Incremental focal loss GANs
Information Processing & Management(2020)
摘要
Generative Adversarial Networks (GANs) have achieved inspiring performance in both unsupervised image generation and conditional cross-modal image translation. However, how to generate quality images at an affordable cost is still challenging. We argue that it is the vast number of easy examples that disturb training of GANs, and propose to address this problem by down-weighting losses assigned to easy examples. Our novel Incremental Focal Loss (IFL) progressively focuses training on hard examples and prevents easy examples from overwhelming the generator and discriminator during training. In addition, we propose an enhanced self-attention (ESA) mechanism to boost the representational capacity of the generator. We apply IFL and ESA to a number of unsupervised and conditional GANs, and conduct experiments on various tasks, including face photo-sketch synthesis, map↔aerial-photo translation, single image super-resolution reconstruction, and image generation on CelebA, LSUN, and CIFAR-10. Results show that IFL boosts learning of GANs over existing loss functions. Besides, both IFL and ESA make GANs produce quality images with realistic details in all these tasks, even when no task adaptation is involved.
更多查看译文
关键词
Generative adversarial networks,Image generation,Image-to-image translation,Face photo-sketch synthesis,Image super-resolution reconstruction
AI 理解论文
溯源树
样例
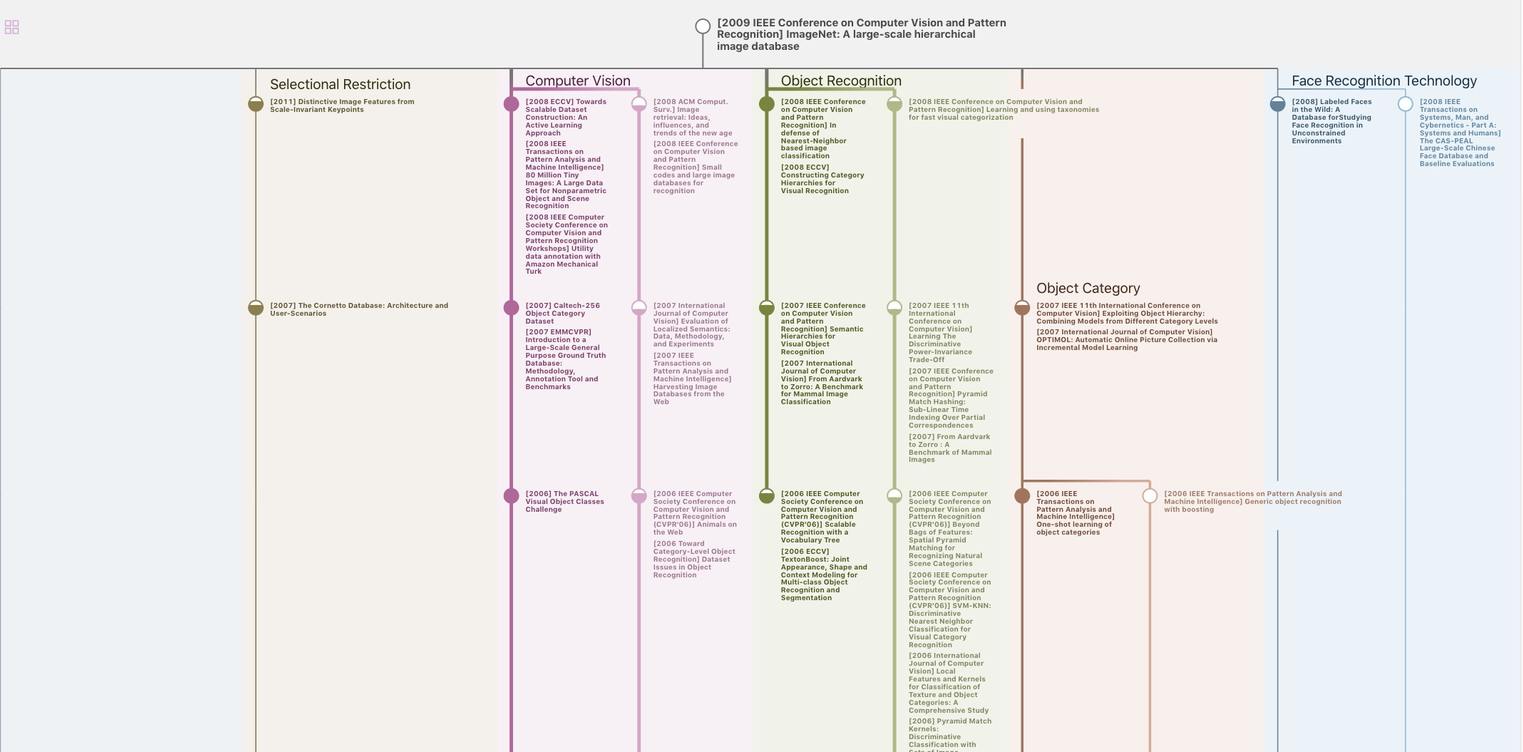
生成溯源树,研究论文发展脉络
Chat Paper
正在生成论文摘要