Things2Vec: Semantic Modeling in the Internet of Things with Graph Representation Learning
IEEE Internet of Things Journal(2020)
摘要
The advent of fifth generation (5G) enables the Internet of Things (IoT) to connect a massive number of things. The interaction and communication among these things generate an enormous amount of context-aware data that is semantically diverse. Traditional data representation approaches, such as semantic annotation, ontology, and semantic Web technology are rule based, which lack flexibility and adaptability when applied to IoT. To address the challenge, this article mainly focuses on the problem of semantic representation, which is essential for processing and fusion of IoT data. To serve as a bridge, we propose a high-level framework, namely, Things2Vec, which aims to produce the latent semantic representations from the interaction of things through the graph embedding technique. These semantic representations benefit various IoT semantic analysis tasks, such as the IoT service recommendation and automation of things. In Things2Vec, we utilize the graph to model the function sequence relationships that are generated by the interaction of things, which is called the IoT context graph. Since these function sequence relationships are heterogeneous in terms of semantics, it causes general graph embeddings to fail to learn complete information. Thus, we propose a biased random walk procedure, which is tailored to capture the neighborhoods of nodes with different types of semantic relationships. Extensive experiments are carried out, and our results show that the proposed method can effectively capture the semantic relationships among context-aware data in IoT.
更多查看译文
关键词
Graph embedding,Internet of Things (IoT),semantics
AI 理解论文
溯源树
样例
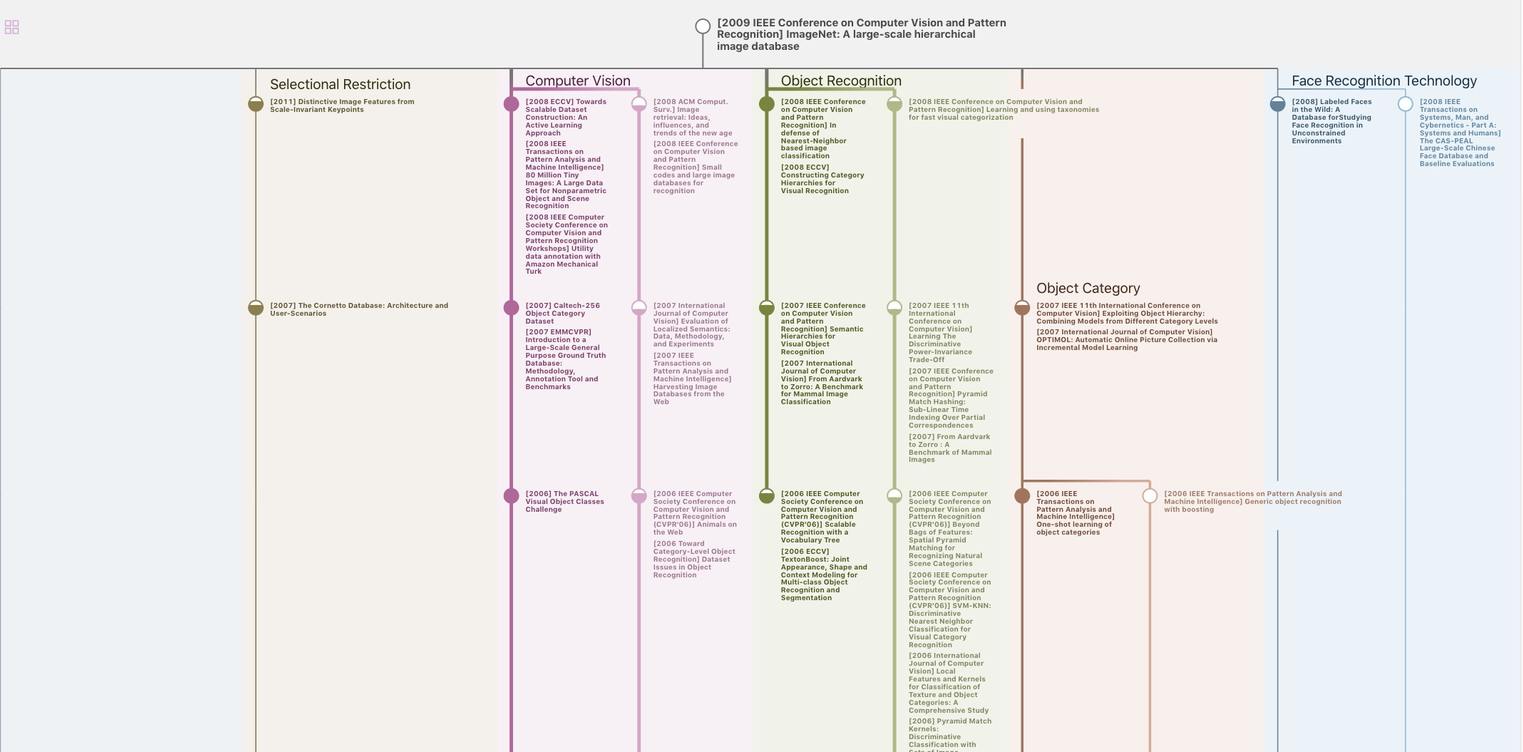
生成溯源树,研究论文发展脉络
Chat Paper
正在生成论文摘要