI 理解论文
溯源树
样例
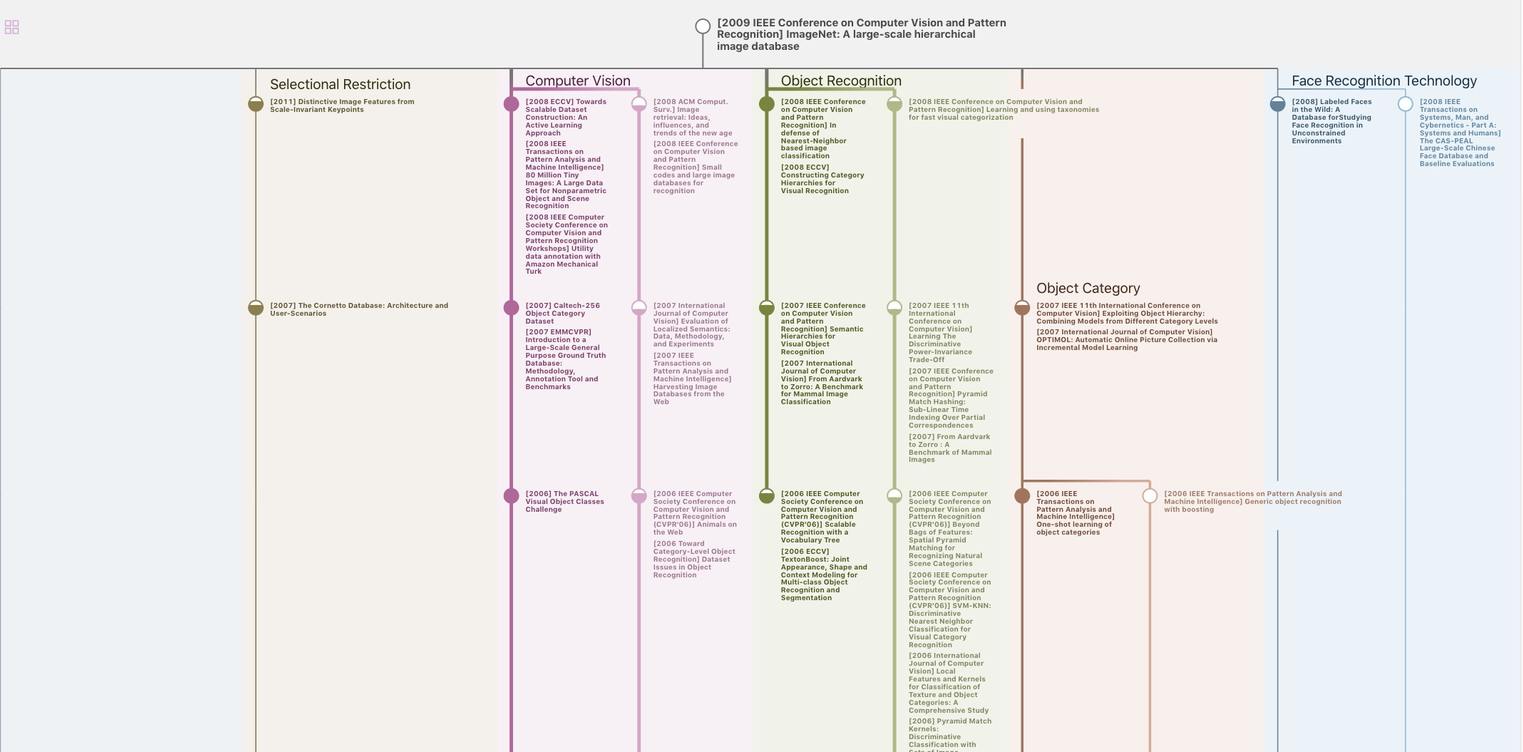
生成溯源树,研究论文发展脉络
Chat Paper
正在生成论文摘要